Deep Reinforcement Learning Enabled Multi-UAV Scheduling for Disaster Data Collection With Time-Varying Value
IEEE TRANSACTIONS ON INTELLIGENT TRANSPORTATION SYSTEMS(2024)
摘要
The congestion and disruption of information infrastructures frequently happen during disasters, which would hinder the understanding of disaster scenarios, and thus impede rapid response activities. With the advantages of high flexibility and efficiency, this paper proposes to use UAVs as temporary and mobile relays for disaster data collection. However, different from many existing data collection scenarios in industrial sectors, the disaster data value varies with UAV arrival time and service time in terms of their importance for disaster response, which makes the scheduling of UAVs challenging. To address such a problem, this paper proposes an attention-based Deep Reinforcement Learning (DRL) method for multi-UAV scheduling considering time-varying data value. Specifically, the problem is modeled as a specific team orienteering problem with time-varying value. Then the relationships between UAV route selection and service time at each node are analyzed, based on which the computing efficiency for solution algorithms can be improved. After that, an attention-based DRL method is developed, with a calibrated attention model and decoding method. Finally, systematic computational experiments are conducted to evaluate the performance of the proposed method, which demonstrates its superiority over popular methods in UAV scheduling, especially for large-scale and complex scenarios.
更多查看译文
关键词
Disaster response,unmanned aerial vehicle,multi-UAV scheduling,data collection,deep reinforcement learning,time-varying value
AI 理解论文
溯源树
样例
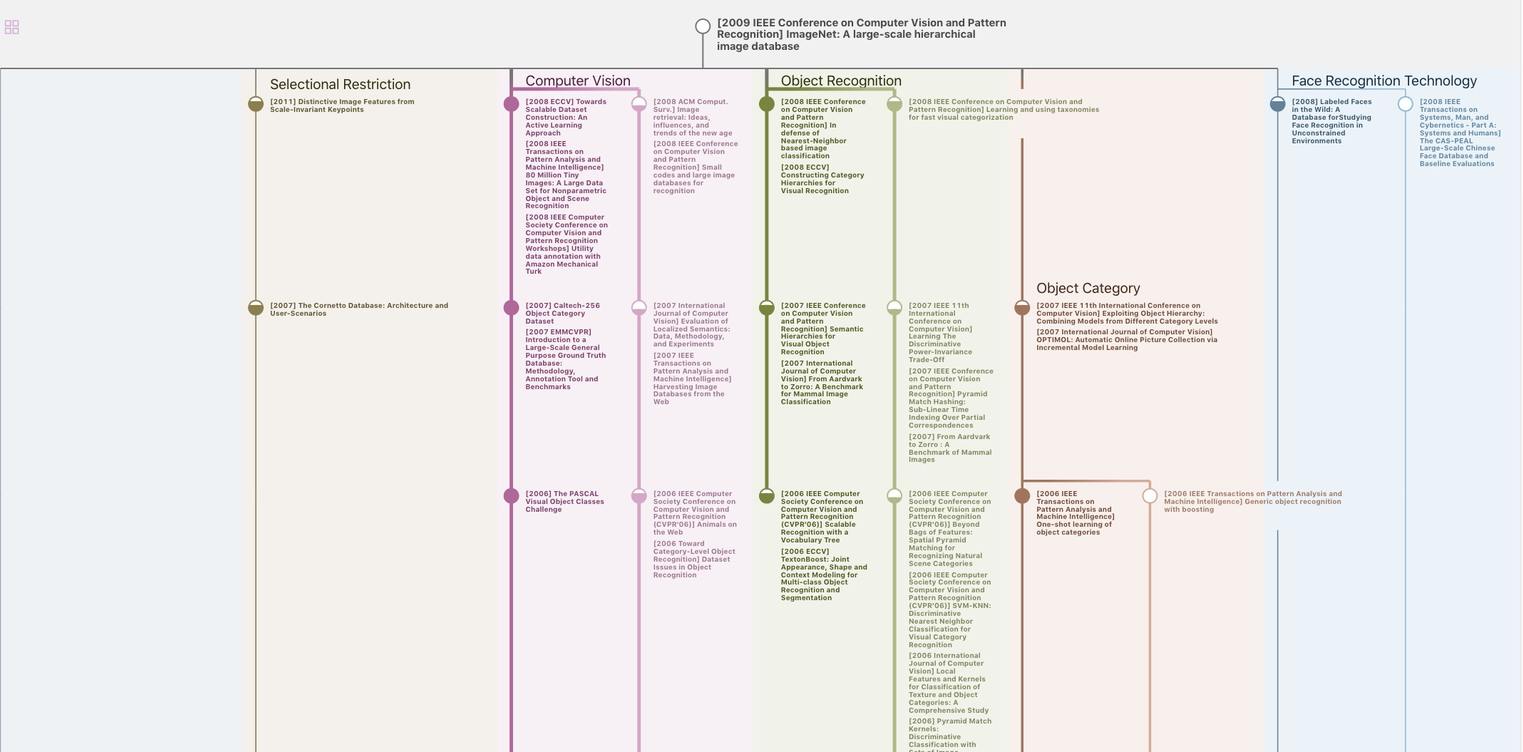
生成溯源树,研究论文发展脉络
Chat Paper
正在生成论文摘要