Watch Your Speed: Injecting Malicious Voice Commands via Time-Scale Modification.
IEEE Trans. Inf. Forensics Secur.(2024)
摘要
Existing adversarial example (AE) attacks against automatic speech recognition (ASR) systems focus on adding deliberate noises to input audio. In this paper, we propose a new attack that purely speeds up or slows down original audio instead of adding perturbations, and we call it Time-Scale Modification Adversarial Example (TSMAE). By investigating the impact of speed variation on 100, 000 pieces of audio clips, we found that misrecognition manifests in three categories: delete, substitution, and insertion. These are the accumulated results caused by the misrecognition of both the acoustic and language models inside an ASR system. Despite the challenges, i.e., ASR systems are typically black-box and reveal no gradient information, we managed to launch one-segment untargeted and targeted TSMAE attacks based on particle swarm optimization algorithms. Our untargeted attacks only require modifying the speed of one segment (e.g., 20 ms), and our targeted attacks can generate meaningful yet benign audio to cause an ASR system to output a malicious output, e.g.,
“open the door”
. We validate the feasibility of TSMAE on two open-source ASR models (e.g., DeepSpeech and Sphinx) and four commercial ones (e.g., IBM, Google, Baidu, and iFLYTEK). Results show that our untargeted attack can successfully attack all 6 ASR models with one segment modification, and our targeted attack is robust to various factors, such as model versions and speech sources. Finally, both attacks can bypass existing open-source defense methods, and our insights call attention to the defense’s focus from coping with perturbation to emerging adversarial example attacks.
更多查看译文
关键词
Speech recognition,time-scale modification,adversarial machine learning
AI 理解论文
溯源树
样例
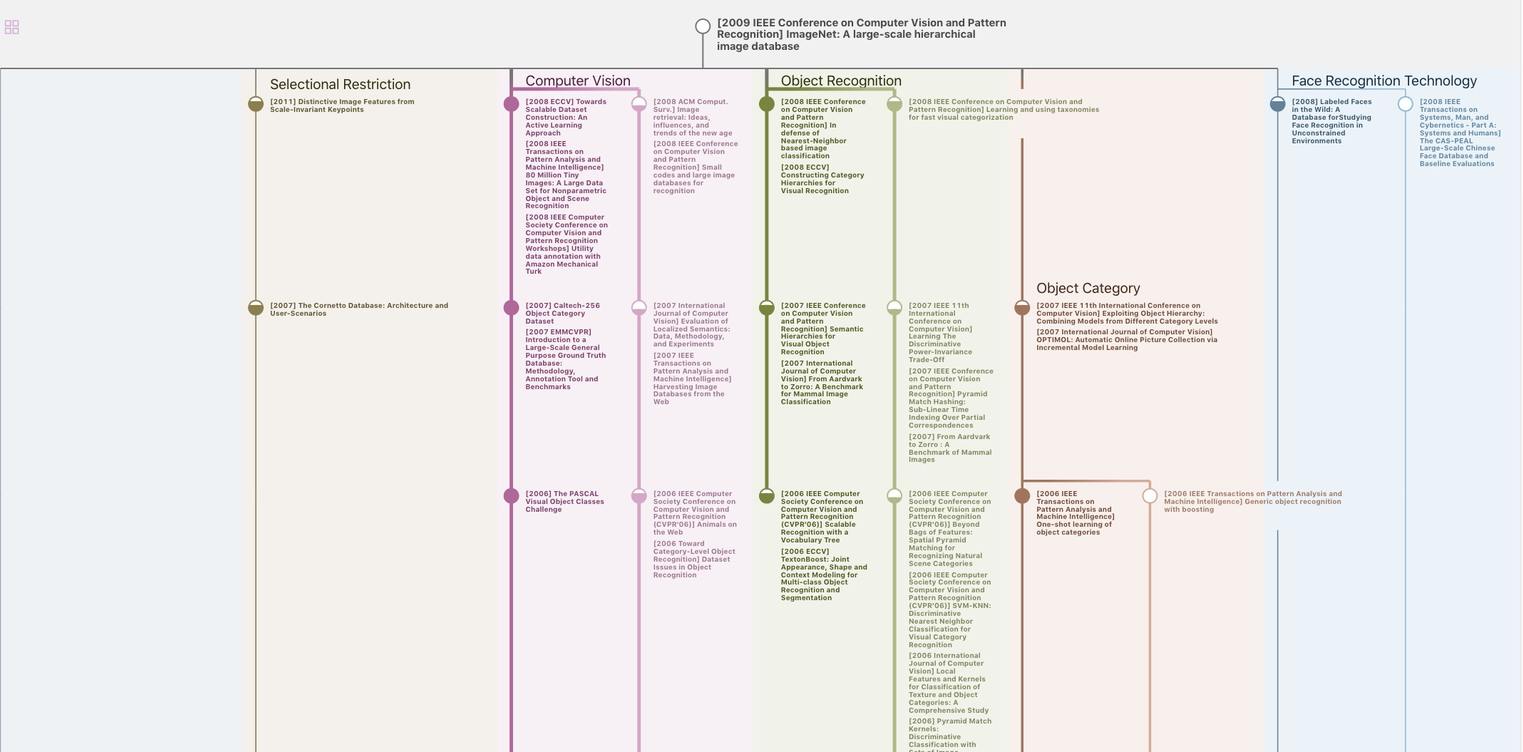
生成溯源树,研究论文发展脉络
Chat Paper
正在生成论文摘要