Counterexample Guided Neural Network Quantization Refinement
IEEE TRANSACTIONS ON COMPUTER-AIDED DESIGN OF INTEGRATED CIRCUITS AND SYSTEMS(2024)
Abstract
Deploying neural networks (NNs) in low-resource domains is challenging because of their high computing, memory, and power requirements. For this reason, NNs are often quantized before deployment, but such an approach degrades their accuracy. Thus, we propose the counterexample-guided neural network quantization refinement (CEG4N) framework, which combines search-based quantization and equivalence checking. The former minimizes computational requirements, while the latter guarantees that the behavior of an NN does not change after quantization. We evaluate CEG4N on a diverse set of benchmarks, including large and small NNs. Our technique successfully quantizes the networks in the chosen evaluation set, while producing models with up to 163% better accuracy than state-of-the-art techniques.
MoreTranslated text
Key words
Equivalent quantization (EQ),neural network equivalence (NNE),neural network (NN) quantization
AI Read Science
Must-Reading Tree
Example
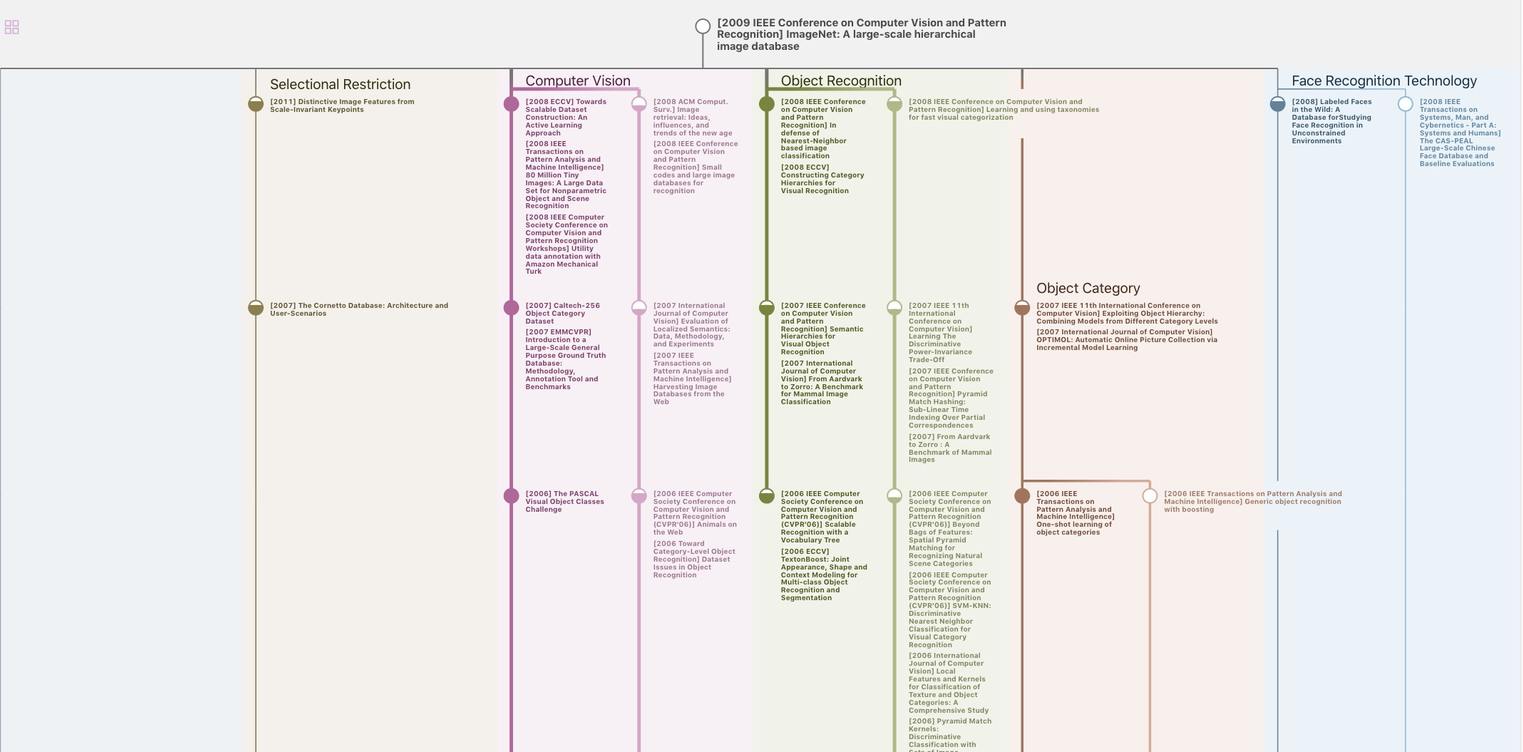
Generate MRT to find the research sequence of this paper
Chat Paper
Summary is being generated by the instructions you defined