Discrete-Time Nonlinear Optimal Control Using Multi-Step Reinforcement Learning
IEEE TRANSACTIONS ON CIRCUITS AND SYSTEMS II-EXPRESS BRIEFS(2024)
Abstract
This brief solves the optimal control problem of discrete-time nonlinear systems by proposing a multi-step reinforcement learning (RL) algorithm. The proposed multi-step RL algorithm is established based on the discrete-time optimal Bellman equation, which takes advantage of policy iteration (PI) and value iteration (VI). Benefiting from the multi-step integration mechanism, the algorithm is accelerated. The convergence of multi-step RL is proved by mathematical induction. For real-world implementation purposes, neural network (NN) and Actor-Critic architecture are introduced to approximate the iterative value functions and control policies. A numerical simulation of Chua's circuit illustrates the effectiveness of the proposed algorithm.
MoreTranslated text
Key words
Convergence,Optimal control,Heuristic algorithms,Mathematical models,Approximation algorithms,Reinforcement learning,Nonlinear systems,optimal bellman equation,actor-critic architecture,optimal control
AI Read Science
Must-Reading Tree
Example
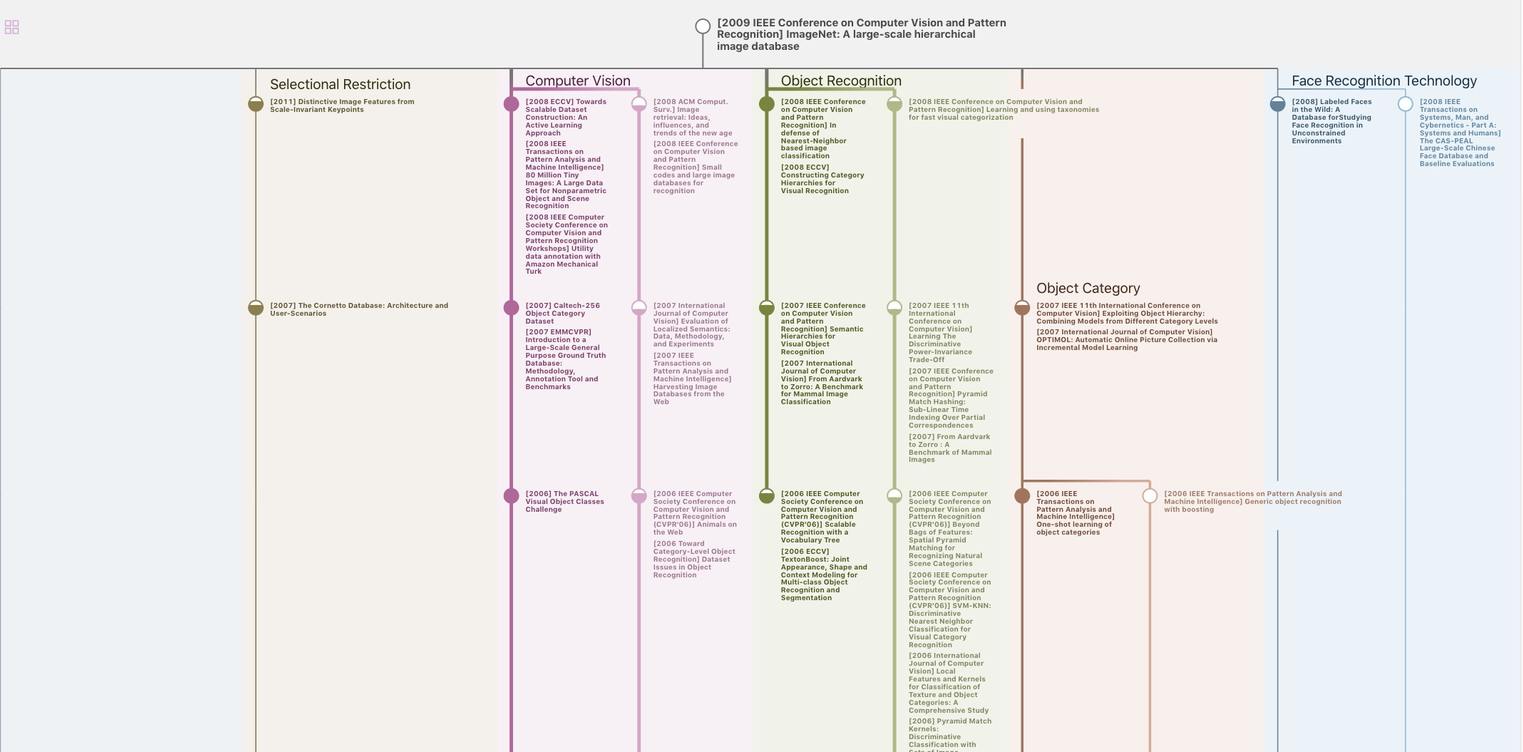
Generate MRT to find the research sequence of this paper
Chat Paper
Summary is being generated by the instructions you defined