An Evolving Preference-Based Recommendation System
IEEE TRANSACTIONS ON EMERGING TOPICS IN COMPUTATIONAL INTELLIGENCE(2023)
摘要
In this "Info-plosion" era, recommendation systems (or recommenders) play a significant role in finding interesting items in a surge of the on-line digital activity and e-commerce. Because of its practicability, the matrix factorization (MF) technique has been widely applied for recommendation systems. Prior MF-based studies on recommendations generally extract latent factors from users and items to make recommendations. However, user's preferences may change over time in real-world applications. In this paper, by integrating the transformer and matrix factorization techniques, a novel recommendation system, namely Evolution-Based Transformer Recommendation (Evo-TransRec), is developed to effectively describe the evolution of user preferences over time. Several optimization techniques are equipped to Evo-TransRec to capture the evolution relations and predict the user preference. The experimental results show that Evo-TransRec outperforms all the state-of-the-art baselines on real datasets to demonstrate the practicability.
更多查看译文
关键词
Deep learning,recommendation system,matrix factorization,transformer
AI 理解论文
溯源树
样例
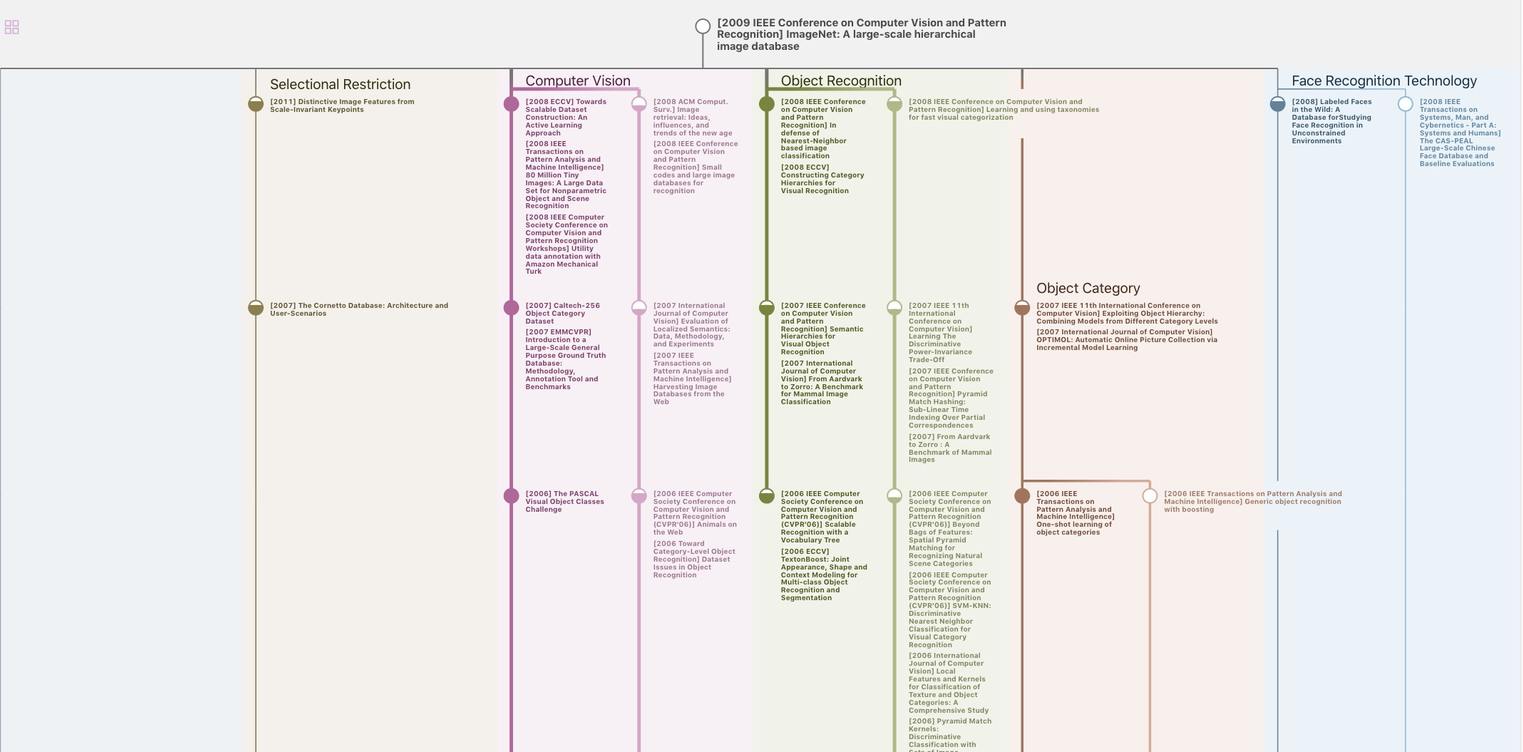
生成溯源树,研究论文发展脉络
Chat Paper
正在生成论文摘要