CenterCoop: Center-Based Feature Aggregation for Communication-Efficient Vehicle-Infrastructure Cooperative 3D Object Detection.
IEEE Robotics Autom. Lett.(2024)
摘要
Vehicle-Infrastructure Cooperative (VIC) 3D object detection is a challenging task for balancing communication bandwidth and detection performance. Intermediate fusion is recently studied to reach a better balance by transferring feature maps. Existing works mainly perform spatial-wise fusion and adopt feature compression to alleviate bandwidth cost by high-resolution feature maps, which would inevitably lead to information loss. Besides, overlapping observations between the two sensors would lead to near-duplicate detections, making trivial improvement to cooperative task while causing unnecessary bandwidth cost. To mitigate these problems, we propose a novel feature aggregation framework called CenterCoop, which first encodes the informative cues from the whole Bird's Eye View (BEV) context into compact center representations, enabling feature aggregation at sequence-level to significantly reduce the communication cost. Furthermore, to tackle the redundancy of transmitted data, we incorporate communication-aware regularization which enforces the network to extract complementary and beneficial cues for collaboration task. From an information-theoretic perspective, the proposed auxiliary constraints facilitate cooperative-view independence mining, resulting in enlarged perception range within the limited bandwidth. Extensive experiments on the DAIR-V2X dataset demonstrate the superior performance-bandwidth trade-off of CenterCoop, which achieves the state-of-the-art detection performance with less than 10% bandwidth cost.
更多查看译文
关键词
Computer vision for transportation,sensor fusion,object detection,segmentation and categorization
AI 理解论文
溯源树
样例
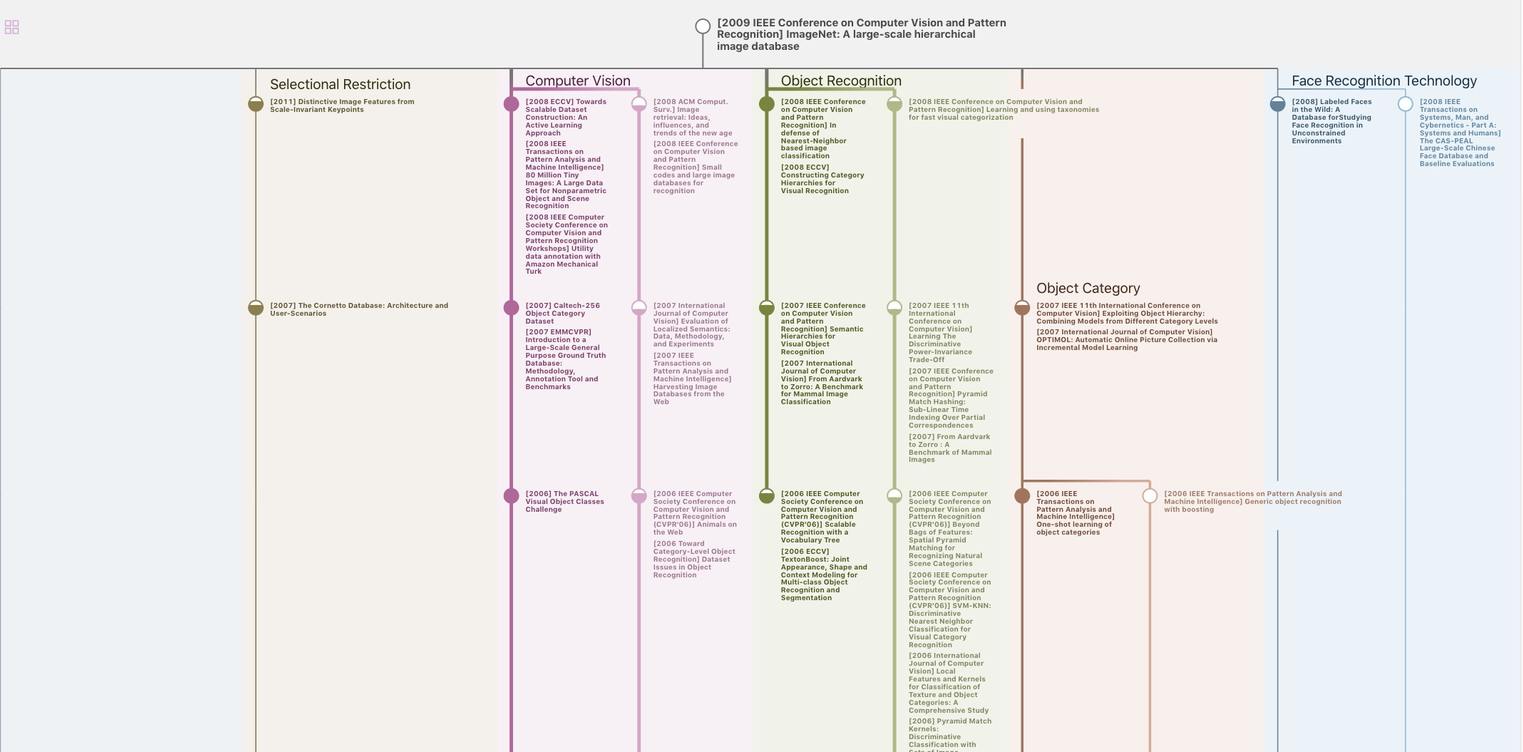
生成溯源树,研究论文发展脉络
Chat Paper
正在生成论文摘要