Retrieve, Generate and Rerank: Simple and Effective Framework for Guided Human-Like Questions Generation.
2023 8th IEEE International Conference on Network Intelligence and Digital Content (IC-NIDC)(2023)
Abstract
Most existing methods for question generation create questions purely depend on the source text. The problem is that these methods tend to directly copy the expressions from given passages and lack the sufficient capability to ask diverse and human-like questions. To alleviate this problem, we present a simple and effective Retrieve-Generate-Rerank (RGR) framework that guides the generation with references in training set. Specifically, for every inputted passage and answer, a variety of references are retrieved, with irrelevant information filtered to produce clues. Each clue guides the generation of a question with different patterns and expressions. These questions are then re-ranked to find the most human-like outcome. Experimental results show that our approach consistently improve the performance of existing models on two datasets, and achieves state-of-the-art result on NewsQA. In addition, further investigation and human evaluation demonstrate that our method can generate more diverse and consistent questions.
MoreTranslated text
Key words
Question Generation,Retrieval-augmented Text Generation,Diversity
AI Read Science
Must-Reading Tree
Example
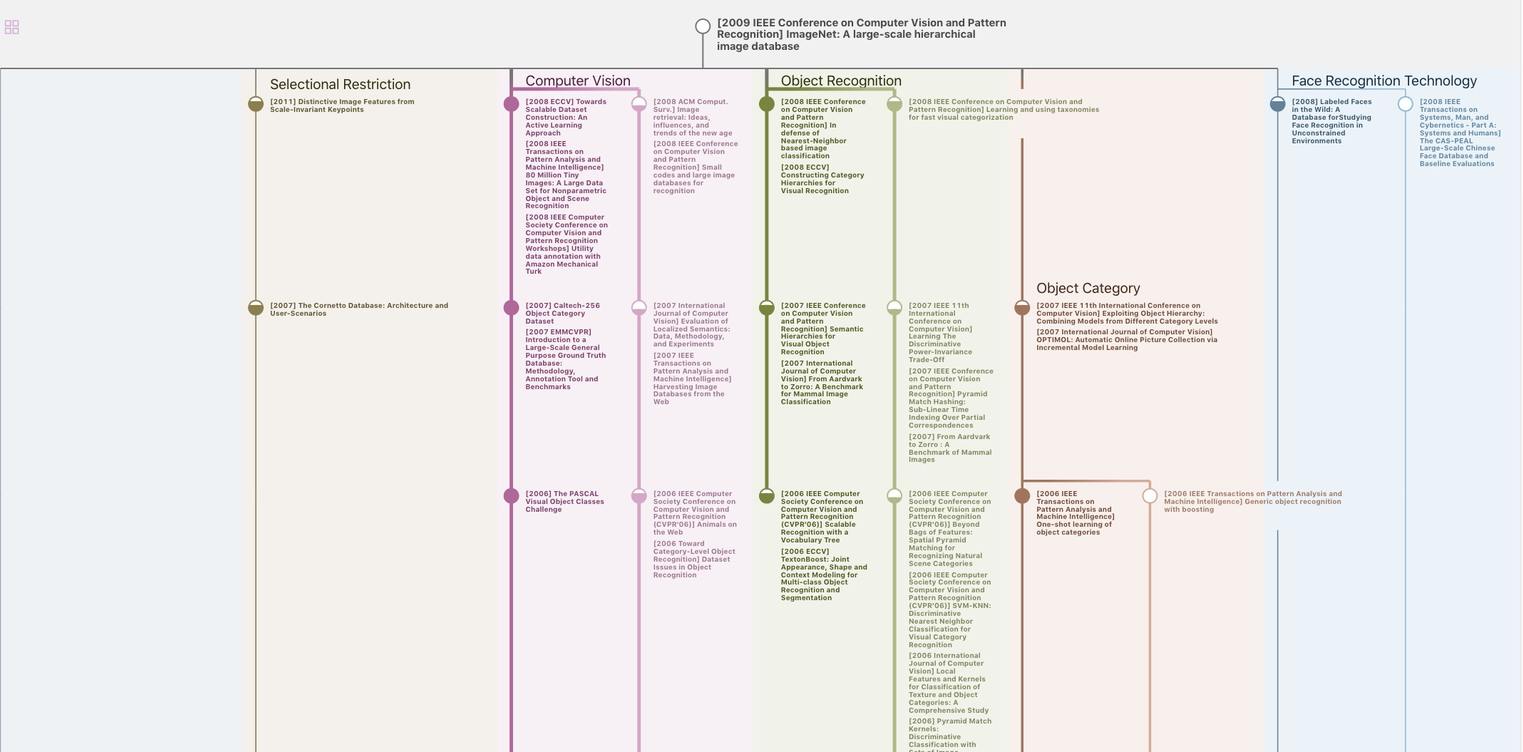
Generate MRT to find the research sequence of this paper
Chat Paper
Summary is being generated by the instructions you defined