Ego Vehicle Trajectory Prediction Based on Time-Feature Encoding and Physics-Intention Decoding
IEEE TRANSACTIONS ON INTELLIGENT TRANSPORTATION SYSTEMS(2024)
Abstract
In the stage of man-machine cooperative driving, accurately predicting the trajectory of the ego vehicle can help intelligent system understand future risk and adjust the control authority of the man-machine, thereby improving the performance of the man-machine system and eliminating man-machine conflicts. However, existing high-performance trajectory prediction methods are more focused on fully autonomous vehicles, and it is difficult to deal with the problem of driving trajectory prediction with different risks when the driver is in the loop. So, an ego vehicle trajectory prediction method based on time-feature encoding and physics-intention decoding (TFE-PID) is proposed. Through the bidirectional enhancement of the encoding and decoding process, it can accurately predict the trajectory of the ego vehicle by using only the state data of the vehicle and the driver. In the encoding stage, time and feature information are used for dual encoding, which makes the amount of information carried in the context vector used for decoding more abundant. In the decoding stage, context vector, physical prediction data, and driver's intention are used to control the flow of information in the network, which enables the model to converge in a direction that is more consistent with the physical characteristics of the vehicle and driver's intention. The experimental results show that TFE-PID can accurately predict the trajectory of the ego vehicle under different risky driving behaviors of drivers, and has good prediction stability and generalization ability.
MoreTranslated text
Key words
Trajectory,Encoding,Decoding,Hafnium,Vehicles,Human-machine systems,Feature extraction,Trajectory prediction,man-machine cooperative driving,deep learning,encoding and decoding
AI Read Science
Must-Reading Tree
Example
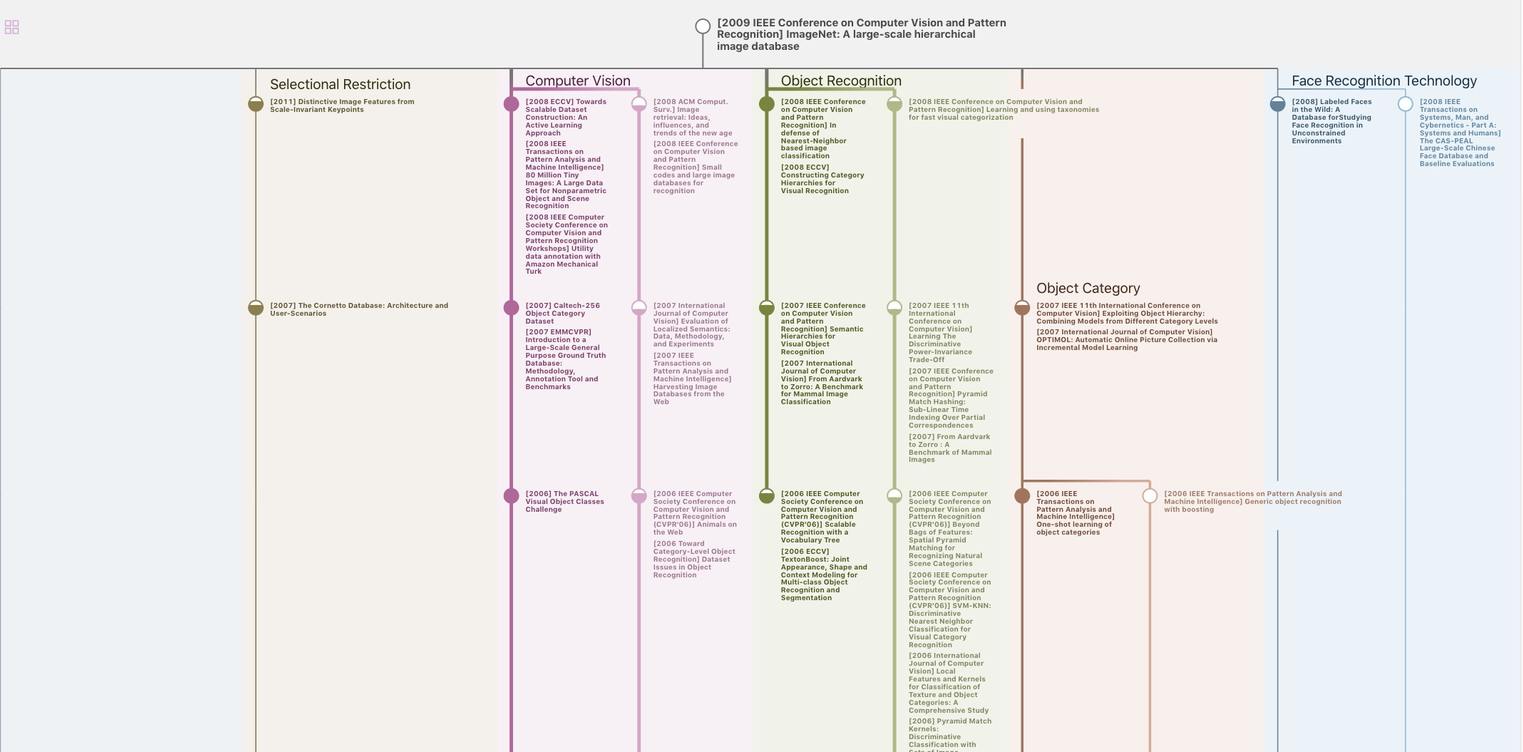
Generate MRT to find the research sequence of this paper
Chat Paper
Summary is being generated by the instructions you defined