FedPIA: Parameter Importance-Based Optimized Federated Learning to Efficiently Process Non-IID Data on Consumer Electronic Devices
IEEE Transactions on Consumer Electronics(2023)
摘要
Federated learning is a distributed machine learning method for learning consumer data generated by consumer electronic devices. It provides personalized intelligent services for users while maintaining data privacy by sharing models instead of user data. It is challenging for these methods to learn data efficiently and with high quality. The performance and quality of the model in federated learning are affected by differences in data from different devices, which can lead to divergence between local and global models. Therefore, this paper proposes a parameter importance-based optimization federated learning method, FedPIA. First, two key indicators, including the rate of parameter change and the acceleration rate of parameter change, are provided to evaluate the importance of model parameters, which can reflect the divergence of the data from different devices. Then, a regular term is created based on these indicators to construct a new loss function, reducing the divergence between local and global models. Last, this paper efficiently trains a high-quality model on consumer data with this optimization approach. Experiments show that FedPIA is significantly better than FedCL, FedAvg, and Scaffold in accuracy, F1 scores and communication overhead.
更多查看译文
关键词
Consumer Electronics,Privacy Protection,Federated Learning,Non-IID
AI 理解论文
溯源树
样例
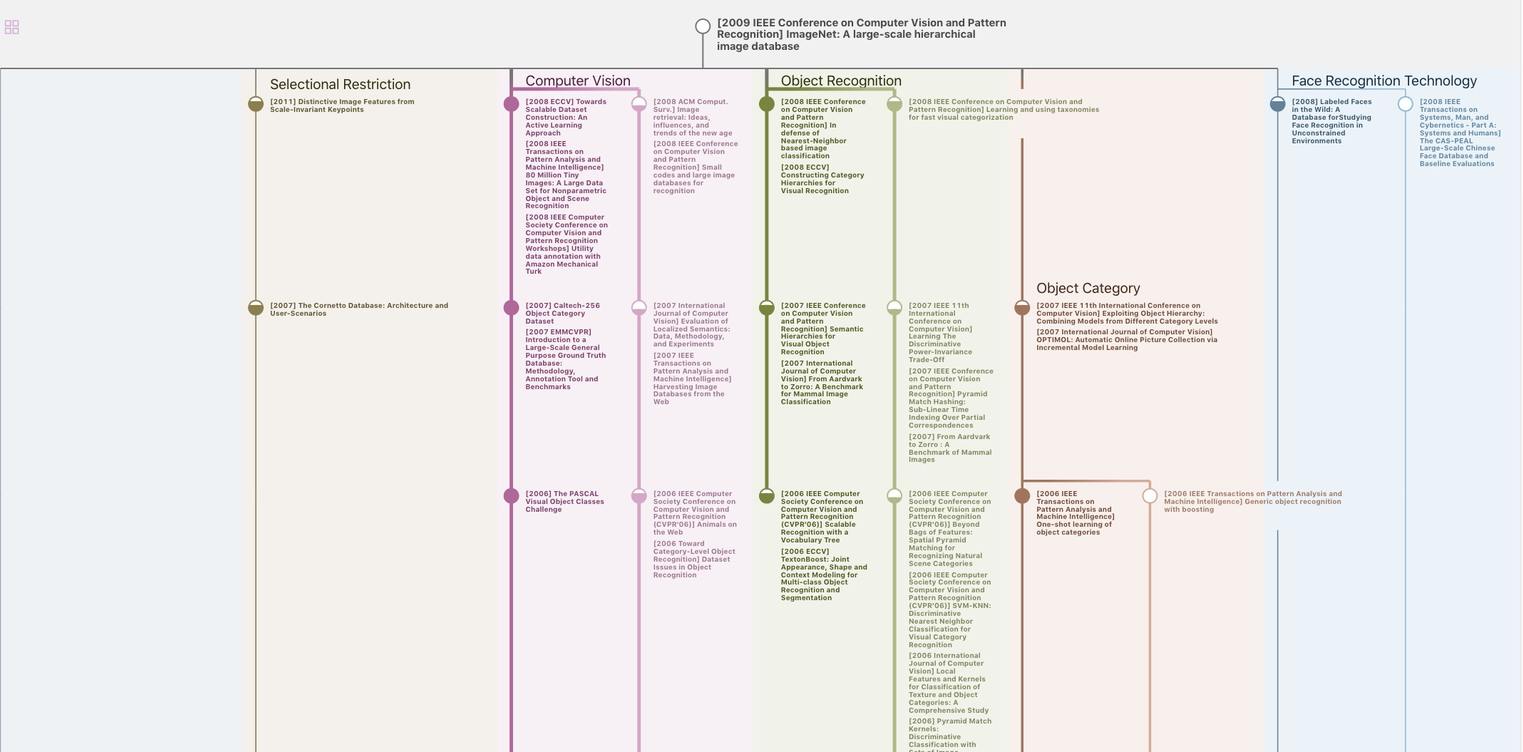
生成溯源树,研究论文发展脉络
Chat Paper
正在生成论文摘要