An Evolutionary Multitasking Method for High-Dimensional Receiver Operating Characteristic Convex Hull Maximization
IEEE TRANSACTIONS ON EMERGING TOPICS IN COMPUTATIONAL INTELLIGENCE(2024)
Abstract
Maximizing receiver operating characteristic convex hull (ROCCH) is a hot research topic of binary classification, since it can obtain good classifiers under either balanced or imbalanced situation. Recently, evolutionary algorithms (EAs) especially multi-objective evolutionary algorithms (MOEAs) have shown their competitiveness in addressing the problem of ROCCH maximization. Thus, a series of MOEAs with promising performance have been proposed to tackle it. However, designing a MOEA for high-dimensional ROOCH maximization is much more challenging due to the "curse of dimension". To this end, in this paper, an evolutionary multitasking approach (termed as EMT-ROCCH) is proposed, where a low-dimensional ROCCH maximization task T-a is constructed to assist the original high-dimensional task T-o. Specifically, in EMT-ROCCH, a low-dimensional assisted task T-a is firstly created. Then, two populations, P-a and P-o, are used to evolve tasks T-a and T-o, respectively. During the evolution, a knowledge transfer from P-a to P-o is designed to transfer the useful knowledge from P-a to accelerate the convergence of P-o. Moreover, a knowledge transfer from P-o to P-a is developed to utilize the useful knowledge in P-o to repair the individuals in P-a, aiming to avoid P-a being trapped into the local optima. Experiment results on 12 high-dimensional datasets have shown that compared with the state-of-the-arts, the proposed EMT-ROCCH could achieve ROCCH with higher quality.
MoreTranslated text
Key words
Evolutionary computation,Evolutionary multitasking,ROC convex hull,multi-objective evolutionary algorithm,knowledge transfer,classification
AI Read Science
Must-Reading Tree
Example
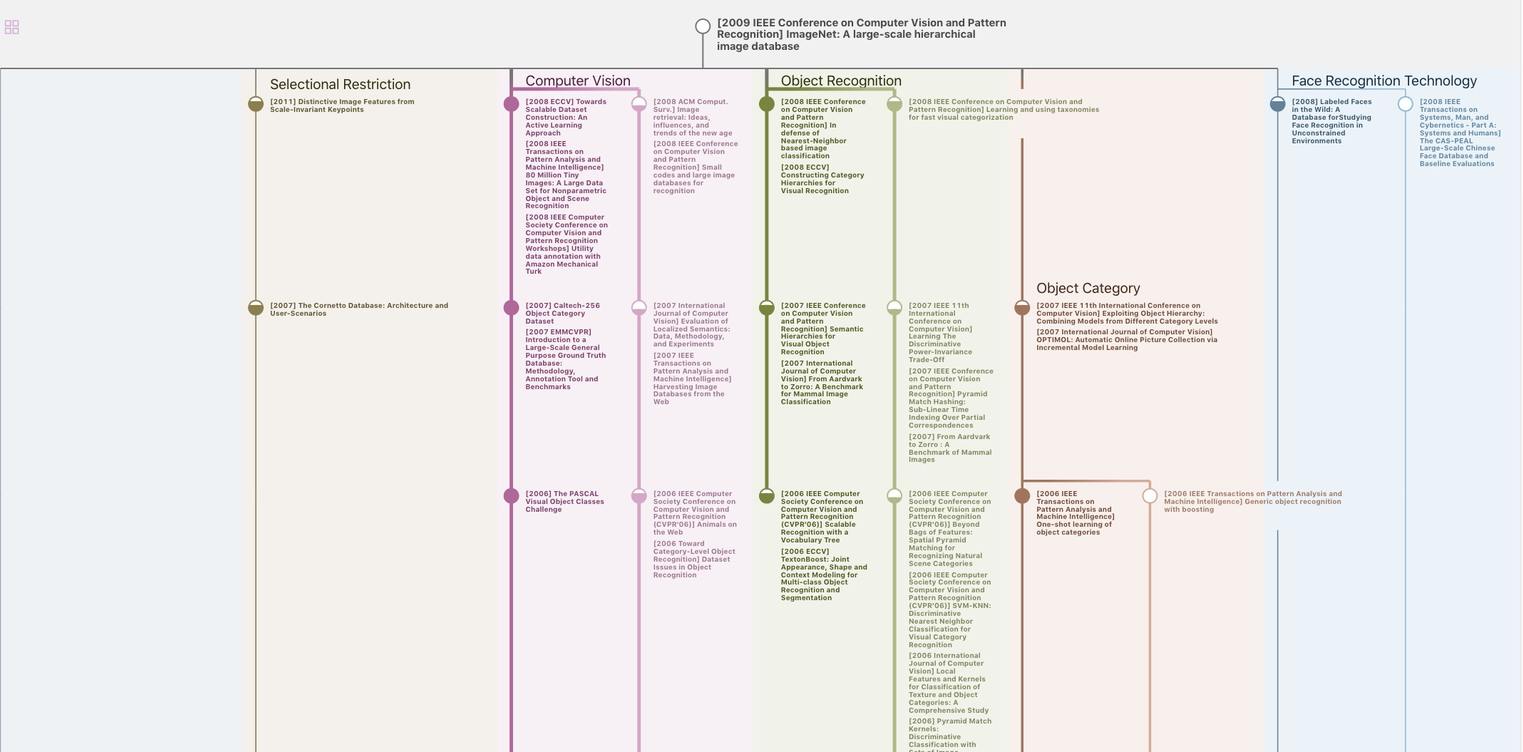
Generate MRT to find the research sequence of this paper
Chat Paper
Summary is being generated by the instructions you defined