Fast Anomaly Detection for IoT Services Based on Multi-source Log Fusion
IEEE Internet of Things Journal(2023)
摘要
With the fast development of Internet of Things (IoT), anomaly detection has recently become a common concern for IoT smart applications. The circuit detection mode of all services to detect application anomaly is widely adopted. However, regularly collecting key performance indicators (KPIs) of all services under different clouds is a time-consuming task. Moreover, too many alerts in a short period of time will affect the processing speed of engineers. Recently, some researches of inferring the key service on the call paths can solve the above problems. However, it is still a challenge to locate dynamic and scattered key services accurately. In this paper, we propose a service inference-based anomaly detection approach (SIADA), which integrates three sources of logs: call, business and metric. SIADA leverages the deep graph representation with context-aware multi-graph fusion based on a recurrent encoder. This should to infer key services, and adopts Variational Auto-Encoder (VAE) with the flow model to detect multivariate time series anomalies for key services. We have conducted extensive experiments on the public dataset MicroSS. SIADA achieves the best average accuracy of 92% in service inference and best average F1-score of 0.98 in anomaly detection, which is improved by 12.17% and 6.42% compared with the best SOTA baseline, respectively. Moreover, the total detection time, network transmission and average alert number are reduced by 42.12%, 81.87%, and 83.5%, respectively.
更多查看译文
关键词
Fast anomaly detection,Key service inference,Log fusion,IoT service,Service operation
AI 理解论文
溯源树
样例
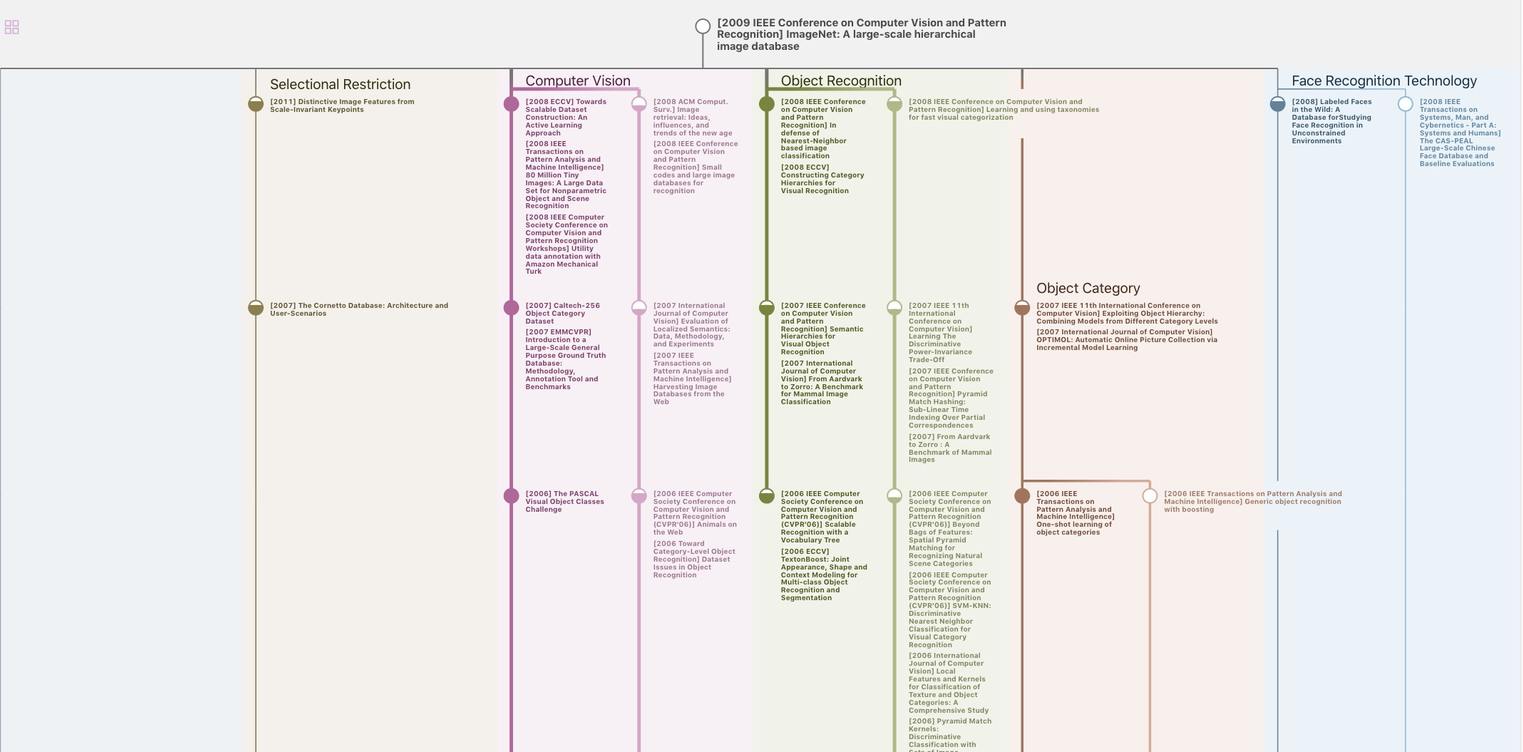
生成溯源树,研究论文发展脉络
Chat Paper
正在生成论文摘要