RoseAgg: Robust Defense Against Targeted Collusion Attacks in Federated Learning.
IEEE Trans. Inf. Forensics Secur.(2024)
摘要
Recent defense approaches against targeted model poisoning attacks aim to prevent specific prediction failures in federated learning (FL). However, these defenses remain susceptible to targeted collusion attacks, particularly under conditions of high proportions of malicious clients and attack density. To address these vulnerabilities, we propose RoseAgg, which dynamically identifies a plausible clean ingredient from local updates and leverages it to constrain the influence of poisoned updates. Firstly, RoseAgg recognizes and confines common characteristics found in poisoned updates, such as scaled-up magnitudes or similar directional contributions. Furthermore, RoseAgg dynamically extracts a plausible clean ingredient using a dimension-reduction method. This clean ingredient becomes the foundation for the server to bootstrap credit scores for each local update, ensuring the dominance of benign updates over poisoned ones. Ultimately, the server computes a weighted average of local updates based on credit scores, generating a global update for refining the global model. Comprehensive evaluations on four benchmark datasets showcase RoseAgg’s effectiveness against seven advanced attacks. The code is available at https://github.com/SleepedCat/RoseAgg.
更多查看译文
关键词
Federated Learning,Targeted Model Poisoning,Collusion Attacks,Robust Defense
AI 理解论文
溯源树
样例
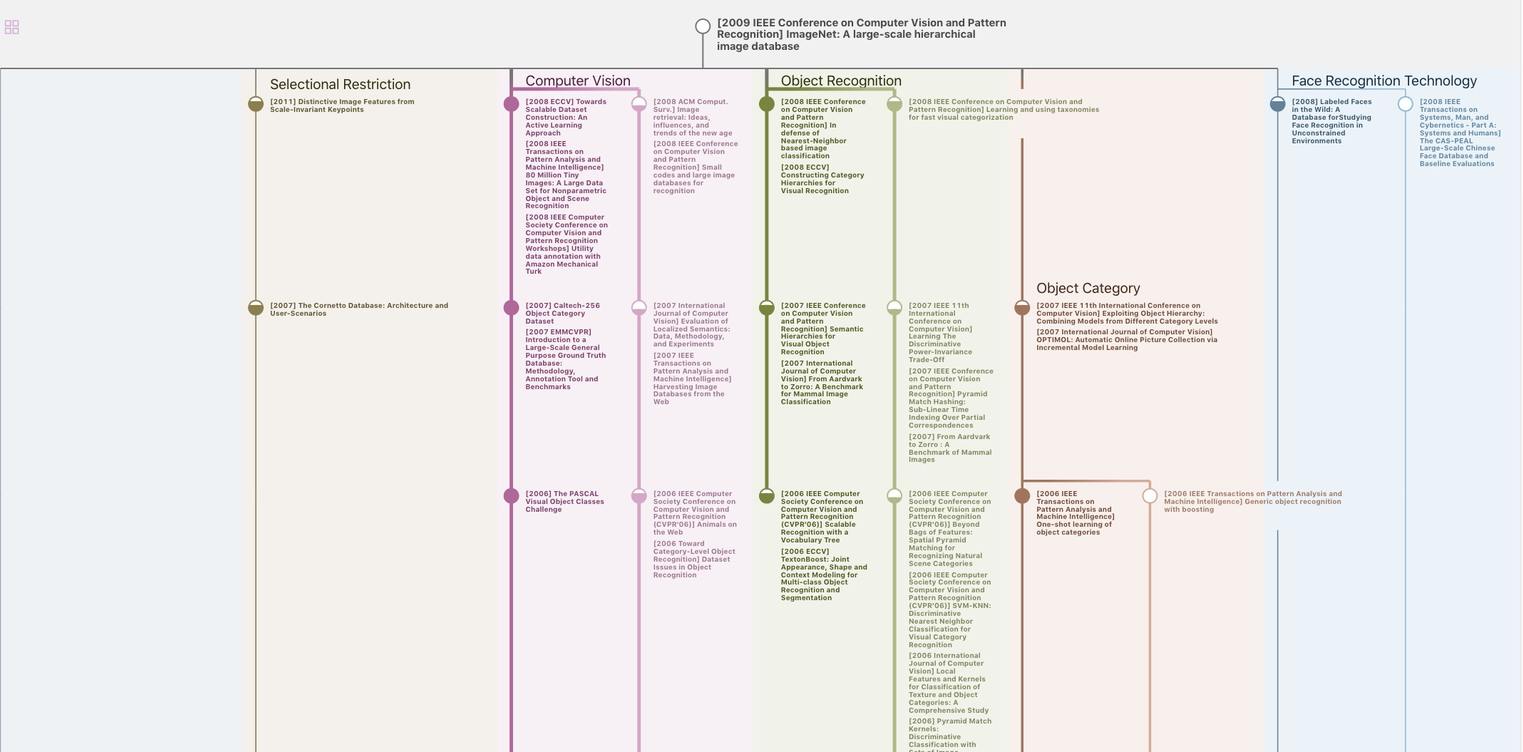
生成溯源树,研究论文发展脉络
Chat Paper
正在生成论文摘要