Heart Disease Prediction with 100% Accuracy, Using Machine Learning: Performance Improvement with Features Selection and Sampling.
2023 8th IEEE International Conference on Network Intelligence and Digital Content (IC-NIDC)(2023)
摘要
Heart diseases are commonly diagnosed by using the technique of; angiography which is time consuming procedure. Therefore, researchers have been encouraged to find out the alternatives like; machine learning (ML). In this research work, we present a method to predict the heart disease using correlation based on feature subset selection and sampling. Different ML algorithms have been used in this research to model and cross validate. The dataset consists of 14 features and 1000 records of heart disease patients. Two sampling techniques; Oversampling and Synthetic Minority Optimization Technique (SMOTE) have been used. In case of oversampling, the Random Forest (RF) algorithm achieved highest prediction accuracy i.e., 99.20%. Proposed model with SMOTE improved the prediction accuracy of all algorithms to 100% except k-nn, which is also improved 18.16%. In this study the features selection has been made on the basis of correlation, in future we plan to tap in other feature selection methodologies. Furthermore in this study cross validation have been used to address the issue of over-fitting, this work can be extended by using other techniques like; Federated Learning to address the subjected problem.
更多查看译文
关键词
Machine Learning,Over-Sampling,Cardiovascular diseases,Features Selection,Cross validation,Correlation
AI 理解论文
溯源树
样例
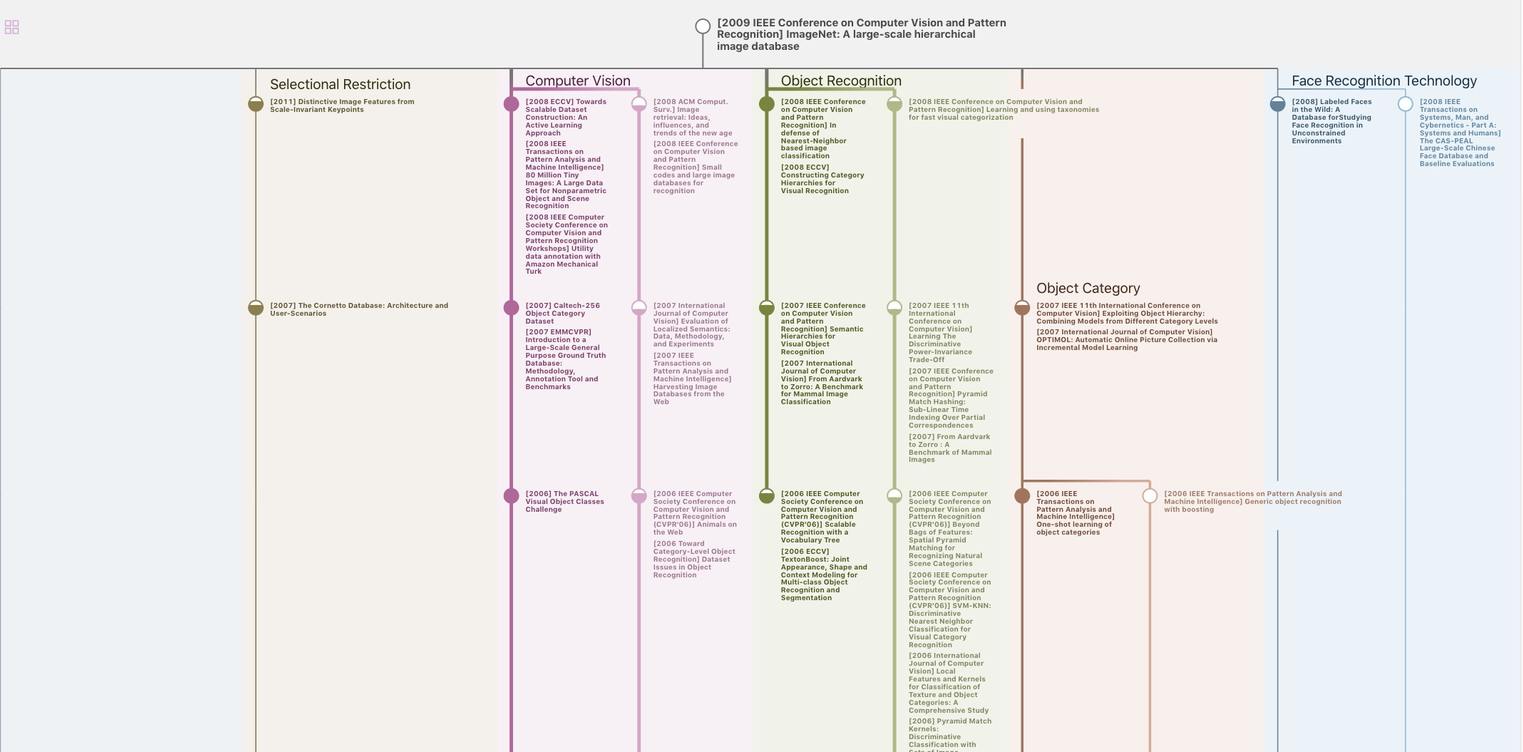
生成溯源树,研究论文发展脉络
Chat Paper
正在生成论文摘要