Asynchronous Federated Learning for Resource Allocation in Software-Defined Internet of UAVs.
IEEE Internet Things J.(2024)
摘要
The use of Unmanned Aerial Vehicles (UAVs) as flying base stations to support various tasks, such as data collection, machine learning (ML) model training, and wireless communication in Internet of Things (IoT) networks, has garnered significant attention in recent years. Nonetheless, several challenges have arisen in this context, including data privacy concerns and limited onboard computational and communication resources. These challenges make the direct transmission of raw data to a central server for training impractical. Moreover, UAV-based networks are susceptible to fluctuating channel conditions and the heterogeneous computing capabilities of IoT devices. Therefore, enhancing the reliability and efficiency of such networks is imperative. In this paper, we introduce a novel framework known as the Asynchronous Federated Framework for IoT-enabled UAV (AF3N) networks. AF3N enables local model training with subsequent parameter transmission to the Mobile Edge Computing (MEC) server. To further enhance learning efficiency, we incorporate a device selection strategy into the AF3N framework. Additionally, we employ a multi-agent Asynchronous Advantage Actor-Critic (A3C)-based joint resource allocation algorithm aimed at reducing latency and energy utilization within the Internet of UAVs (IoUAV) network. Through extensive simulations we comprehensively examine the efficacy and performance of our proposed framework.
更多查看译文
关键词
Internet of Things (IoT),Unmanned Aerial Vehicles (UAVs),Communication Optimization,Transmission Power Optimization
AI 理解论文
溯源树
样例
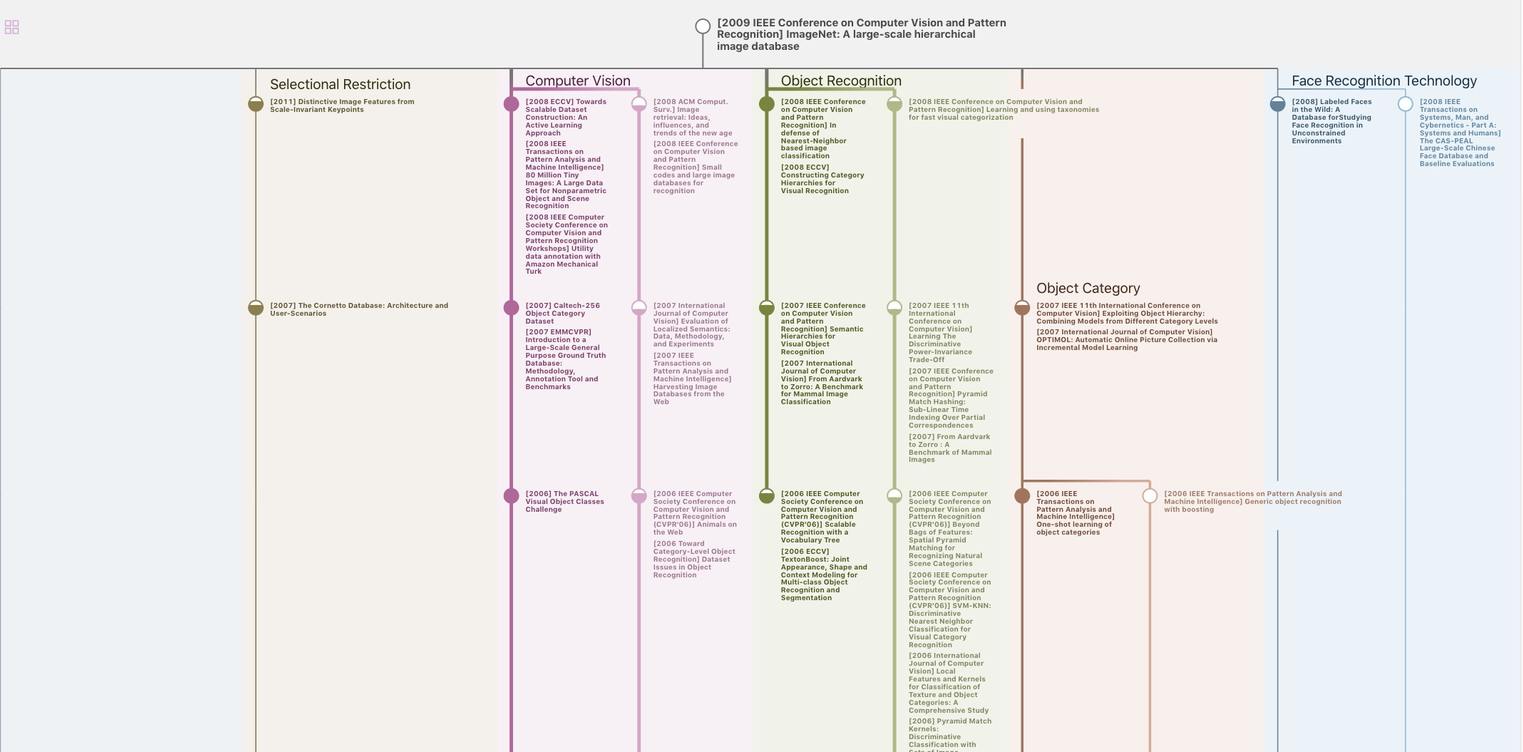
生成溯源树,研究论文发展脉络
Chat Paper
正在生成论文摘要