LeoVR: Motion-inspired Visual-LiDAR Fusion for Environment Depth Estimation
IEEE Transactions on Mobile Computing(2023)
摘要
Environment depth estimation by fusing camera and radar enables a broad spectrum of applications such as autonomous driving, environmental perception, context-aware localization and navigation. Various pioneering approaches have been proposed to achieve accurate and dense depth estimation by integrating vision and LiDAR through deep learning. However, due to the challenges of sparse sampling of in-vehicle LiDARs, high ground-truth annotation overhead, and severe dynamics in real environments, existing solutions have not yet achieved widespread deployment on commercial autonomous vehicles. In this paper, we propose LeoVR, a motion-inspired self-supervised visual-LiDAR fusion approach that enables accurate environment depth estimation. Leveraging the vehicle motion information, LeoVR employs two effective system frameworks to
$(i)$
optimize the depth estimation results, and
$(ii)$
provide supervision signals for DNN training. We fully implemented LeoVR on both a robotic testbed and a commercial vehicle and conducted extensive experiments over an 8-month period. The results demonstrate that LeoVR achieves remarkable performance with an average depth estimation error of 0.17
$m$
, outperforming existing state-of-the-art solutions by
$\gt $
45.9%. Besides, even cold-start in real environments by self-supervised training, LeoVR still achieves an average error of 0.2
$m$
, outperforming the related works by
$\gt $
47.8% and comparable to supervised training methods.
更多查看译文
关键词
Visual-LiDAR Fusion,Depth Estimation,Factor Graph,Self-Supervised Learning
AI 理解论文
溯源树
样例
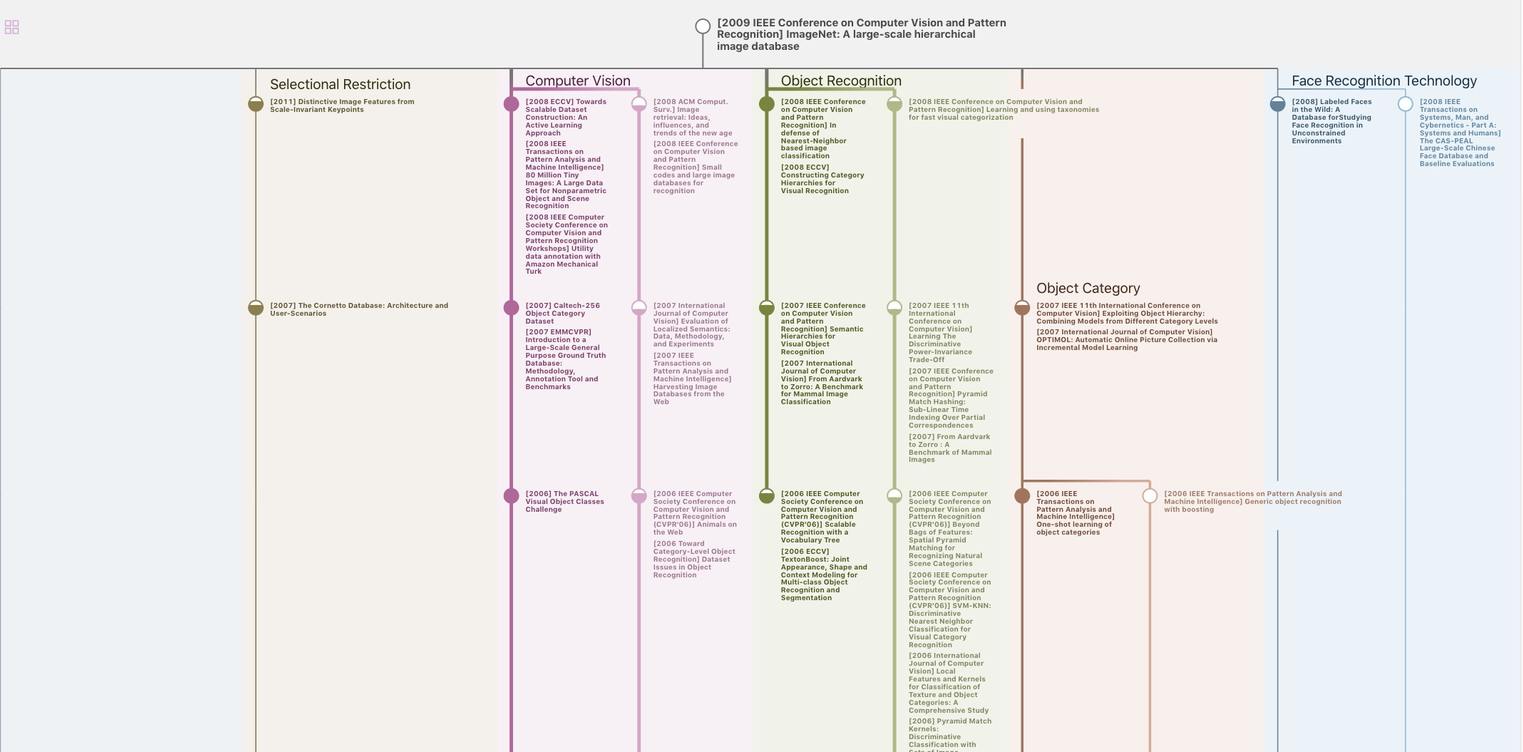
生成溯源树,研究论文发展脉络
Chat Paper
正在生成论文摘要