A Deep Learning Framework to Explore Influences of Data Noises on Lane-Changing Intention Prediction
IEEE TRANSACTIONS ON INTELLIGENT TRANSPORTATION SYSTEMS(2024)
关键词
Predictive models,Hidden Markov models,Trajectory,Deep learning,Data models,Kinematics,Feature extraction,Lane-changing,data noises,attention mechanism,deep learning,driving intention
AI 理解论文
溯源树
样例
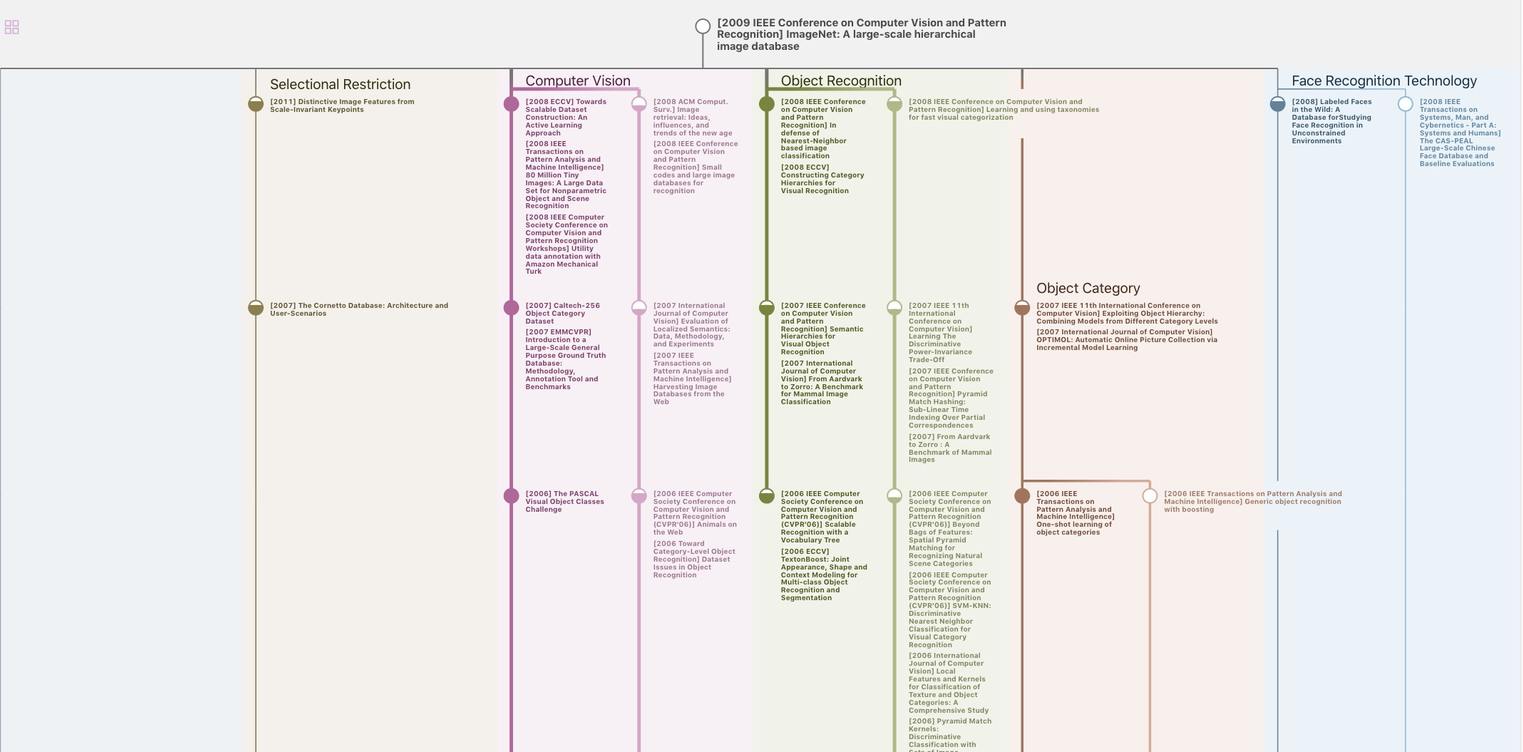
生成溯源树,研究论文发展脉络
Chat Paper
正在生成论文摘要