Joint Cost Learning and Payload Allocation With Image-Wise Attention for Batch Steganography.
IEEE Trans. Inf. Forensics Secur.(2024)
摘要
In recent years, although cost learning methods have made great progress in single-image steganography, its development in batch steganography is relatively slower, which is a more practical communication scenario in the real world. The difficulties are capturing the full view of the image batch and building connections between cost learning and payload allocation by neural networks. To address the issues, this paper proposes a cost learning framework for batch steganography called JoCoP (Joint Cost Learning and Payload Allocation), wherein the policy network is designed to learn the optimal embedding policies for a batch of images via the collaboration between a cost learning module and a payload allocation module. In specific layers of the policy network, in the cost learning module, the intermediate feature maps of embedding costs are extracted for different images independently, which are sent to the payload allocation module. In the payload allocation module, to implement implicit payload allocation, the feature maps corresponding to different images within the same batch are adjusted by an image-wise attention mechanism. Afterwards, these adjusted feature maps are returned to the cost learning module for subsequent feature extraction in the next layer. Owing to the collaboration between the two modules and the batch-level receptive field in the image-wise attention mechanism, the embedding costs and the payload allocation can be jointly optimized in an end-to-end manner. Experimental results show that the proposed JoCoP outperforms existing methods against both single-image steganalyzers and pooled steganalyzers based on feature extraction and convolutional neural networks.
更多查看译文
关键词
Batch steganography,cost learning,payload allocation,image-wise attention
AI 理解论文
溯源树
样例
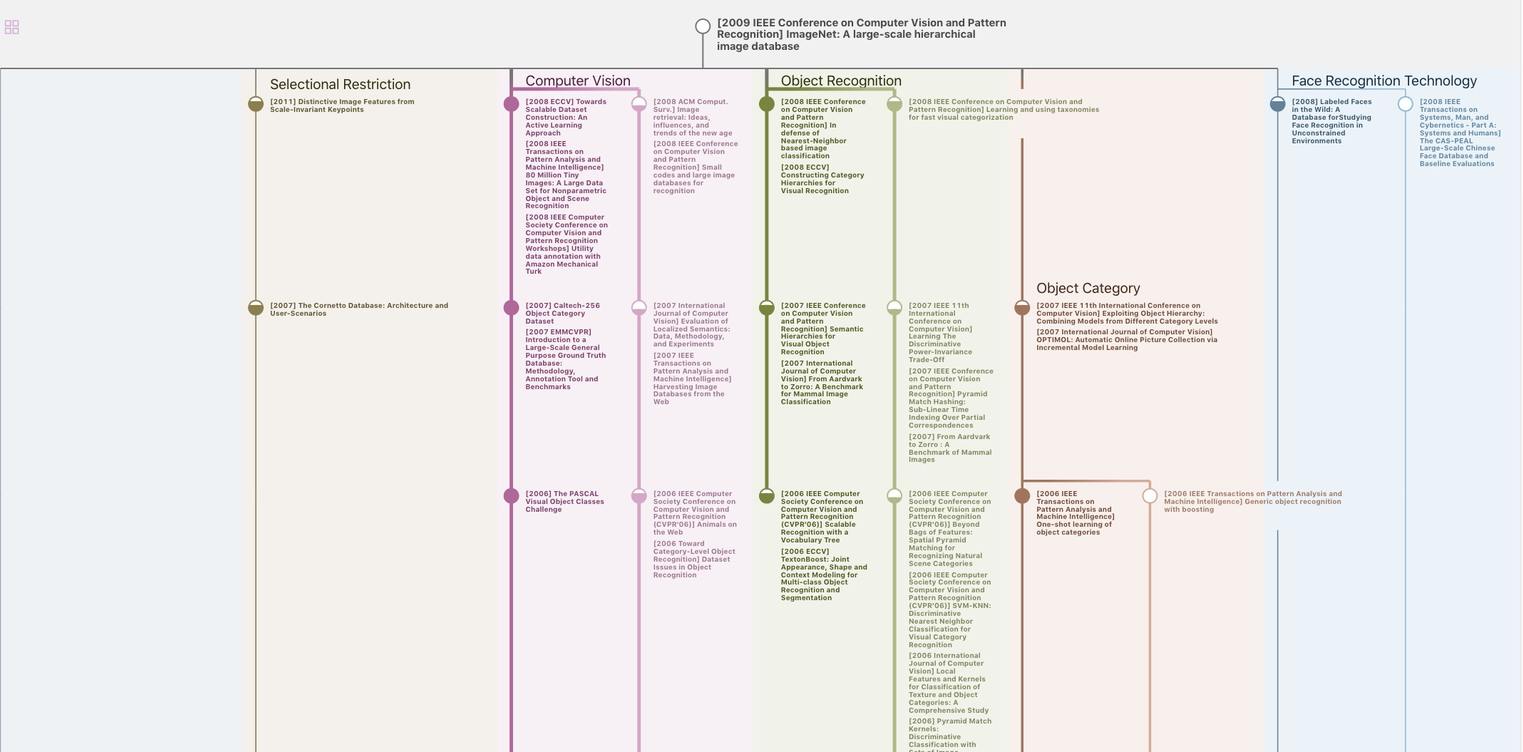
生成溯源树,研究论文发展脉络
Chat Paper
正在生成论文摘要