Unlabeled Data Assistant: Improving Mask Robustness for Face Recognition
IEEE TRANSACTIONS ON INFORMATION FORENSICS AND SECURITY(2024)
摘要
The existing masked face recognition algorithms almost tend to adopt synthetic masked face datasets for training. However, these models are limited as they rely on existing mask augmentation methods, which contain few mask patterns and cannot simulate shadows and textures in realistic scenes. To overcome this limitation, we propose a semi-supervised face recognition framework to fully exploit unlabeled real masked face samples, improving the mask robustness of the recognition model. More specifically, unlike the original face embedding network, we design a part-aware network to explore multi-region face representation based on the face structure. In this way, we obtain multiple face sub-embeddings, which correspond to different regions of the face, including the upper half, the lower half and the whole. Crucially, we use the norm of the sub-embedding to represent the activation state of the facial region features. For the input unlabeled masked face image, we restrict the sub-embedding norm of its lower half to weaken the face feature representation of the occluded area. For normal face samples, their partial features are kept activated by maintaining the sub-embedding norm, which guides the deep network does not ignore the available information. Moreover, we employ the margin-based recognition loss for normal samples to ensure that the model is sufficiently discriminative for normal facial features. Extensive experimental results on both normal and real masked face datasets show that our approach significantly outperforms the state-of-the-arts.
更多查看译文
关键词
Unlabeled data,masked face recognition,semi-supervision
AI 理解论文
溯源树
样例
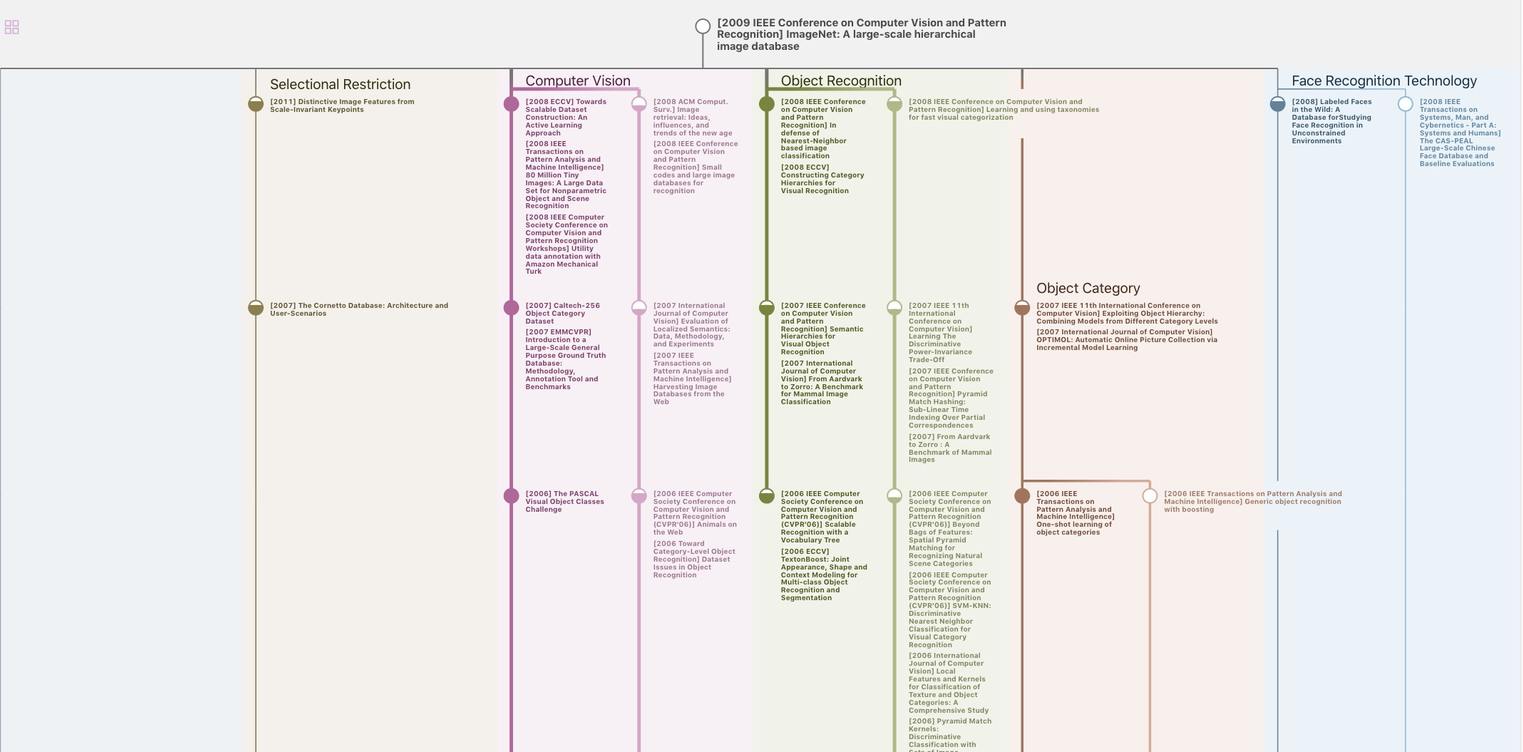
生成溯源树,研究论文发展脉络
Chat Paper
正在生成论文摘要