Parallelization of Recurrent Neural Network-Based Equalizer for Coherent Optical Systems via Knowledge Distillation
JOURNAL OF LIGHTWAVE TECHNOLOGY(2024)
摘要
The recurrent neural network (RNN)-based equalizers, especially the bidirectional long-short-term memory (biLSTM) structure, have already been proven to outperform the feed-forward NNs in nonlinear mitigation in coherent optical systems. However, the recurrent connections still prevent the computation from being fully parallelizable. To circumvent the non-parallelizability of recurrent-based equalizers, we propose, for the first time, knowledge distillation (KD) to recast the biLSTM into a parallelizable feed-forward 1D-convolutional NN structure. In this work, we applied KD to the cross-architecture regression problem, which is still in its infancy. We highlight how the KD helps the student's learning from the teacher in the regression problem. Additionally, we provide a comparative study of the performance of the NN-based equalizers for both the teacher and the students with different NN architectures. The performance comparison was carried out in terms of the Q-factor, inference speed, and computational complexity. The equalization performance was evaluated using both simulated and experimental data. The 1D-CNN outperformed other NN types as a student model with respect to the Q-factor. The proposed 1D-CNN showed a significant reduction in the inference time compared to the biLSTM while maintaining comparable performance in the experimental data and experiencing only a slight degradation in the Q-factor in the simulated data.
更多查看译文
关键词
Artificial intelligence,machine learning,recurrent neural networks,parallelization,knowledge distillation,nonlinear equalizer,coherent detection
AI 理解论文
溯源树
样例
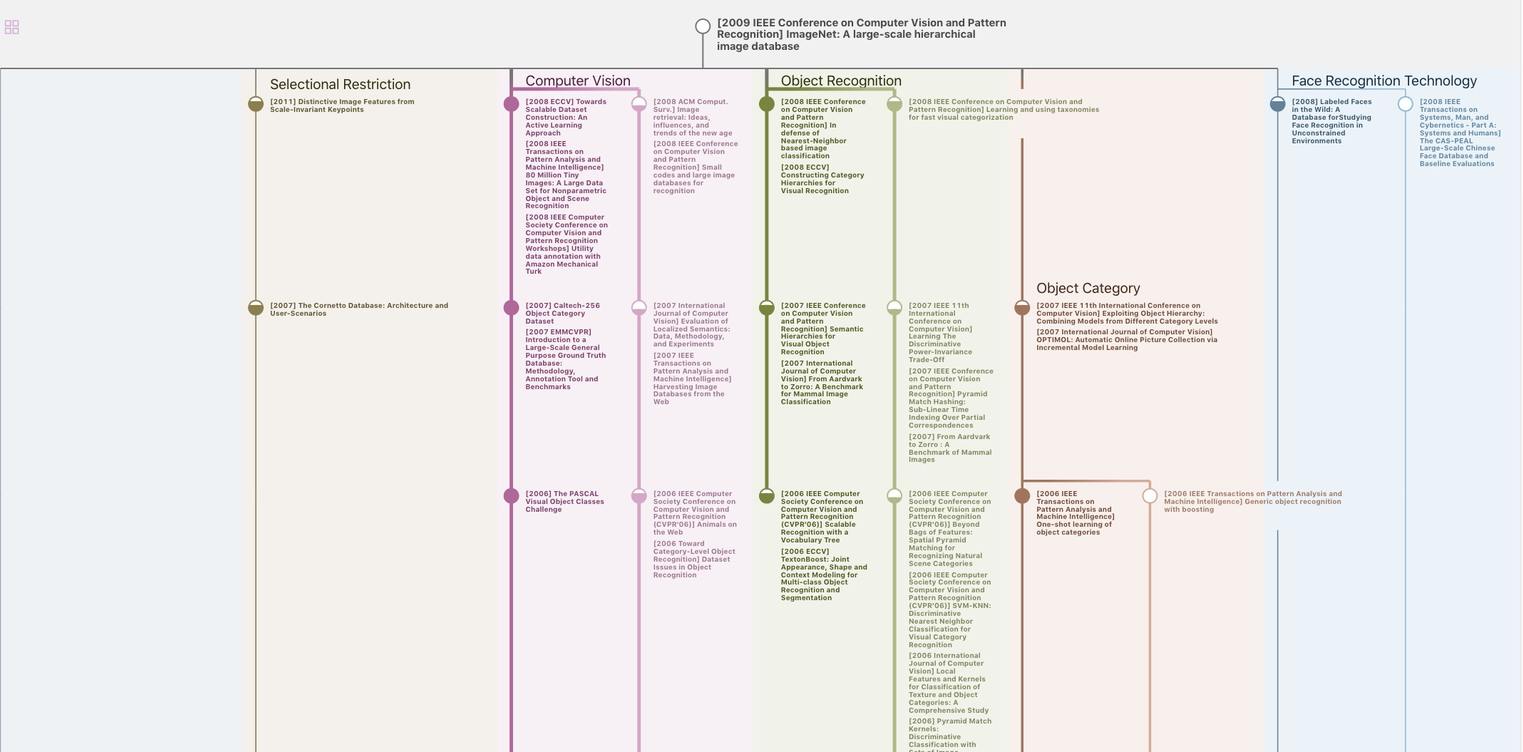
生成溯源树,研究论文发展脉络
Chat Paper
正在生成论文摘要