PMSA-DyTr: Prior-Modulated and Semantic-Aligned Dynamic Transformer for Strip Steel Defect Detection
IEEE Transactions on Industrial Informatics(2024)
关键词
Automated visual inspection (AVI),encoder-decoder network,salient detection,steel strip,transformer
AI 理解论文
溯源树
样例
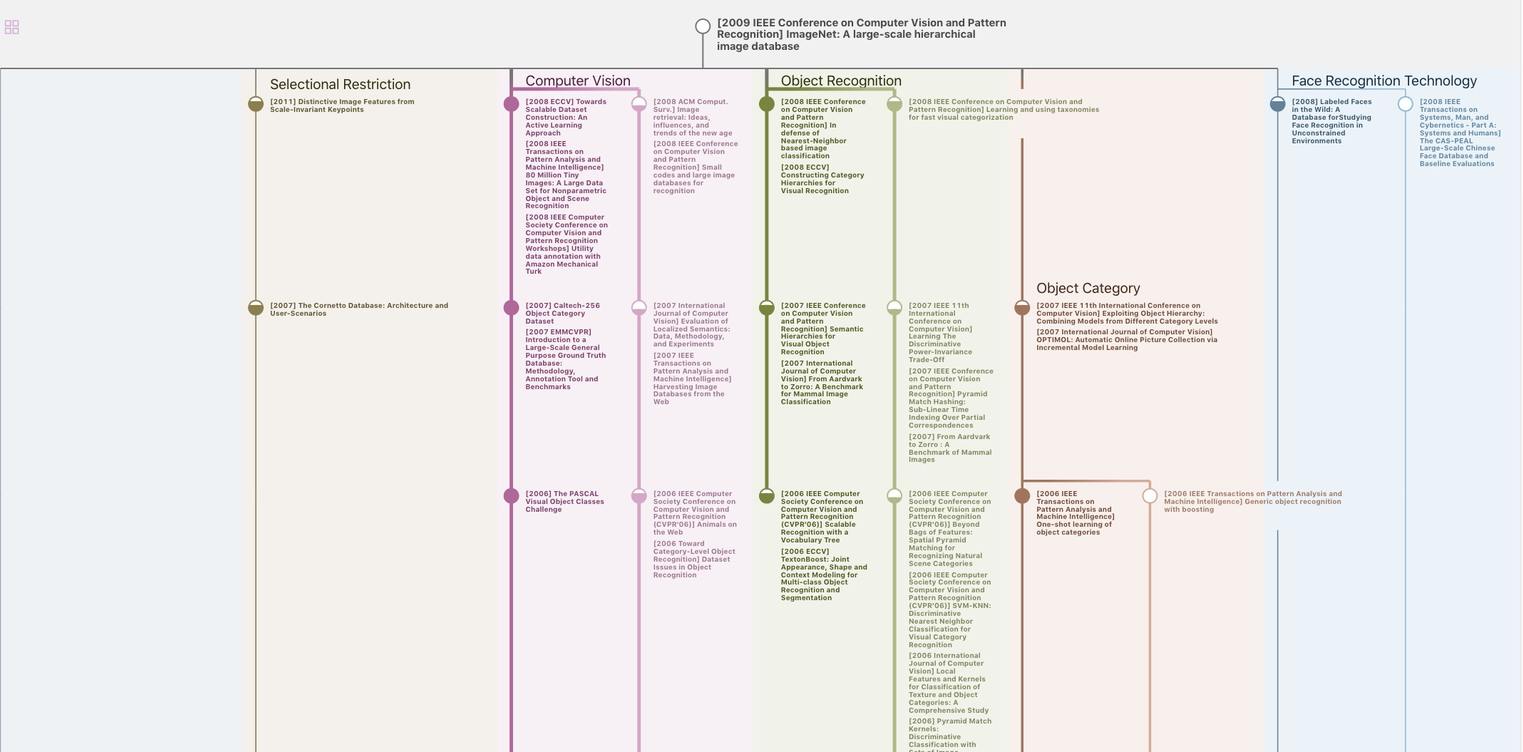
生成溯源树,研究论文发展脉络
Chat Paper
正在生成论文摘要