MetaSTNet: Multimodal Meta-Learning for Cellular Traffic Conformal Prediction.
IEEE Trans. Netw. Sci. Eng.(2024)
摘要
Network traffic prediction techniques have attracted much attention since they are valuable for network congestion control and user experience improvement. While existing prediction techniques can achieve favorable performance when there is sufficient training data, it remains a great challenge to make accurate predictions when only a small amount of training data is available. To tackle this problem, we propose a deep learning model, entitled MetaSTNet, based on a multimodal meta-learning framework. It is an end-to-end network architecture that trains the model in a simulator and transfers the meta-knowledge to a real-world environment, which can quickly adapt and obtain accurate predictions on a new task with only a small amount of real-world training data. In addition, we further employ cross conformal prediction to assess the calibrated prediction intervals. Extensive experiments have been conducted on realworld datasets to illustrate the efficiency and effectiveness of MetaSTNet.
更多查看译文
关键词
Cellular traffic prediction,meta-learning,spatiotemporal dependencies,multimodal data,prediction intervals
AI 理解论文
溯源树
样例
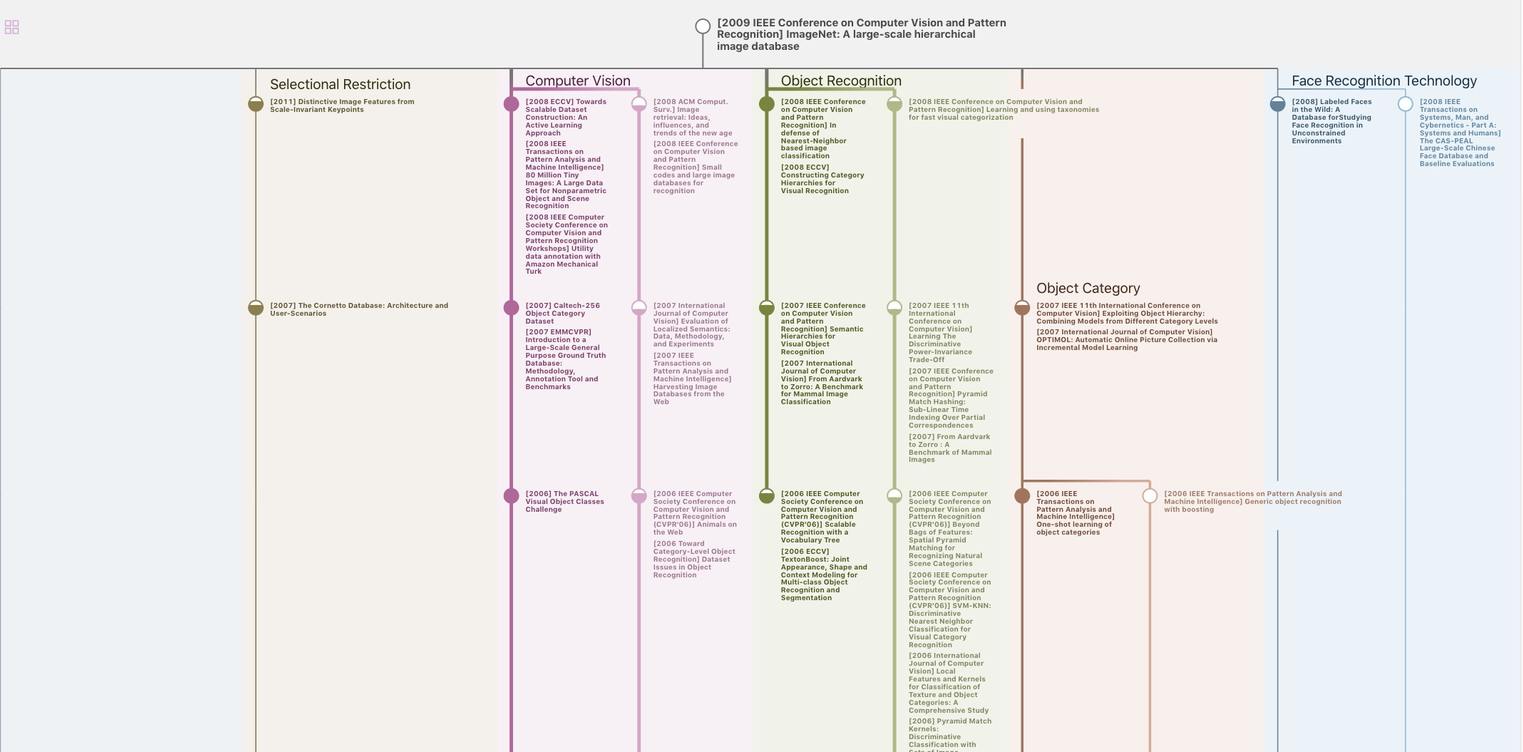
生成溯源树,研究论文发展脉络
Chat Paper
正在生成论文摘要