Small Sample Image Segmentation By Coupling Convolutions and Transformers
IEEE Transactions on Circuits and Systems for Video Technology(2023)
摘要
Compared with natural image segmentation, small sample image segmentation tasks, such as medical image segmentation and defect detection, have been less studied. Recent studies made efforts on bringing together Convolutional Neural Networks (CNNs) and Transformers in a serial or interleaved architecture in order to incorporate long-range dependencies into the features extracted using CNNs. In this study, we argue that these architectures limit the capability of the combination of CNNs and Transformers. To this end, we propose a dual-stream small sample image segmentation network, namely, the Interactive Coupling of Convolutions and Transformers Based UNet (ICCT-UNet)
1
, motivated by the success achieved using the UNet in the scenario of small sample image segmentation. Within this network, a CNN stream is paralleled with a Transformer stream while maintaining feature exchange inside each block through the proposed Window-Based Multi-head Cross-Attention (W-MHCA) mechanism. To derive an overall segmentation, the features learned by both the streams are further fused using a Residual Fusion Module (RFM). Experimental results show that the ICCT-UNet outperforms, or at least performs comparably to, its counterparts on eight sets of medical and defective images. These promising results should be attributed to the effective combination of the local and global features fulfilled by the proposed interactive coupling method.
更多查看译文
关键词
Image Segmentation,Convolutional Neural Networks,Transformers,Cross-Attention
AI 理解论文
溯源树
样例
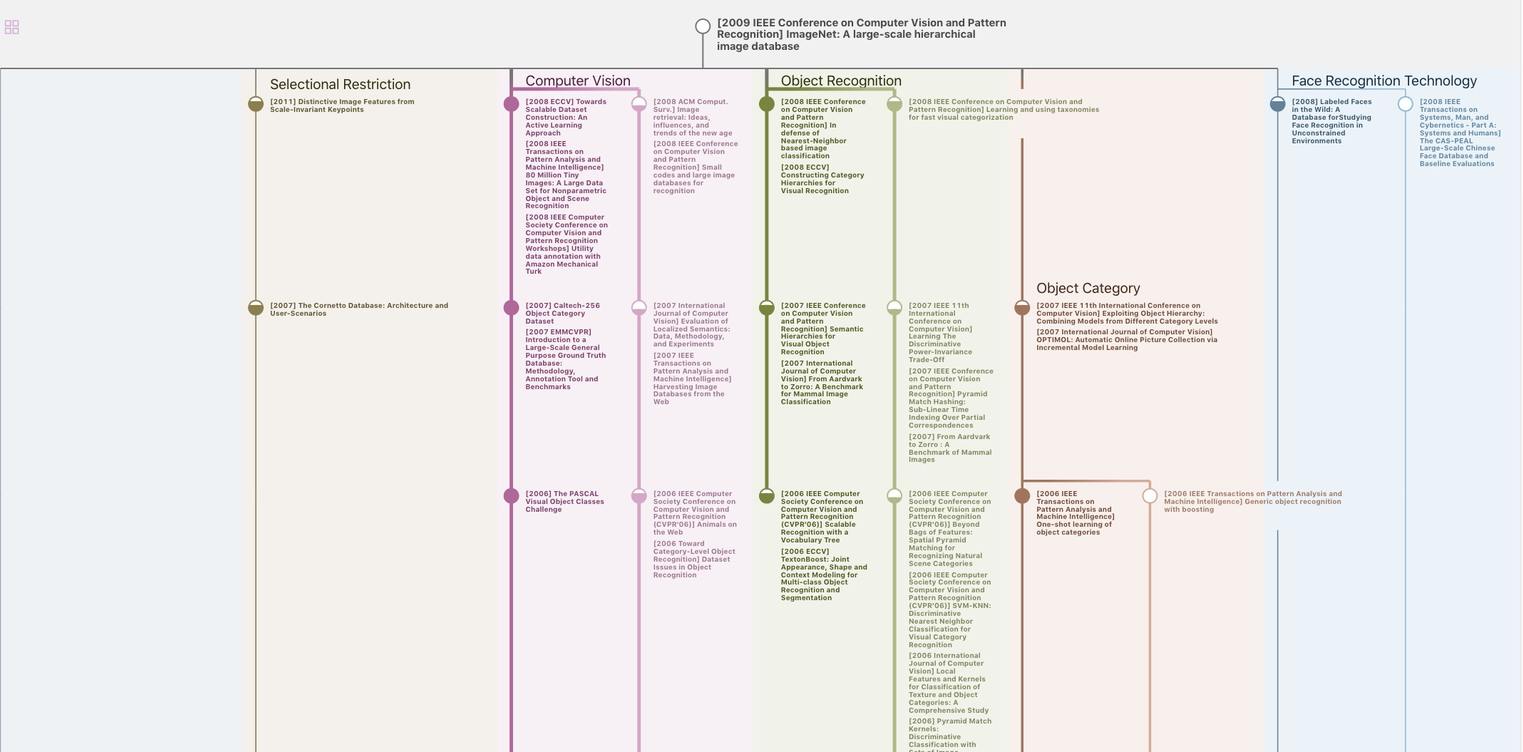
生成溯源树,研究论文发展脉络
Chat Paper
正在生成论文摘要