Bridge Gap in Pixel and Feature Level for Cross-Modality Person Re-Identification.
IEEE Trans. Circuits Syst. Video Technol.(2024)
Abstract
Visible thermal person re-identification (VT-ReID) plays a vital role in intelligent surveillance systems, particularly in weak lighting environments. VT-ReID faces substantial challenges, including the cross-modality gap and intra-class variations. Existing methods address these challenges through either pixel-level image translation techniques or feature-level metric learning techniques. However, the former approaches require additional computational costs and often generate noisy images, making model training challenging. The latter methods focus on constraining the relations between individual instances or class centers, while often ignoring joint consideration of the relationship between the two aspects. In addition, these works do not fully investigate the mutual benefits at both pixel-level and feature-level. To address these limitations, we propose a unified Dual-level Smooth Gap (DSG) learning framework that simultaneously smooths the cross-modality gap at the pixel and feature levels. Specifically, on the one hand, we develop a parameter-free Class-aware Modality Mix (CMM) to smooth the cross-modality gap at the pixel level. CMM can capture and explore internal information between the two modalities by mixing images from different modalities belonging to the same class. On the other hand, we devise an efficient Center-guided Metric Learning (CML) to reduce the inter-modality discrepancy and intra-class variations at the feature level. CML enhances model discrimination and generalization by enforcing constraints on both class centers and instances. Experiments on two benchmark datasets demonstrate the mutual benefits of our proposed and show the superior performance of our method over state-of-the-art methods.
MoreTranslated text
Key words
Person Re-Identification,Cross-Modality,Metric Learning,Modality Alignment
AI Read Science
Must-Reading Tree
Example
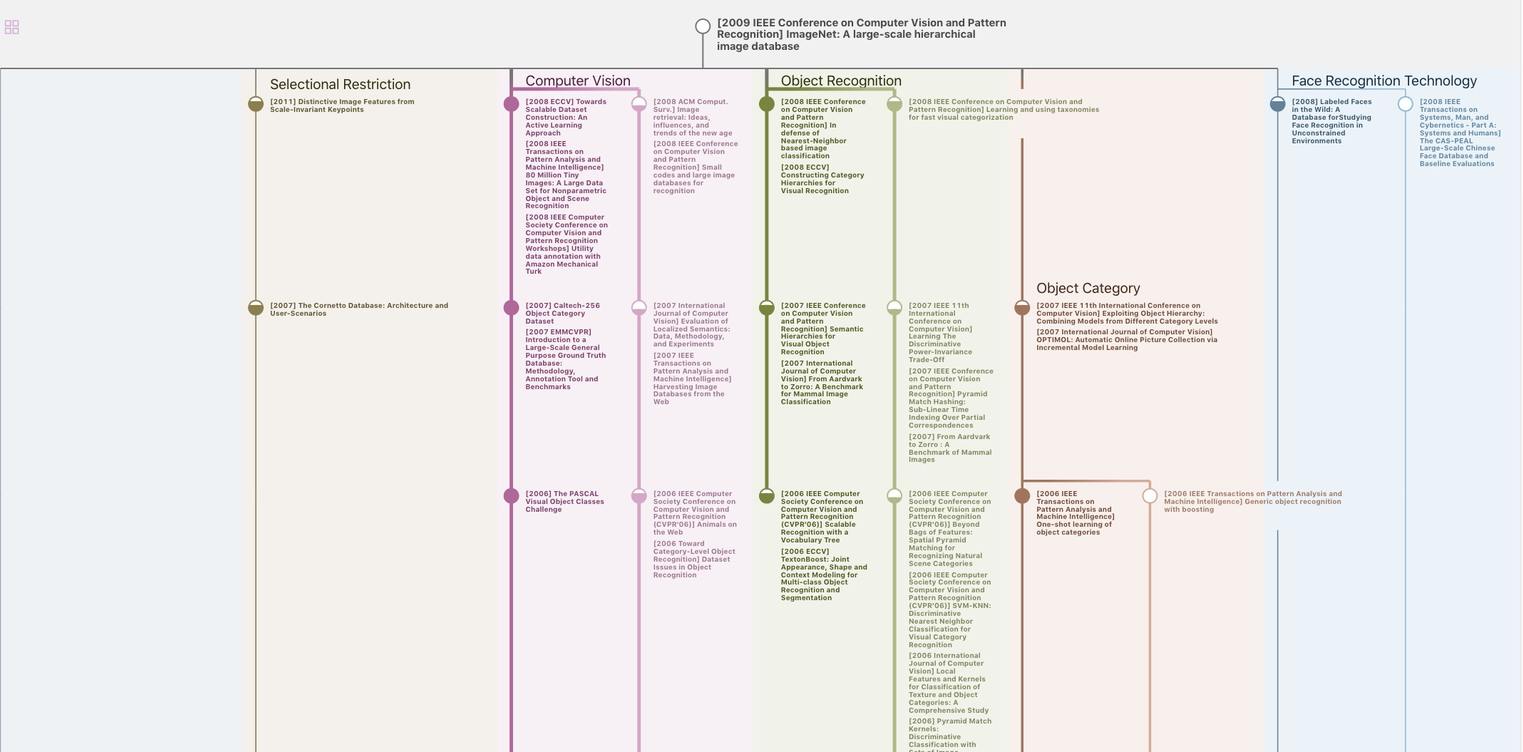
Generate MRT to find the research sequence of this paper
Chat Paper
Summary is being generated by the instructions you defined