Hybrid Network Model Based on Data Enhancement for Short-Term Power Prediction of New PV Plants
JOURNAL OF MODERN POWER SYSTEMS AND CLEAN ENERGY(2024)
摘要
This study proposes a hybrid network model based on data enhancement to address the problem of low accuracy in photovoltaic (PV) power prediction that arises due to insufficient data samples for new PV plants. First, a time-series generative adversarial network (TimeGAN) is used to learn the distribution law of the original PV data samples and the temporal correlations between their features, and these are then used to generate new samples to enhance the training set. Subsequently, a hybrid network model that fuses bi-directional long-short term memory (BiLSTM) network with attention mechanism (AM) in the framework of deep & cross network (DCN) is constructed to effectively extract deep information from the original features while enhancing the impact of important information on the prediction results. Finally, the hyperparameters in the hybrid network model are optimized using the whale optimization algorithm (WOA), which prevents the network model from falling into a local optimum and gives the best prediction results. The simulation results show that after data enhancement by TimeGAN, the hybrid prediction model proposed in this paper can effectively improve the accuracy of short-term PV power prediction and has wide applicability.
更多查看译文
关键词
Predictive models,Data models,Correlation,Training,Optimization,Hybrid power systems,Meteorology,New photovoltaic (PV) plant,short-term prediction,time-series generative adversarial network (TimeGAN),hybrid network,hyperparameter
AI 理解论文
溯源树
样例
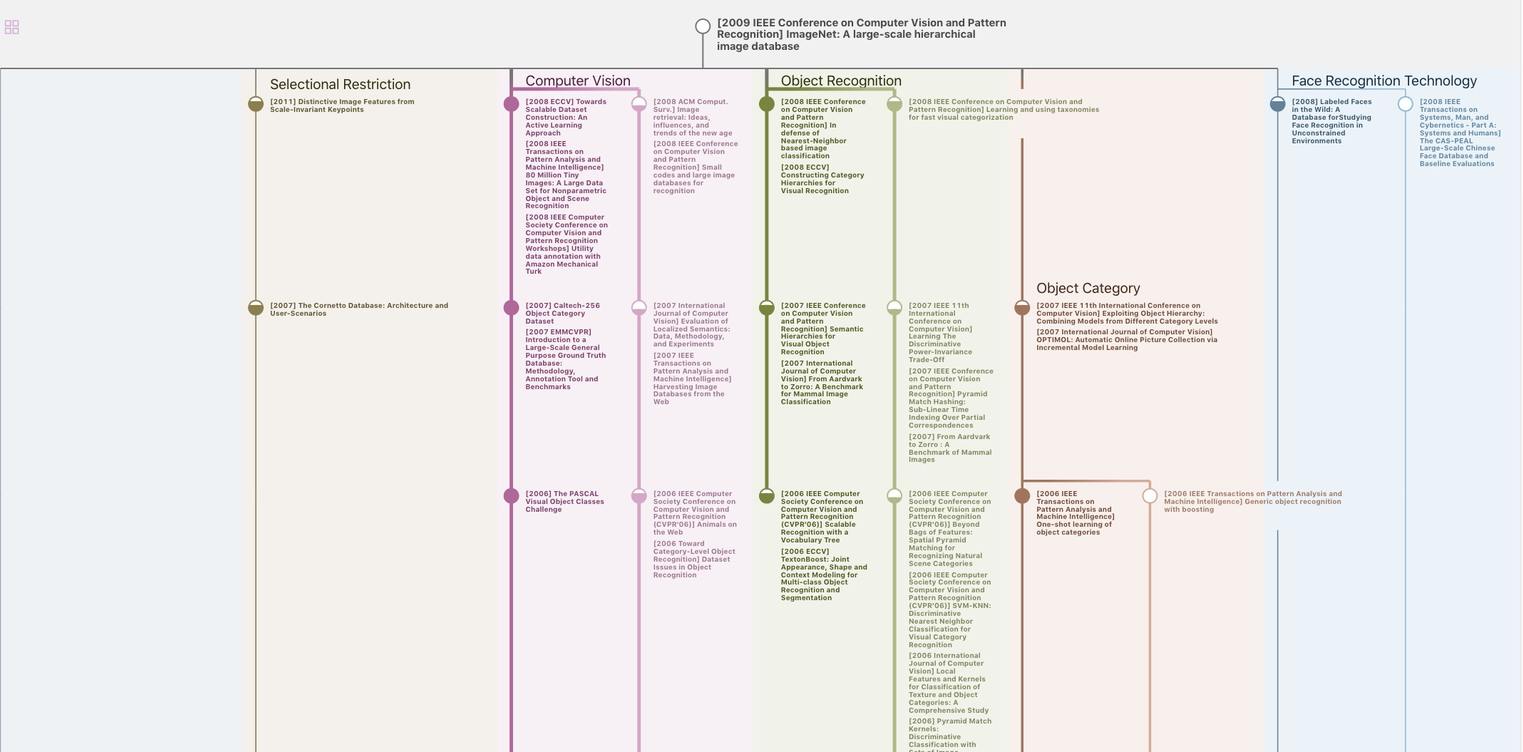
生成溯源树,研究论文发展脉络
Chat Paper
正在生成论文摘要