Privacy-Preserving Federated Learning for Power Transformer Fault Diagnosis With Unbalanced Data
IEEE TRANSACTIONS ON INDUSTRIAL INFORMATICS(2023)
摘要
This article is concerned with developing a privacy-preserving distributed-learning-based fault diagnosis approach for power transformers. Due to the constraints of data privacy, it is not possible to have enough labeled samples for training. Recently, the emergence of federated learning (FL) has provided a secure and distributed learning framework. However, the unbalanced data from multiple power stations may reduce the overall performance of FL while an untrusted central server can threaten the data privacy and security of clients. To address such challenges, a privacy-preserving FL scheme is developed for transformer fault diagnosis, where a multistep data-sharing strategy and an adaptive differential privacy technology are proposed. Specifically, amounts of shared data and noise perturbation will be designed according to the quantity of local data by the central server. The experimental results on the dataset generated according to IEC publication 60599 show that the proposed method has high diagnostic accuracy across various categories of transformer faults and even on training datasets with extremely unbalanced data quantity where the average accuracy is as high as 95.28%.
更多查看译文
关键词
Data-sharing strategy,differential privacy (DP),power transformer fault diagnosis,privacy-preserving federated learning (FL),unbalanced data
AI 理解论文
溯源树
样例
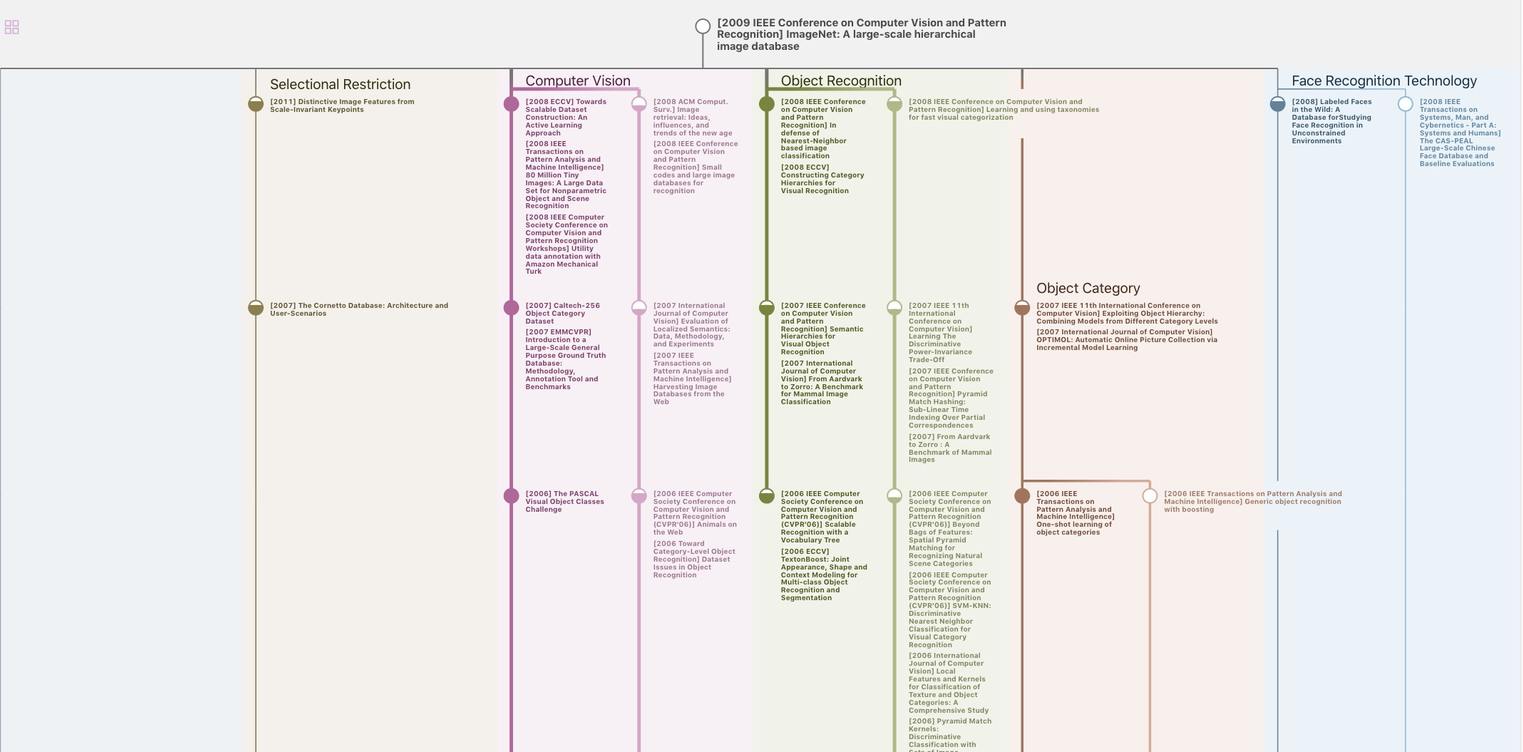
生成溯源树,研究论文发展脉络
Chat Paper
正在生成论文摘要