Biscale Convolutional Self-Attention Network for Hyperspectral Coastal Wetlands Classification
IEEE GEOSCIENCE AND REMOTE SENSING LETTERS(2024)
摘要
Coastal wetlands classification is a hot but challenging issue. Hyperspectral image (HSI) can provide abundant spectral information for coastal wetlands, and deep learning excels at extracting abstract features. However, effectively leveraging global and local features to enhance the accuracy of coastal wetlands classification remains a significant challenge. In this letter, we propose a biscale convolutional self-attention network (termed as HyperBCS) for hyperspectral coastal wetlands classification. HyperBCS consists of biscale adding module (BSAM) and convolutional self-attention module (CSM). On the one hand, BSAM uses two branches to extract features of different scales. On the other hand, CSM is a paralleled structure of convolution and self-attention, which can effectively extract both local and global features. Experiments on two hyperspectral datasets captured by Zhuhai-1 satellite indicate that HyperBCS can improve the accuracy of hyperspectral coastal wetlands classification, showcasing the highest accuracy (OA = 98.29% and 96.82%, Kappa = 0.976 and 0.958 in two datasets) compared with other methods.
更多查看译文
关键词
Coastal wetlands classification,convolution,deep learning,hyperspectral image (HSI),self-attention mechanism
AI 理解论文
溯源树
样例
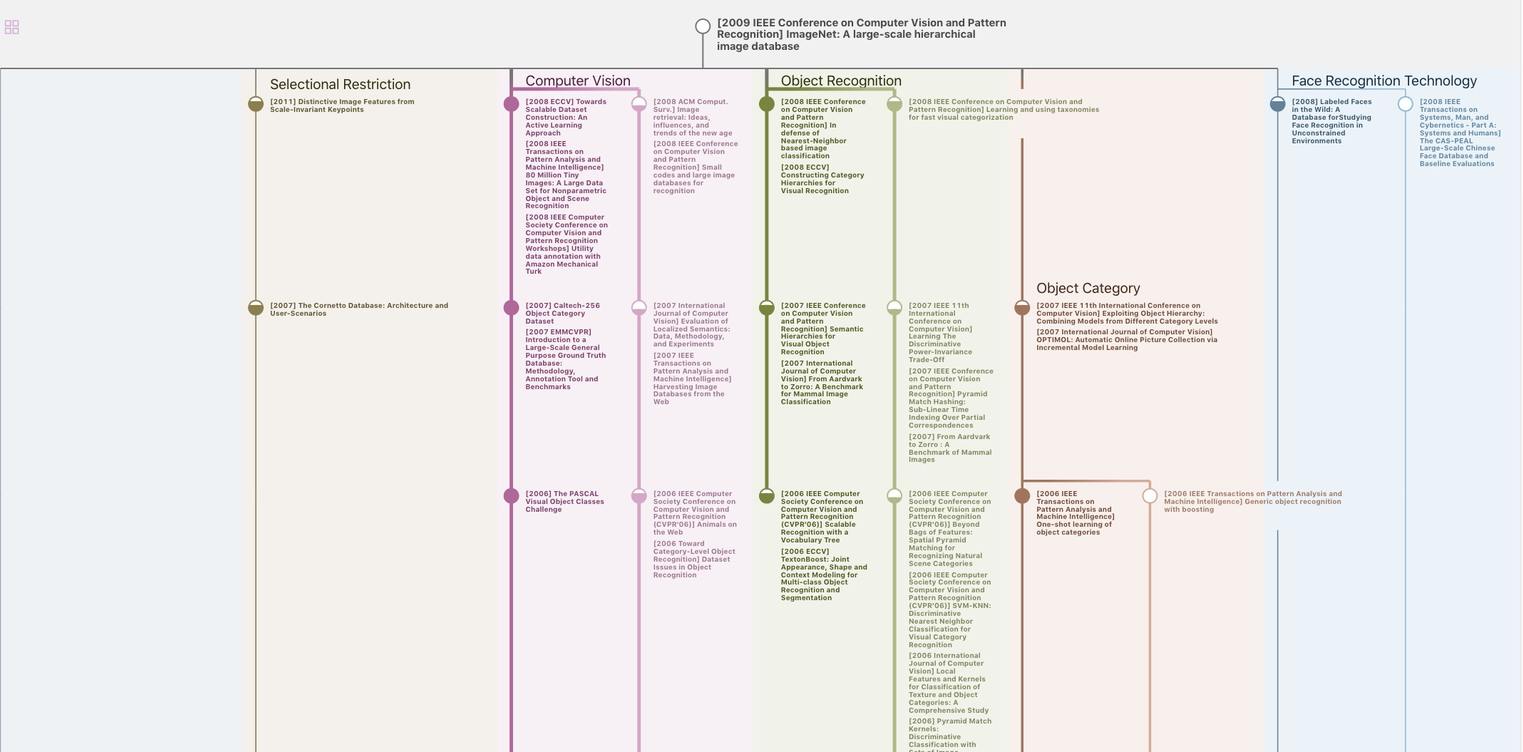
生成溯源树,研究论文发展脉络
Chat Paper
正在生成论文摘要