Multidimensional Scaling Algorithm for Mobile Swarming UAVs Localization
IEEE Transactions on Intelligent Vehicles(2023)
Abstract
In recent years, unmanned aerial vehicles (UAVs), especially swarming UAVs, have been widely used in various Internet of Things (IoT) scenarios. Multi-Dimensional Scaling (MDS) localization is sensitive to noise and requires communication between each UAV, which is not feasible in reality. Moreover, the MDS method cannot be applied to dynamic UAV clusters due to the high computational complexity, which introduces a large amount of communication overhead. To address the above problems, this paper proposes a fast-clustering localization method named Dynamic Cluster-MDS (DC-MDS), which is able to cluster UAV swarms according to the energy of UAVs in a short period of time, localize among clusters, and merge clusters and clusters upon completion of localization. This algorithm can greatly improve the accuracy of localization and the robustness of the algorithm. In addition, the DC-MDS method can reduce the computational complexity and prevent the optimization from falling into the local optimum to maintain the stability of the algorithm by introducing the learning rate. The scaling by majorizing a complicated function (SMACOF) algorithm based on MDS optimization is sensitive to initialization, and the initialization of DC-MDS uses the core map fusion algorithm, which can improve the accuracy of node initialization and reduce the number of subsequent calculations. Finally, simulation results show that the algorithm proposed in this paper has significantly improved the accuracy and algorithm robustness for UAV cluster localization and can be used for dynamic UAV swarm localization.
MoreTranslated text
Key words
MDS,localization,SMACOF,UAV swarms
AI Read Science
Must-Reading Tree
Example
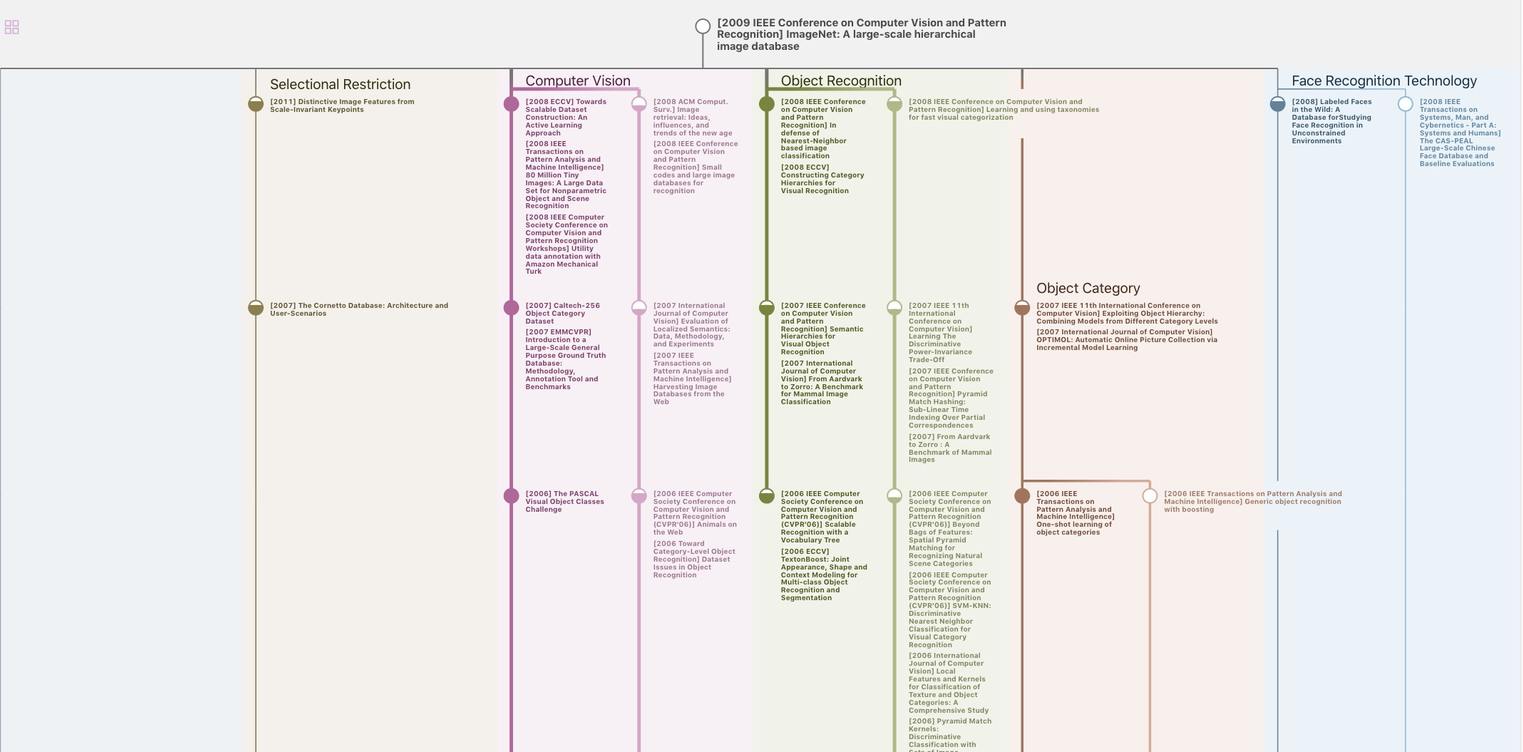
Generate MRT to find the research sequence of this paper
Chat Paper
Summary is being generated by the instructions you defined