Heterogeneous Data-Driven Failure Diagnosis for Microservice-Based Industrial Clouds Towards Consumer Digital Ecosystems
IEEE Transactions on Consumer Electronics(2023)
摘要
Consumer digital ecosystems include a large volume of different types of applications, and those applications are usually deployed in industrial cloud computing systems. Currently, microservices are one of the most prevailing architectures for industrial clouds. Similar to other architectures, microservices may also produce failures, so failure diagnosis for microservices becomes an inevitable problem in industrial clouds. A majority of existing methods focus on statistical analysis for monitoring data or system topological structure. However, because these methods usually only harness service-level or machine-level metrics, they cannot complete fine-grained failure diagnosis, increasing the running risk of microservice-based industrial clouds. To tackle this issue, in this paper, we design a novel graph structure to represent failure dependencies, especially the heterogeneity, and name it as the heterogeneous failure dependence graph (HFDG). We propose a framework to inform engineers which type of and where failures occur in industrial clouds. The HFDG can be used to mine the propagation of failures between different types of components. We also propose a novel neural network model based on attention mechanism and heterogeneous graph neural network, to fully leverage the metric data and HFDG. We performed experiments on three large-scale public datasets from real-world microservices-based systems. The experimental results demonstrate the superior performance of our model compared to well-known baselines.
更多查看译文
关键词
Heterogeneous Data,Consumer Digital Ecosystems,Industrial Cloud,Failure Diagnosis,Microservice
AI 理解论文
溯源树
样例
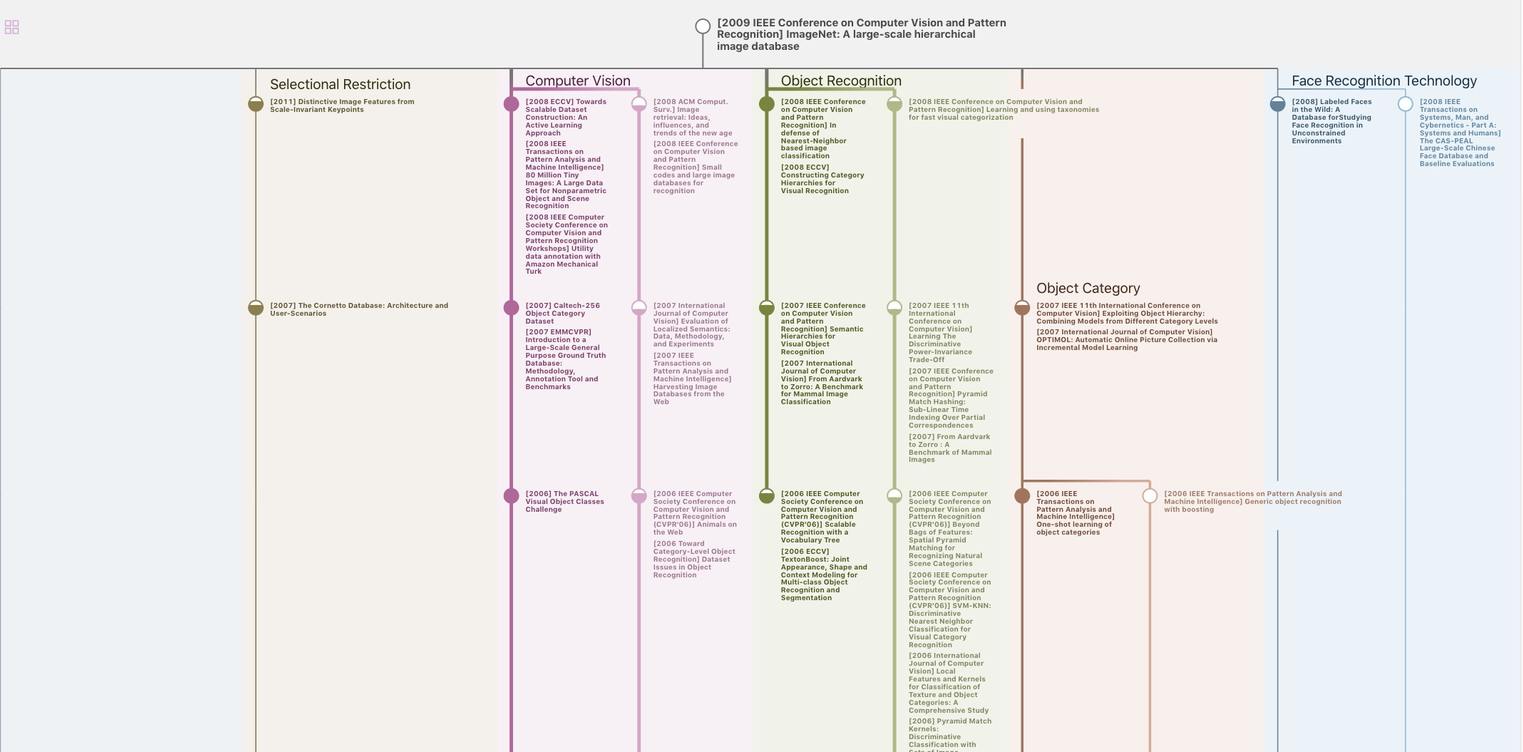
生成溯源树,研究论文发展脉络
Chat Paper
正在生成论文摘要