Continual Learning for Robotic Grasping Detection With Knowledge Transferring
IEEE TRANSACTIONS ON INDUSTRIAL ELECTRONICS(2023)
摘要
Massive data-driven robotic grasping techniques require the robots to grasp a specific object conditioned on the predicted grasping postures generated from a single well-trained grasping detection network. Despite having attained near-perfect performance, two major defects still plague these networks. First and foremost, obtaining a dataset that encompasses various grasping scenarios for training one grasping detection network is a time-consuming and labor-intensive task for researchers. Second, these grasping detection networks often exhibit poor generalization capabilities. They may not be rapidly trained using the data acquired from new scenarios, while simultaneously maintaining their previous grasping performance. In order to effectively tackle the aforementioned challenges, this article presents teacher-student architecture with a selective knowledge module to distill knowledge for continual learning in robotic grasping detection. Additionally, we refine the grasping detection network by introducing the residual feature connections to facilitate the acquisition and utilization of feature information and improve the overall performance. Extensive experiments on both continual learning and original grasping detection datasets in a real robotic grasping scene demonstrate the effectiveness and superiority of our architecture.
更多查看译文
关键词
Continual learning,grasping detection,knowledge distill,robotic grasping
AI 理解论文
溯源树
样例
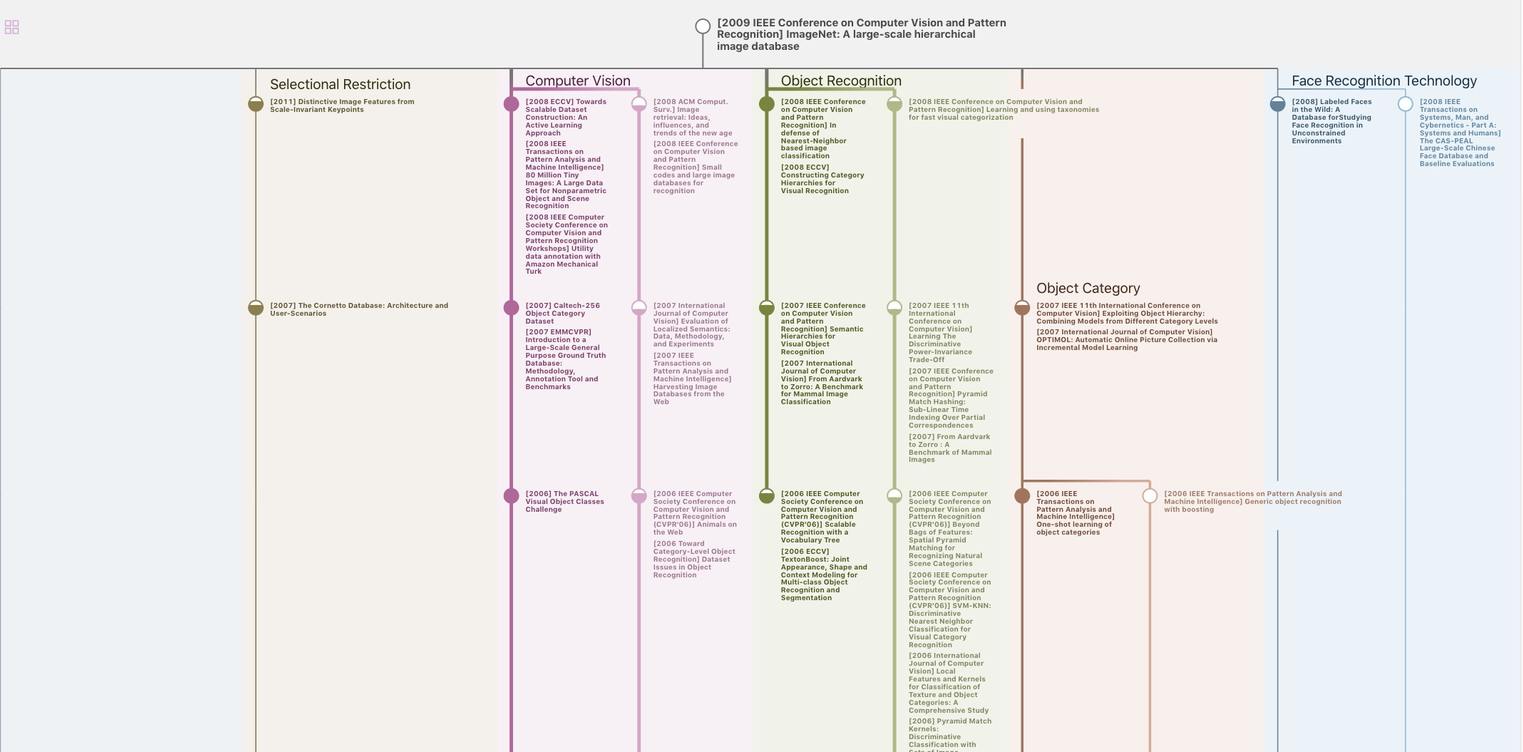
生成溯源树,研究论文发展脉络
Chat Paper
正在生成论文摘要