Inter- to Intradomain: A Progressive Adaptation Method for Machine Fault Diagnosis
IEEE TRANSACTIONS ON INDUSTRIAL INFORMATICS(2023)
摘要
Domain adaptation technologies have been successfully and increasingly applied to machine fault diagnosis. Regardless of the performance gains achieved, almost all approaches focus on interdomain adaptation and ignore the intradomain divergence of the target domain itself. Such intradivergence will cause the model not to adapt to all target domain data, resulting in inferior diagnosis outcomes. To address the abovementioned issue, a progressive adaptation method is proposed for machine fault diagnosis, diminishing the discrepancies from inter- to intradomain to realize more excellent performance. Specifically, smoothness-induced conditional adversarial learning is first deployed to solve the interdomain distribution discrepancy. After that, an adaptive screening mechanism is presented to split the target domain into an easy subset and a hard subset. Intradomain adaptation is then designed to further enhance diagnosis accuracy, in which task loss sharpness, data class imbalance, and label noise are considered simultaneously. The proposed intradomain adaptation is also plug-and-play and can effectively benefit most current approaches. We examine the proposed method on extensive fault diagnosis tasks and compare it with other competing methods from different perspectives, the comprehensive evidence demonstrates the efficacy and superiority of our approach.
更多查看译文
关键词
Intelligent fault diagnosis,interdomain adaptation,intradomain adaptation,machinery
AI 理解论文
溯源树
样例
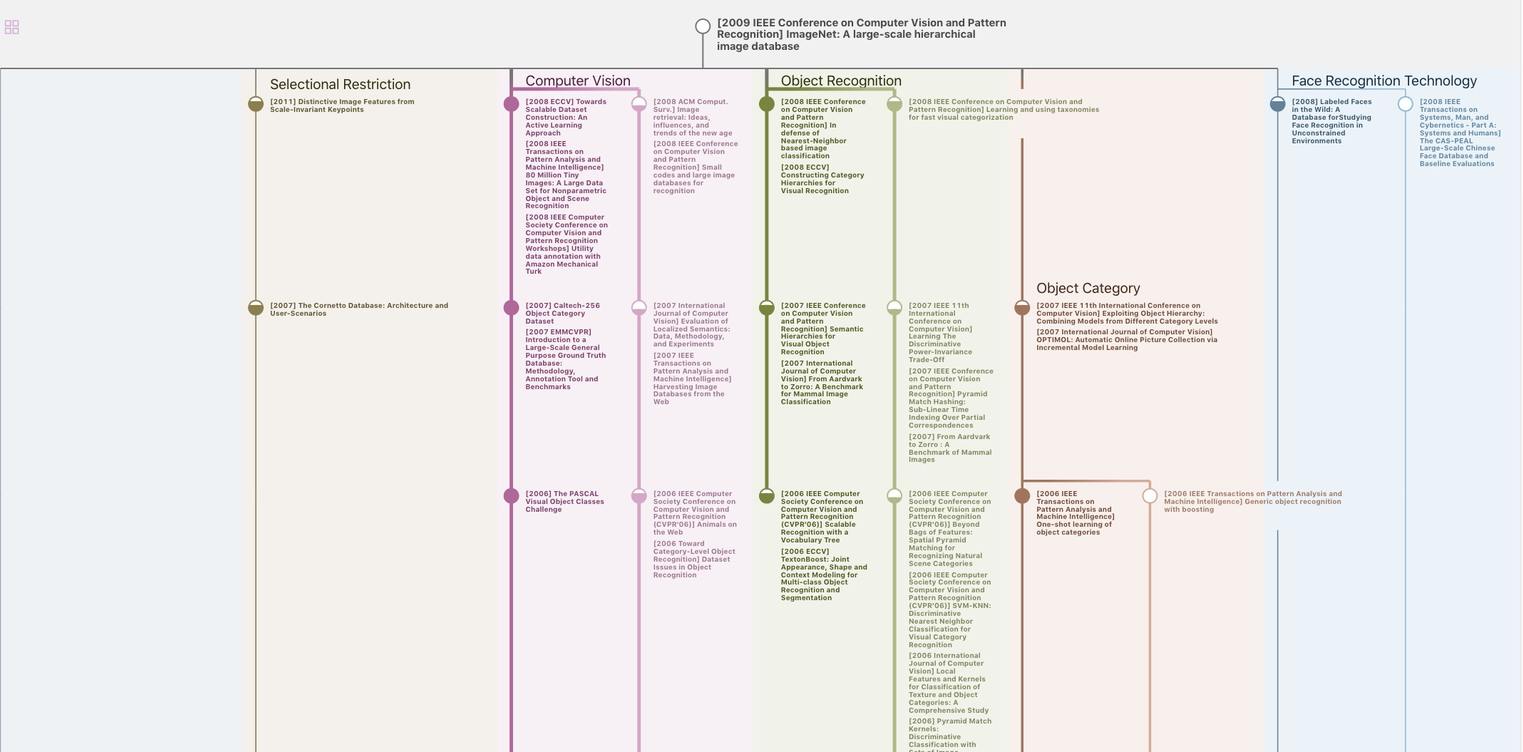
生成溯源树,研究论文发展脉络
Chat Paper
正在生成论文摘要