A Transformation-Aggregation Framework for State Representation of Autonomous Driving Systems
IEEE TRANSACTIONS ON INTELLIGENT TRANSPORTATION SYSTEMS(2024)
摘要
The effective design of state representation plays a pivotal role in bridging the gap between perception and downstream decision-making and control in autonomous driving systems based on reinforcement learning. Among perception observations, accurately representing the set of surrounding participants poses significant challenges due to its variable size and unordered nature. These challenges result in dimension sensitivity and permutation sensitivity issues, respectively. To systematically tackle these complexities, we introduce a general framework for learning a state representation module, which can be regarded as a combination of any transformation and aggregation modules. Specifically, we employ a transformer encoder as the transformation module to attract features individually and design a novel aggregation module called Feature-wise Sorted Query (FSQ) to effectively aggregate the feature set into a state representation vector while adaptively attending to essential participant features. In FSQ, the feature-wise sort and latent query attention mechanisms can address permutation sensitivity and dimension sensitivity, respectively. Additionally, we propose an offline training paradigm to decouple state representation learning from downstream reinforcement learning tasks, enhancing stability and avoiding overfitting for particular scenarios. Simulation and experimental results demonstrate the superior performance and a more stable training process of our method across six varying-size set regression tasks and downstream autonomous driving tasks, particularly when leveraging the offline training paradigm.
更多查看译文
关键词
Autonomous driving,reinforcement learning,state representation
AI 理解论文
溯源树
样例
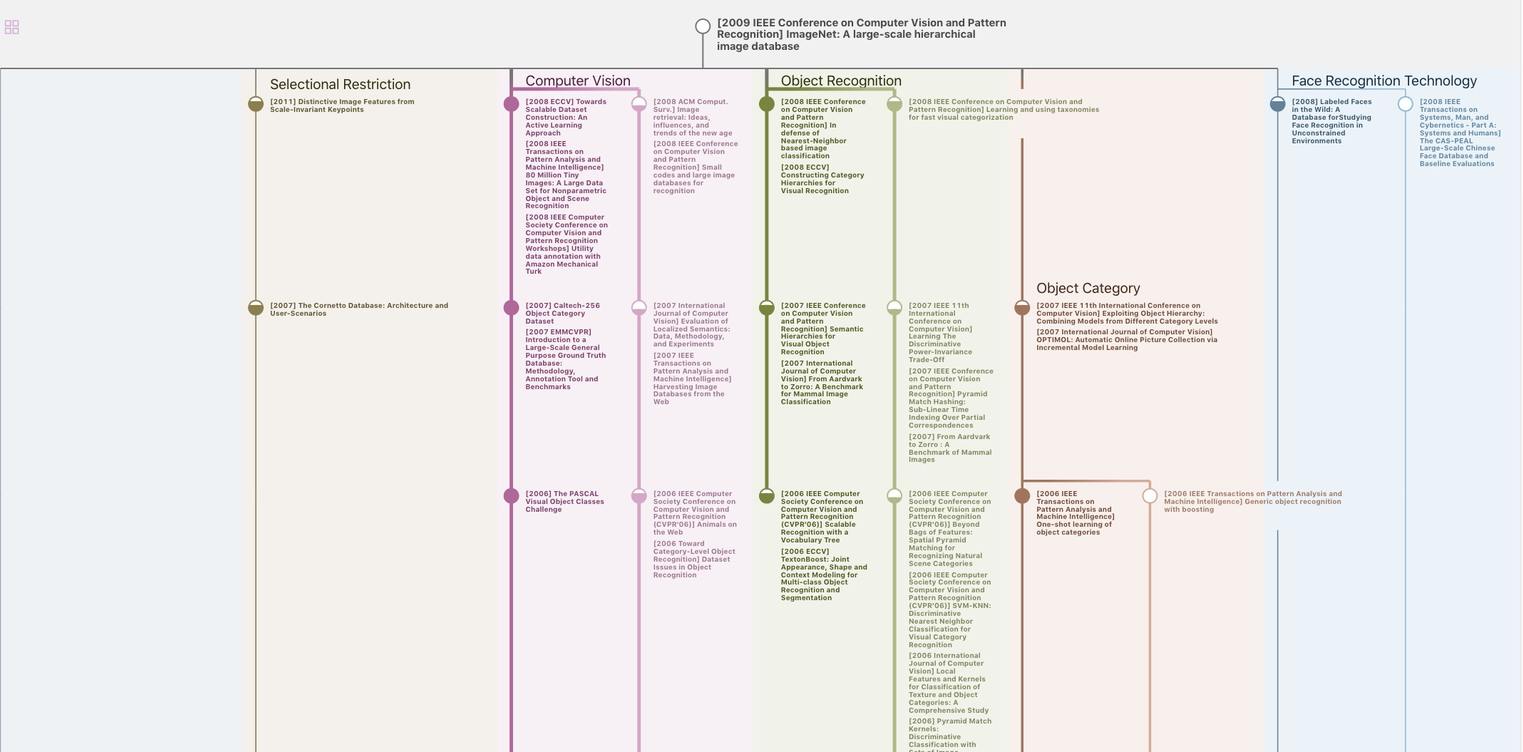
生成溯源树,研究论文发展脉络
Chat Paper
正在生成论文摘要