Federated Fuzzy Clustering for Decentralized Incomplete Longitudinal Behavioral Data.
IEEE internet of things journal(2023)
摘要
The use of medical data for machine learning, including unsupervised methods such as clustering, is often restricted by privacy regulations such as the Health Insurance Portability and Accountability Act (HIPAA). Medical data is sensitive and highly regulated and anonymization is often insufficient to protect a patient's identity. Traditional clustering algorithms are also unsuitable for longitudinal behavioral health trials, which often have missing data and observe individual behaviors over varying time periods. In this work, we develop a new decentralized federated multiple imputation-based fuzzy clustering algorithm for complex longitudinal behavioral trial data collected from multisite randomized controlled trials over different time periods. Federated learning (FL) preserves privacy by aggregating model parameters instead of data. Unlike previous FL methods, this proposed algorithm requires only two rounds of communication and handles clients with varying numbers of time points for incomplete longitudinal data. The model is evaluated on both empirical longitudinal dietary health data and simulated clusters with different numbers of clients, effect sizes, correlations, and sample sizes. The proposed algorithm converges rapidly and achieves desirable performance on multiple clustering metrics. This new method allows for targeted treatments for various patient groups while preserving their data privacy and enables the potential for broader applications in the Internet of Medical Things.
更多查看译文
关键词
Federated learning,fuzzy clustering,behavior,diet,decentralized computing,longitudinal trial,missing data,Internet of Medical Things
AI 理解论文
溯源树
样例
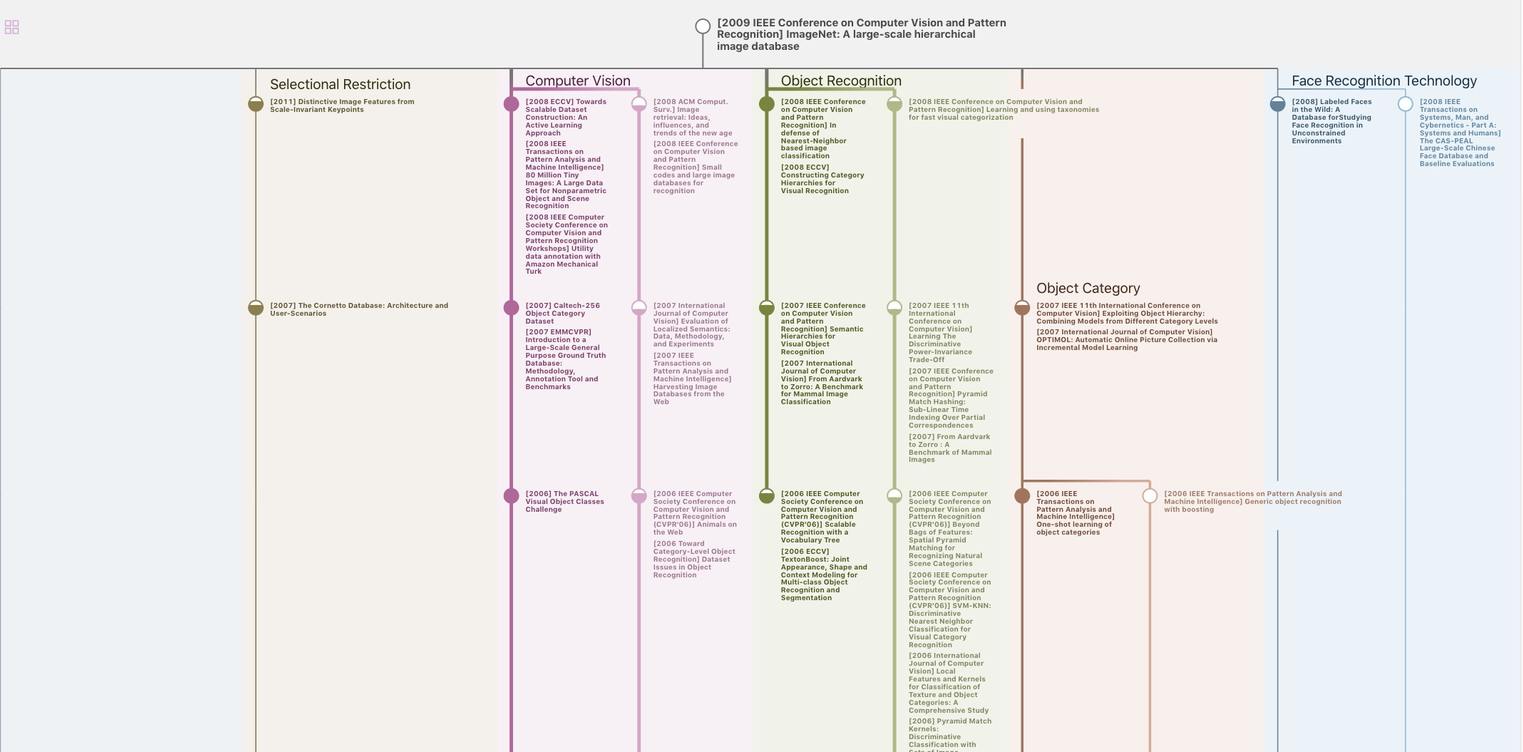
生成溯源树,研究论文发展脉络
Chat Paper
正在生成论文摘要