FHCPL: An Intelligent Fixed-Horizon Constrained Policy Learning System for Risk-Sensitive Industrial Scenario
IEEE TRANSACTIONS ON INDUSTRIAL INFORMATICS(2024)
Abstract
In many industrial scenarios, safety is a crucial factor to consider. In this article, we focus on the safe reinforcement learning problem that maximizes total rewards while enabling agents to avoid risks. We propose an intelligent fixed-horizon constrained policy learning (FHCPL) system, which allows agents to obtain high returns while maintaining risk avoidance behaviors. For discrete cases, a two-stage policy iteration algorithm, named fixed-horizon constrained policy iteration, is proposed, in which the safety of the learned policy is guaranteed. In the first stage, a policy that satisfies the safety constraint is obtained. In the second stage, a final learned policy that can get high returns while satisfying the safety constraint is reached. For continuous cases, we present the fixed-horizon constrained policy optimization algorithm. Empirical results demonstrate that, with the advantage of the fixed-horizon risk, the FHCPL achieves superior performance in terms of reward maximization and risk avoidance.
MoreTranslated text
Key words
Fixed-horizon constraint,policy learning,reinforcement learning (RL),risk-sensitive industrial scenario,safe RL
AI Read Science
Must-Reading Tree
Example
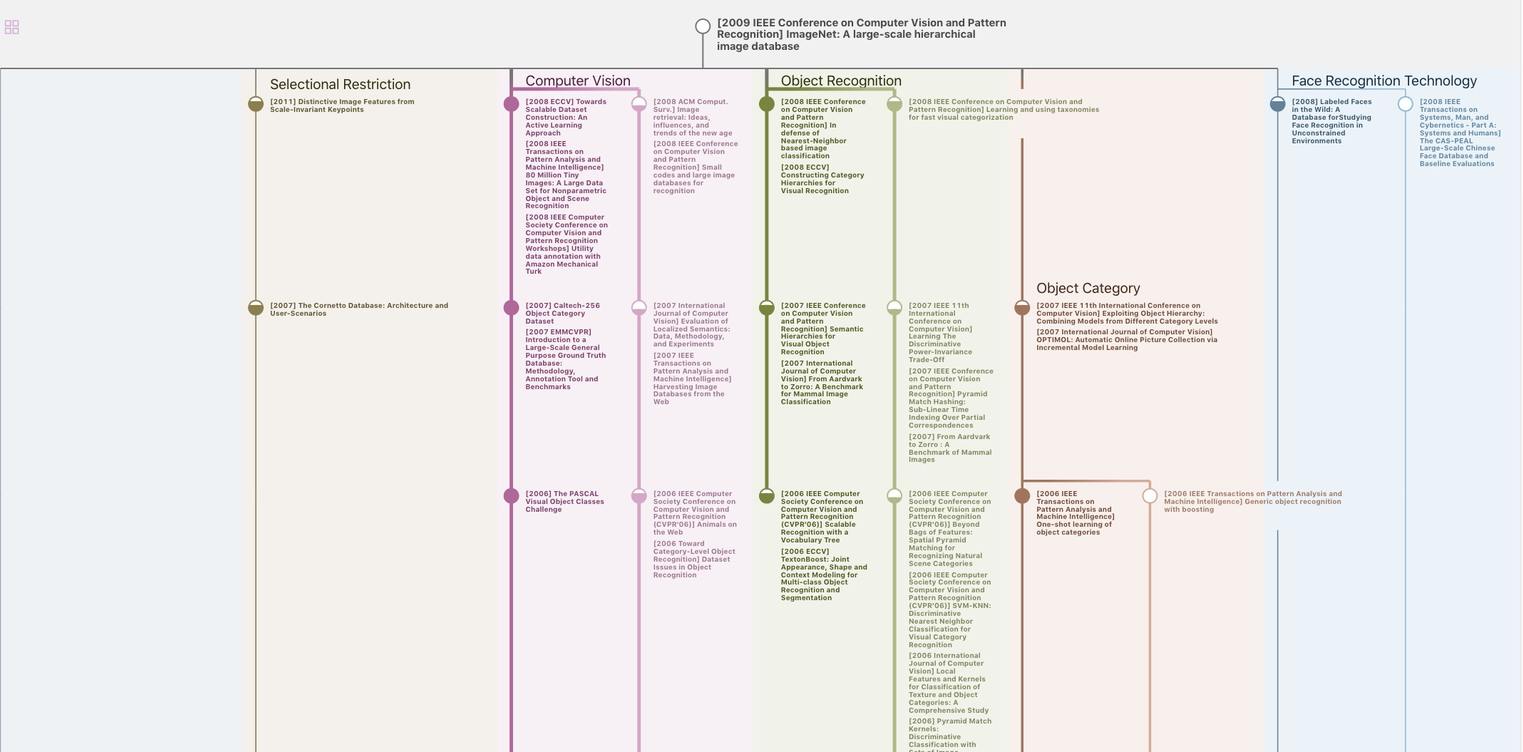
Generate MRT to find the research sequence of this paper
Chat Paper
Summary is being generated by the instructions you defined