PLURAL: 3D Point Cloud Transfer Learning via Contrastive Learning With Augmentations
IEEE TRANSACTIONS ON AUTOMATION SCIENCE AND ENGINEERING(2023)
摘要
Unlocking the power of 3D point cloud machine learning models can be a challenge due to the need for extensive labeled datasets, which presents a challenge when applying these models to new domains. Transfer learning can help overcome this challenge by utilizing data from related tasks to enhance model performance. However, traditional (2D) transfer learning methods struggle with 3D point cloud domain adaptation, due to differences in physical environments and sensor configurations. To address this issue, we propose PLURAL, a novel 3D point cloud transfer learning methodology based on contrastive learning with augmentations. Our approach is inspired by the notion that high-level shape features are more transferable than low-level geometry features. We propose a co-training architecture that includes separate 3D point cloud models with domain-specific parameters, as well as a module for learning domain-invariant features. Additionally, PLURAL extends the approach of contrastive instance alignment to 3D point cloud modeling by considering physics-informed hard sample mining. Our experiments on simulation and real-world datasets demonstrate that PLURAL outperforms state-of-the-art transfer learning methods by a significant margin, effectively reducing the domain gap.
更多查看译文
关键词
Transfer learning,3D point cloud,contrastive learning,hard sample mining
AI 理解论文
溯源树
样例
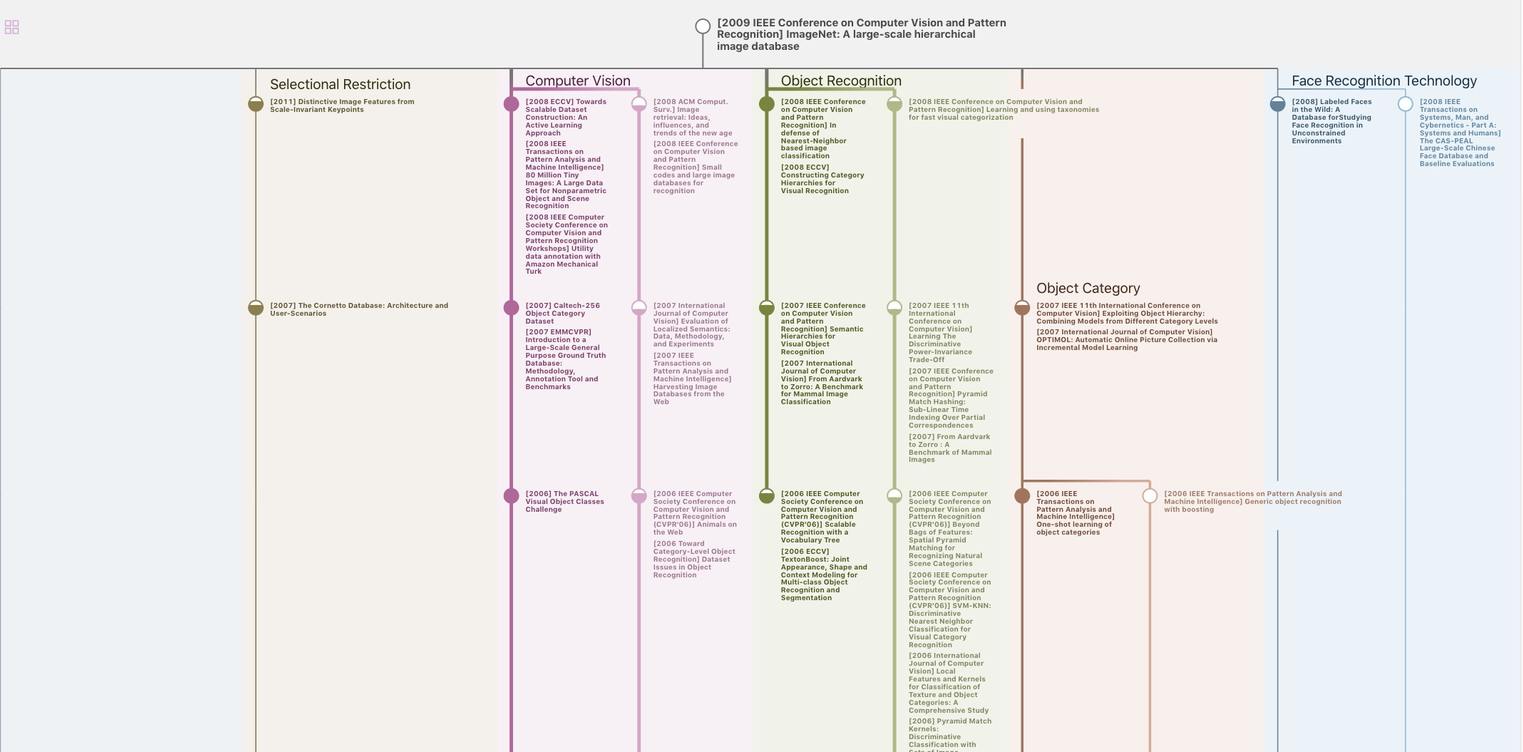
生成溯源树,研究论文发展脉络
Chat Paper
正在生成论文摘要