Improving Graph Collaborative Filtering via Spike Signal Embedding Perturbation
IEEE Transactions on Cognitive and Developmental Systems(2023)
摘要
Nowadays, graph collaborative filtering has proven to be a highly effective method in recommendation systems. It learns user preferences through interactions between users and items. During the training process of graph collaborative filtering, introducing suitable perturbations is crucial to model training. It is commonly used to prevent overfitting and enhance model robustness. Perturbation is widely adopted as a data augmentation technique in recommendation systems and extensively used in contrastive learning. Contrastive learning involves multi-task learning aimed at learning various views from diverse data augmentations. However, these tasks can sometimes interfere with each other, impacting their effectiveness. In contrast to methods that focus on learning various views in contrastive learning to achieve better embedding representations, we propose a straightforward yet highly effective approach to directly incorporate Spike Signal Embedding Perturbation (SEP) into the graph collaborative filtering process.Unlike many other approaches that introduce Gaussian-distributed noise, the spike signals generated by the Poisson encoder typically maintain a positive relationship with the original embeddings. Our experimental results demonstrate that this proposed method significantly enhances the performance of graph collaborative filtering when compared to LightGCN. It leads to substantial improvements in recommendation performance and training efficiency.
更多查看译文
关键词
Recommender systems,collaborative filtering,spike signal,graph convolutional networks
AI 理解论文
溯源树
样例
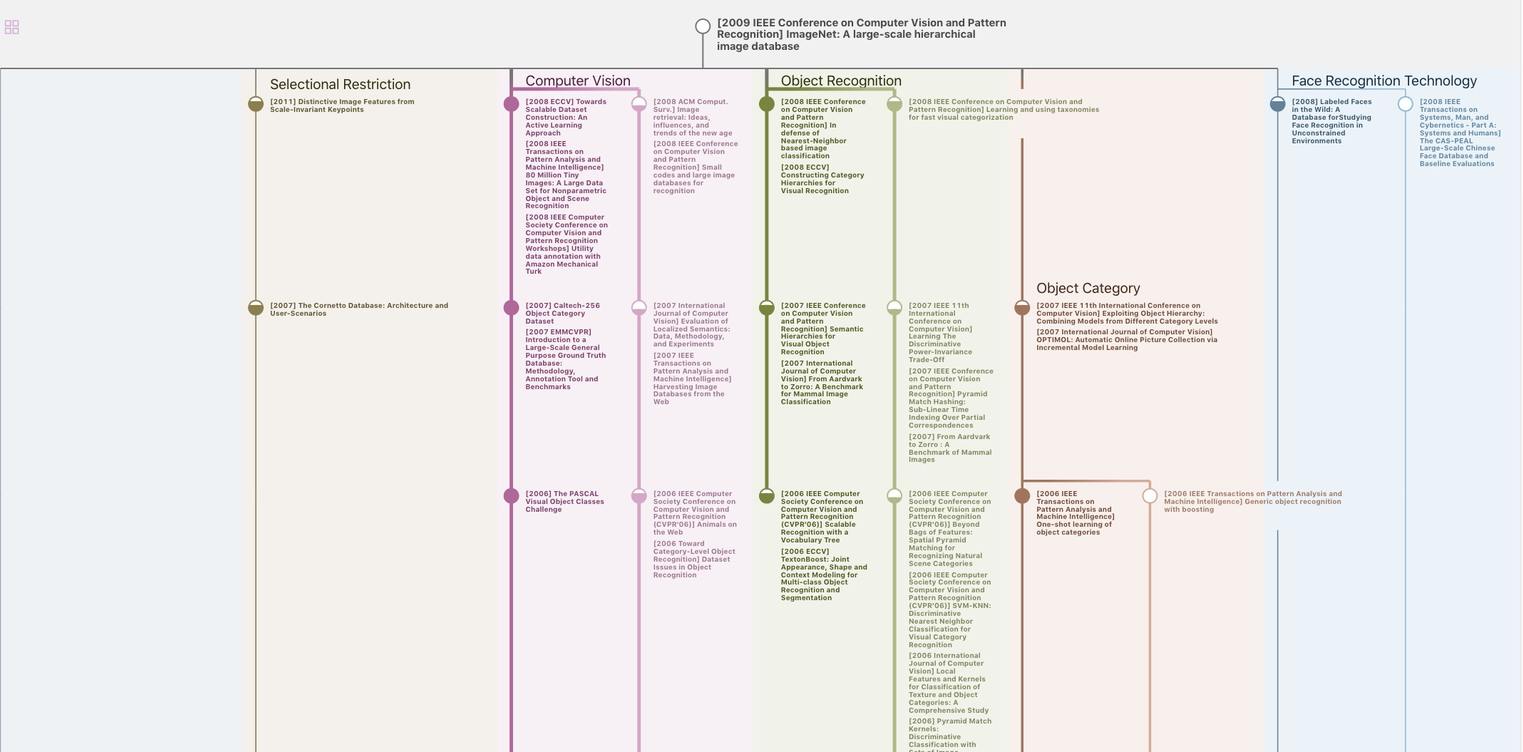
生成溯源树,研究论文发展脉络
Chat Paper
正在生成论文摘要