FedPAGE: Pruning Adaptively Toward Global Efficiency of Heterogeneous Federated Learning
IEEE-ACM TRANSACTIONS ON NETWORKING(2023)
摘要
When workers are heterogeneous in computing and transmission capabilities, the global efficiency of federated learning suffers from the straggler issue, i.e., the slowest worker drags down the overall training process. We propose a novel and efficient federated learning framework named FedPAGE, where workers perform distributed pruning adaptively towards global efficiency, i.e., fast training and high accuracy. For fast training, we develop a pruning rate learning approach generating an adaptive pruning rate for each worker, making the overall update time approximate to the fastest worker's update time, i.e., no stragglers. For high accuracy, we find that structural similarity between sub-models is essential to global model accuracy in the distributed pruning, and thus propose the CIG_X pruning scheme to ensure maximum similarity. Meanwhile, we adopt the sparse training and design model aggregating of different size sub-models to cope with distributed pruning. We prove the convergence of FedPAGE and demonstrate the effectiveness of FedPAGE on image classification and natural language inference tasks. Compared with the state-of-the-art, FedPAGE achieves higher accuracy with the same speedup ratio.
更多查看译文
关键词
Federated learning,straggler issue,global efficiency,distributed pruning
AI 理解论文
溯源树
样例
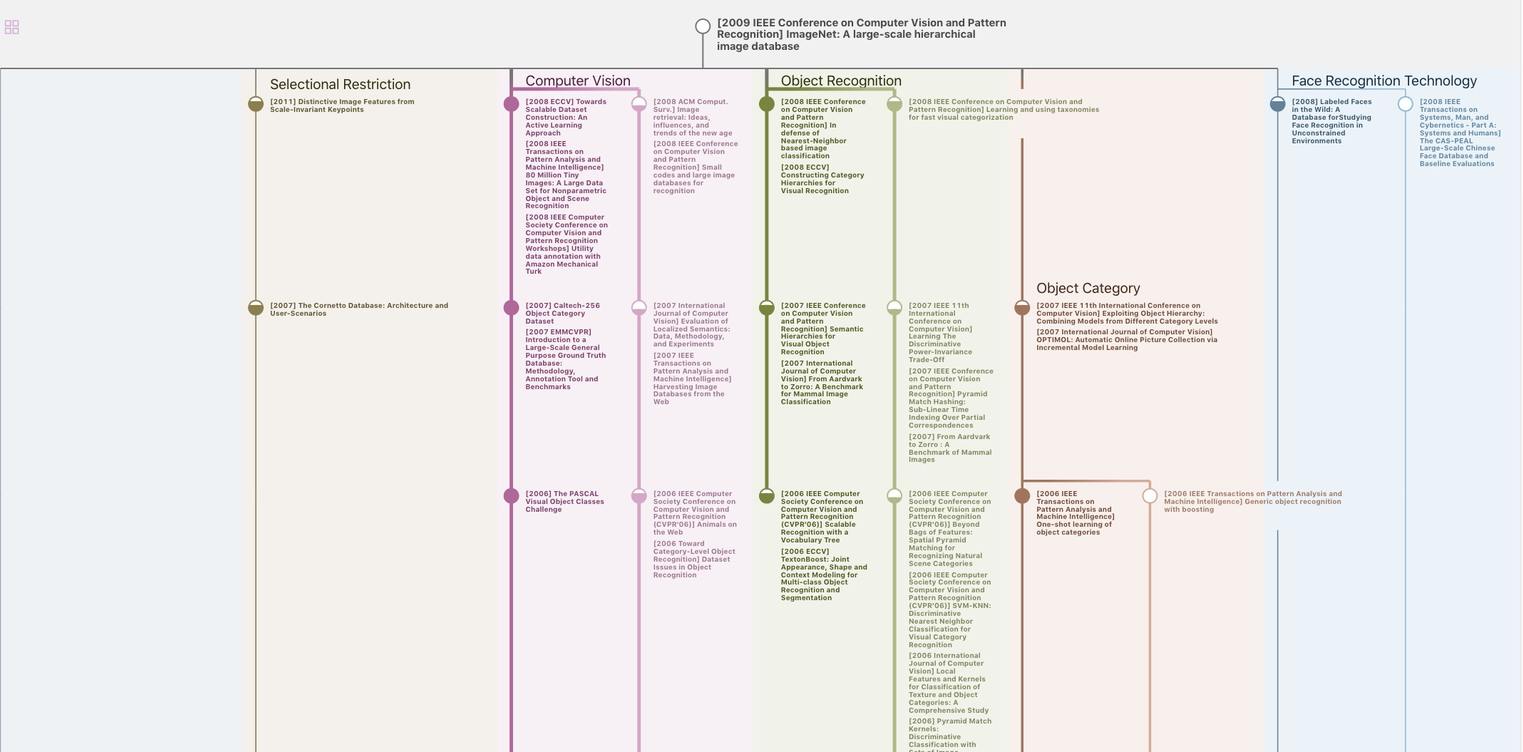
生成溯源树,研究论文发展脉络
Chat Paper
正在生成论文摘要