GlobalDepth: A Global Information Aggregation Network for Depth Estimation
IEEE Transactions on Circuits and Systems II: Express Briefs(2024)
摘要
Depth estimation from a single image is a challenging yet crucial task for reconstructing 3D structures and inferring scene geometry. However, most methods struggle to effectively leverage global information at high resolutions to establish long-distance dependencies between distant pixels, thereby affecting the accurate prediction of depth for objects in shadows and faraway regions. To address this issue, we propose GlobalDepth, which utilizes the Global Information Aggregation Module (GIAM) based on self-attention mechanism to model long-distance dependencies in the spatial domain. We introduce the Global Information Enhancement Module (GIEM) based on multi-scale global average pooling to enhance global information in depth feature maps. Experimental results on the NYU-Depth-v2, KITTI, and SUNRGB-D datasets demonstrate that GlobalDepth outperforms existing methods in terms of performance and generalization capability.
更多查看译文
关键词
depth estimation,global information,self-attention,global average pooling
AI 理解论文
溯源树
样例
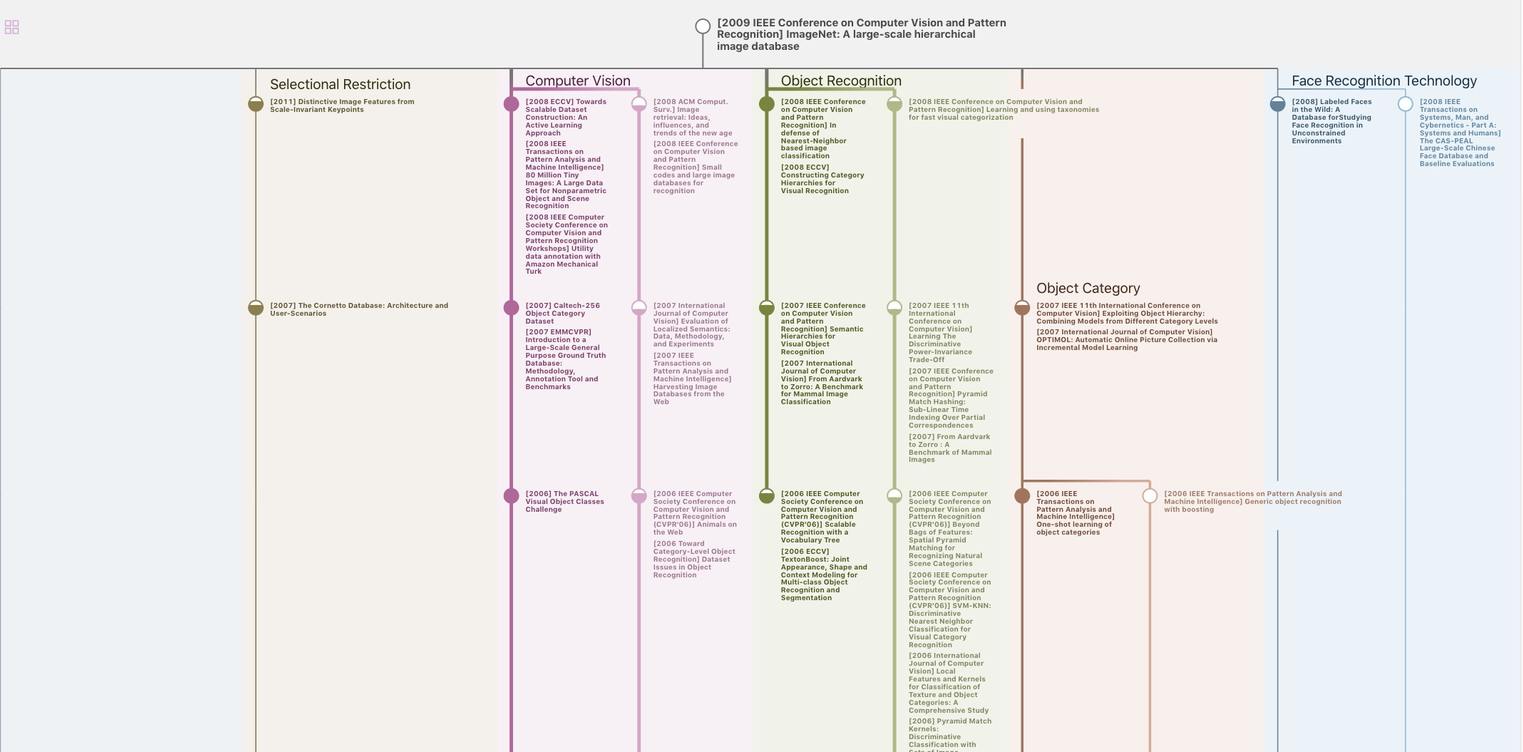
生成溯源树,研究论文发展脉络
Chat Paper
正在生成论文摘要