CiTST-AdderNets: Computing in Toggle Spin Torques MRAM for Energy-Efficient AdderNets
IEEE TRANSACTIONS ON CIRCUITS AND SYSTEMS I-REGULAR PAPERS(2023)
摘要
Recently, Adder Neural Networks (AdderNets) have gained widespread attention as an alternative to traditional Convolutional Neural Networks (CNNs) for deep learning tasks. AdderNets use lightweight addition operations to replace multiplication and accumulation (MAC) operations, but can keep almost the same accuracy compared to other CNNs. Nevertheless, challenges still exist with regards to hardware resources, power consumption, and communication bandwidth, primarily due to the 'Von-Neumann bottlenecks'. However, computing-in-memory (CIM) architecture based on magnetic random-access memory (MRAM) has great potential for edge DNN implementation. In this paper, we propose a novel CIM paradigm using a novel Toggle-Spin-Torques (TST) driven MRAM for energy-efficient AdderNets (called CiTST_AdderNets). In CiTST_AdderNets, MRAM is driven by the interplay of the field-free spin orbit torque (SOT) effect and the spin transfer torque (STT) effect, which offers a fascinating prospect for energy efficiency and speed. Furthermore, a novel CIM paradigm is proposed to implement the dominating subtraction and sum operations in AdderNets, reducing data transfer and the related energy. Meanwhile, a highly parallel array structure integrating computation and storage is designed to support CiTST_AdderNets. In addition, a mapping strategy is proposed to efficiently map the convolution layer on the array. Fully connected layers can also be efficiently computed. The CiTST-AdderNets macro is designed by using a 65-nm CMOS process. Results show that our CiTST-AdderNets consumes about 1.65 mJ, 9.29 mJ, and 42.46 mJ for running VGG8, ResNet-50, and ResNet-18 respectively at 8-bit fixed-point precision. Compared to state-of-the-art platforms, our macro achieves an energy efficiency improvement of 1.45 x to 66.78 x.
更多查看译文
关键词
Computing-in-memory,toggle-spin-torques,magnetic random-access memory,adder neural networks
AI 理解论文
溯源树
样例
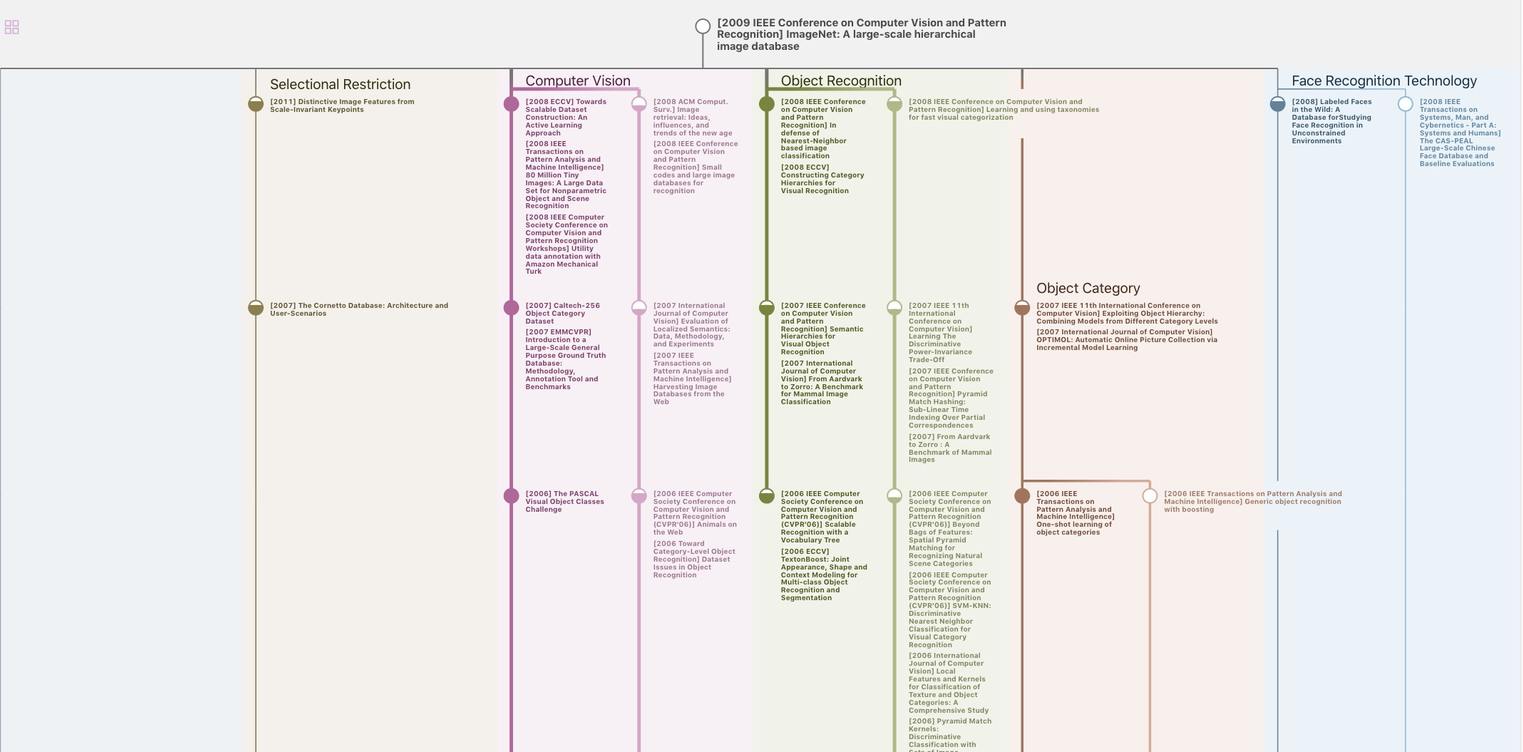
生成溯源树,研究论文发展脉络
Chat Paper
正在生成论文摘要