Acoustic Scene Classification Across Cities and Devices via Feature Disentanglement
IEEE-ACM TRANSACTIONS ON AUDIO SPEECH AND LANGUAGE PROCESSING(2024)
摘要
Acoustic Scene Classification (ASC) is a task that classifies a scene according to environmental acoustic signals. Audios collected from different cities and devices often exhibit biases in feature distributions, which may negatively impact ASC performance. Taking the city and device of the audio collection as two types of data domain, this paper attempts to disentangle the audio features of each domain to remove the related feature biases. A dual-alignment framework is proposed to generalize the ASC system on new devices or cities, by aligning boundaries across domains and decision boundaries within each domain. During the alignment, the maximum classifier discrepancy and gradient reversed layer are used for the feature disentanglement of scene, city and device, while four candidate domain classifiers are proposed to explore the optimal solution of feature disentanglement. To evaluate the dual-alignment framework, three experiments of biased ASC tasks are designed: 1) cross-city ASC in new cities; 2) cross-device ASC in new devices; 3) cross-city-device ASC in new cities and new devices. Results demonstrate the superiority of the proposed framework, showcasing performance improvements of 0.9%, 19.8%, and 10.7% on classification accuracy, respectively. The effectiveness of the proposed feature disentanglement approach is further evaluated in both biased and unbiased ASC problems, and the results demonstrate that better-disentangled audio features can lead to a more robust ASC system across different devices and cities. This paper advocates for the integration of feature disentanglement in ASC systems to achieve more reliable performance.
更多查看译文
关键词
Urban areas,Task analysis,Feature extraction,Testing,Scene classification,Recording,Adaptation models,Acoustic scene classification,domain adaptation,feature disentanglement
AI 理解论文
溯源树
样例
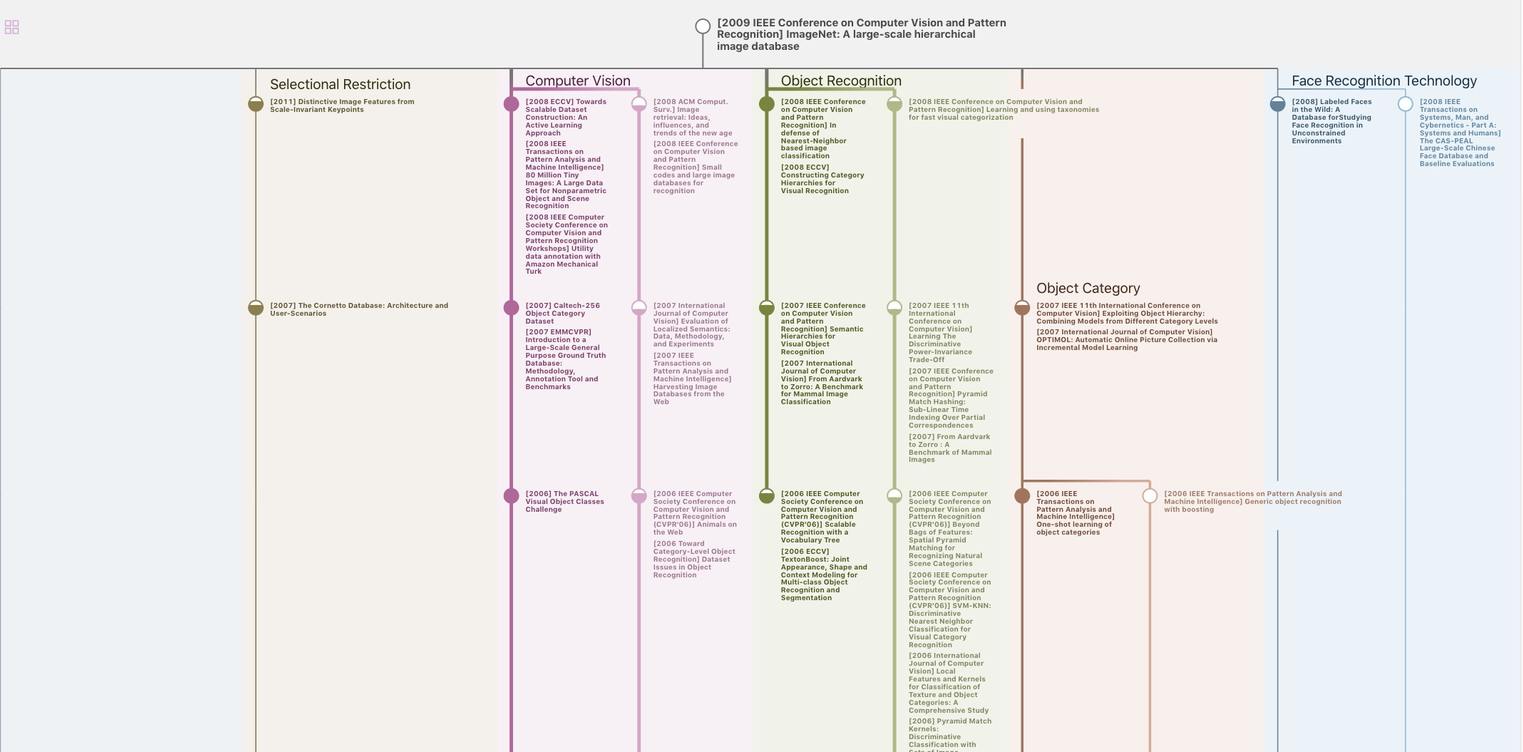
生成溯源树,研究论文发展脉络
Chat Paper
正在生成论文摘要