HASI: Hierarchical Attention-Aware Spatio-Temporal Interaction for Video-Based Person Re-Identification
IEEE Transactions on Circuits and Systems for Video Technology(2023)
摘要
Video-based person re-identification (re-ID) aims to match the same pedestrian of video sequences across non-overlapping cameras. Video re-ID methods generally adopt frame-level feature extraction for different video frames, but they still lack effective spatio-temporal interaction, easily leading to the multi-frame misalignment problem. In this paper, we propose a Hierarchical Attention-aware Spatio-temporal Interaction (HASI) network, including an Attention-aware Temporal Interaction (ATI) module and a Hierarchical Local-spatial Enhancement (HLE) module for video-based person re-ID. In order to avoid the spatial misalignment between video frames, the ATI module employs multiple Frame-to-Frame Temporal Interaction (2FTI) blocks with the Multi-head Inter-frame Alignment Attention (MIAA) to make the current frame iteratively interact with each rest frame of a video in a positive single-cycle manner, rather than only interacting with the adjacent frame or directly building the relationship of all frames at once. This module can not only obtain the long-range non-adjacent temporal information, but also learn the pairwise frame-to-frame relationships. Moreover, the HLE module is designed to enhance the local fine-grained features from multiple Transformer layers, whilst delivering low-level information to further enrich middle-level and high-level semantic knowledge. Thus, our method can learn multi-perspective pedestrian information, including inter-frame long-range interaction information and intra-frame multi-layer global and local information. Extensive experiments demonstrate the superiority of the proposed HASI method compared with the state-of-the-art methods on the three challenging video-based re-ID datasets, i.e., MARS, iLIDS-VID, and PRID-2011.
更多查看译文
关键词
Video-based person re-identification,vision transformer,spatio-temporal interaction,deep feature fusion
AI 理解论文
溯源树
样例
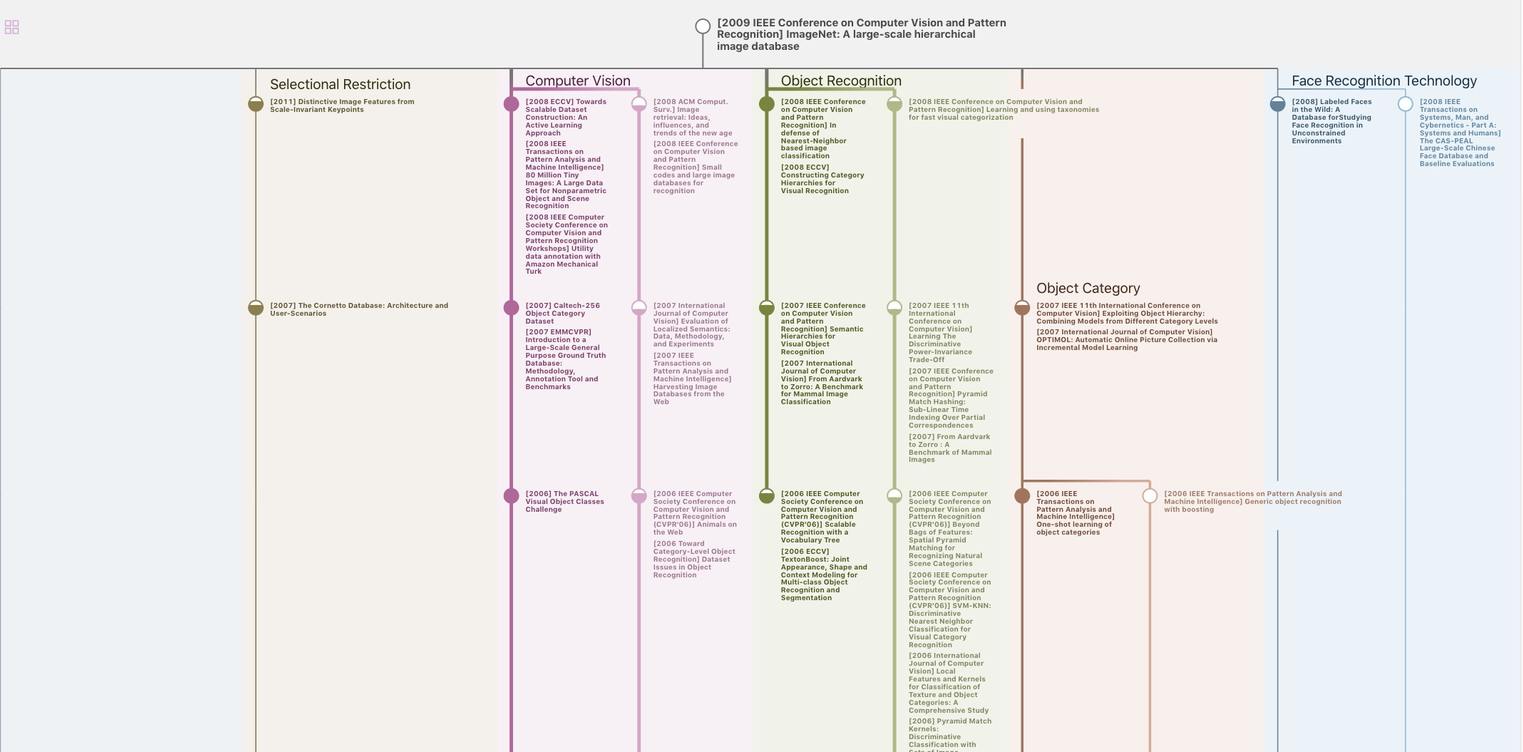
生成溯源树,研究论文发展脉络
Chat Paper
正在生成论文摘要