Explainable Cyberbullying Detection in Hinglish: A Generative Approach
IEEE TRANSACTIONS ON COMPUTATIONAL SOCIAL SYSTEMS(2023)
摘要
The escalating prevalence of online cyberbullying and trolling across various social media platforms has becomes a pressing concern. Extensive research demonstrates the detrimental impact of cyberbullying on the mental well-being of its victims. Given the sheer volume of online content, manual identification of cyberbullying instances proves unfeasible, necessitating the development of automated cyberbullying detection methods. This challenge has attracted considerable attention within the natural language processing (NLP) community, owing to advancements in machine learning techniques. However, most of the methods fail to provide reasoning for their decisions which warrants the use of interpretable models that can explain the model's output in real-time. Interpretable models rather than black-box models with high performance are becoming popular adhering the "right to explanations" laws. Motivated by this, we create a cyberbullying corpus BullyExplain in code-mixed language, where a post has been annotated with four labels, i.e., bully, sentiment, target, and rationales (explainability). Current work addresses the task of explainable cyberbully detection and proposes a unified generative framework, BullyGen by redefining this multitask problem as a text-to-text generation task. Our framework is capable of not only detecting whether the text is a cyberbully or not but also provides reasoning by predicting rationale, target group and sentiment of the text. Experimental results illustrate the efficacy of our proposed model by outperforming the state-of-the-art and several baselines by a significant margin and conclude that text-to-text generation model could be a good alternative for multitask classification problems.
更多查看译文
关键词
Code-mixed,cyberbullying detection,explain-ability,multitask,sentiment,target
AI 理解论文
溯源树
样例
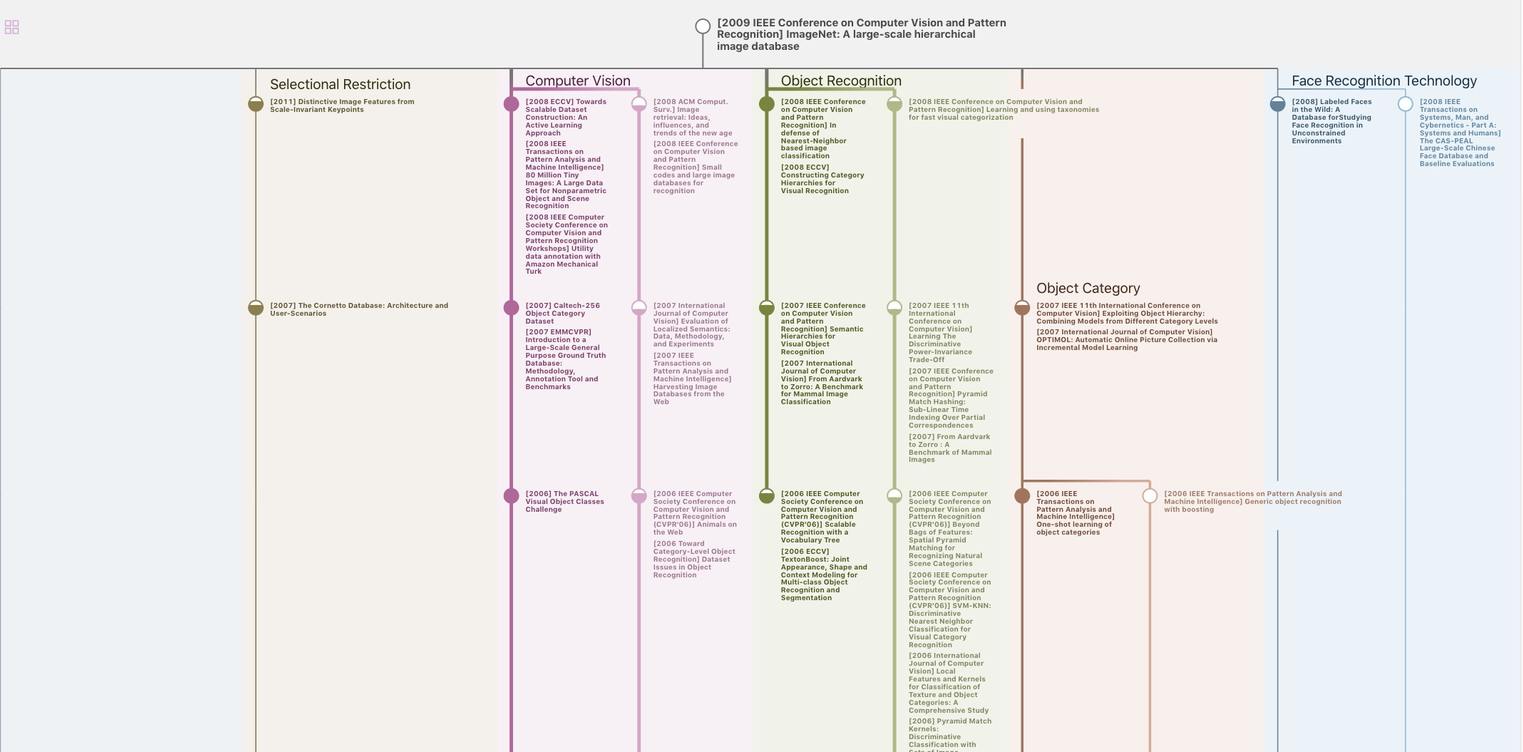
生成溯源树,研究论文发展脉络
Chat Paper
正在生成论文摘要