Accelerating Deep Reinforcement Learning via Phase-Level Parallelism for Robotics Applications
IEEE COMPUTER ARCHITECTURE LETTERS(2024)
摘要
Deep Reinforcement Learning (DRL) plays a critical role in controlling future intelligent machines like robots and drones. Constantly retrained by newly arriving real-world data, DRL provides optimal autonomous control solutions for adapting to ever-changing environments. However, DRL repeats inference and training that are computationally expensive on resource-constraint mobile/embedded platforms. Even worse, DRL produces a severe hardware underutilization problem due to its unique execution pattern. To overcome the inefficiency of DRL, we propose Train Early Start, a new execution pattern for building the efficient DRL algorithm. Train Early Start parallelizes the inference and training execution, hiding the serialized performance bottleneck and improving the hardware utilization dramatically. Compared to the state-of-the-art mobile SoC, Train Early Start achieves 1.42x speedup and 1.13x energy efficiency.
更多查看译文
关键词
Graphics processing units,Robots,Training,Legged locomotion,Backpropagation,Reinforcement learning,Hardware,Computer systems organization,neural nets,mobile computing
AI 理解论文
溯源树
样例
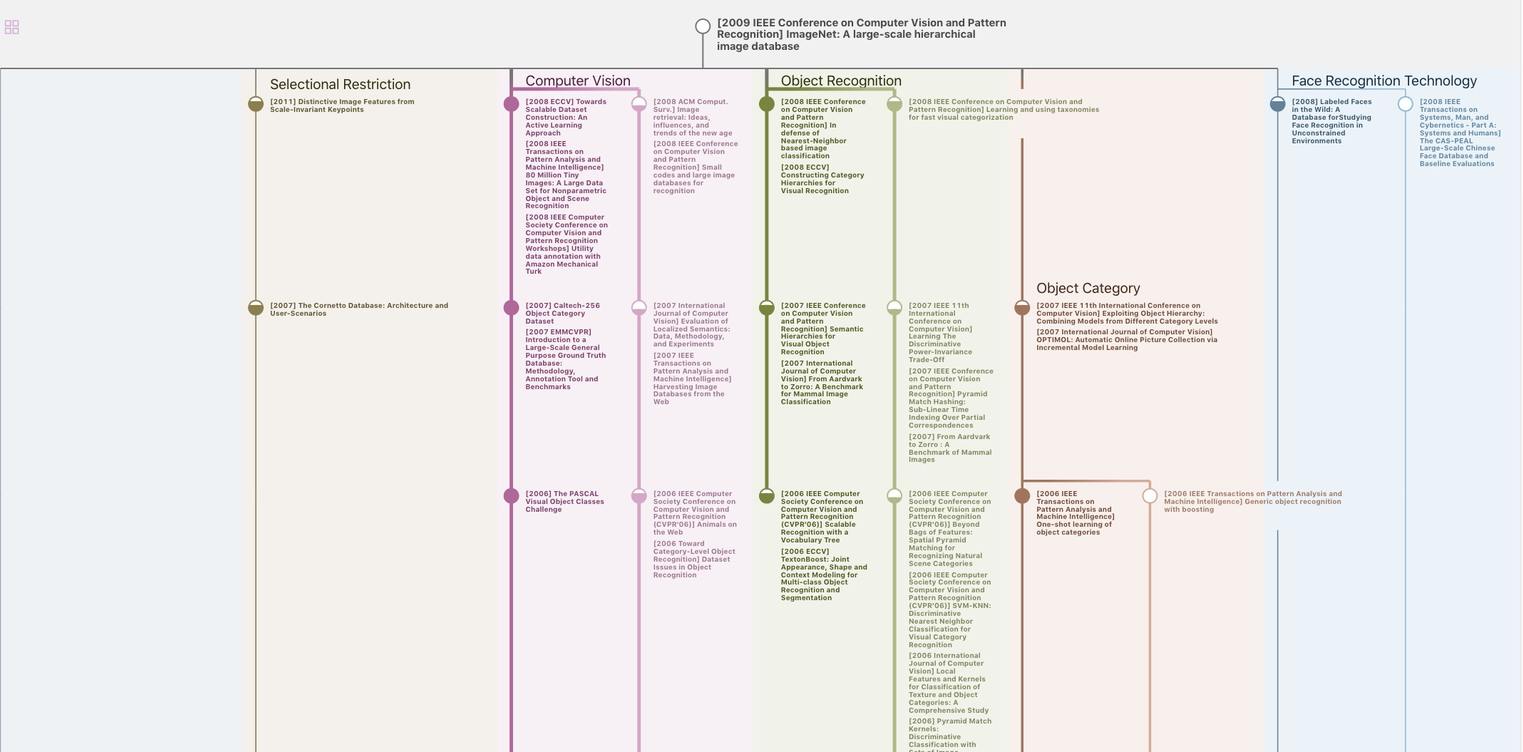
生成溯源树,研究论文发展脉络
Chat Paper
正在生成论文摘要