Implicit Mutual Learning With Dual-Branch Networks for Face Super-Resolution
IEEE Transactions on Biometrics, Behavior, and Identity Science(2024)
摘要
Face super-resolution (SR) algorithms have recently made significant progress. However, most existing methods prefer to employ texture and structure information together to promote the generation of high-resolution features, neglecting the mutual encouragement between them, as well as the effective unification of their own low-level and high-level information, thus yielding unsatisfactory results. To address these problems, we propose an implicit mutual learning of dual-branch networks for face super-resolution, which adequately considers both extraction and aggregation of structure and texture information. The proposed approach consists of four essential blocks. First, the deep feature extractor is equipped with a deep feature reinforcement module (DFRM) based on two-stage cross-dimensional attention (TCA), which behaves in the texture enhancement and structure reconstruction branches, respectively. Then, we elaborate two information exchange blocks for two branches, one for the first information exchange block (FIEB) from the texture branch to the structure branch and one for the second information exchange block (SIEB) from the structure branch to the texture branch. These two interaction blocks perform further fusion enhancement of potential features. Finally, a hybrid fusion network (HFNet) based on supervised attention executes adaptive aggregation of the enhanced texture and structure maps. Additionally, we use a joint loss function that modifies the recovery of structure information, diminishes the use of potentially erroneous information, and encourages the generation of realistic face images. Experiments on public datasets show that our method consistently achieves better quantitative and qualitative results than SOTA methods.
更多查看译文
关键词
Face super-resolution,cross-dimensional attention,information exchange,hybrid fusion
AI 理解论文
溯源树
样例
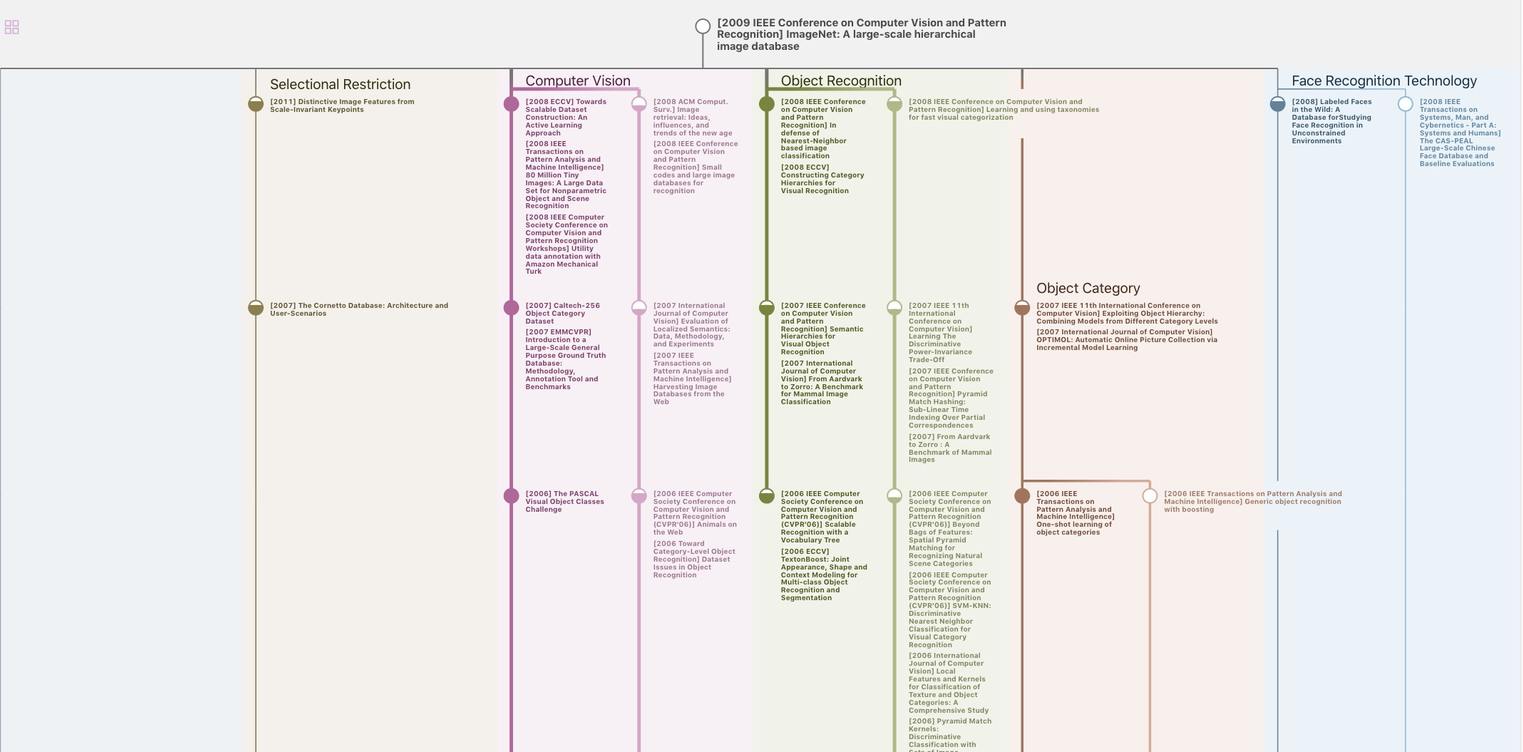
生成溯源树,研究论文发展脉络
Chat Paper
正在生成论文摘要