Underwater Target Detection Using Deep Learning: Methodologies, Challenges, Applications, and Future Evolution
IEEE ACCESS(2024)
摘要
This paper provides a study of the latest target (object) detection algorithms for underwater wireless sensor networks (UWSNs). To ensure selection of the latest and state-of-the-art algorithms, only algorithms developed in the last seven years are taken into account that are not entirely addressed by the existing surveys. These algorithms are classified based on their architecture and methodologies of operation and their applications are described that are helpful in their selection in a diverse set of applications. The merits and demerits of the algorithms are also addressed that are helpful to improve their performance in future investigation. Moreover, a comparative analysis of the described algorithms is also given that further provides an insight to their selection in various applications and future enhancement. A depiction of the addressed algorithms in various applications based on publication count over the latest decade (2023-2013) is also given using the IEEE database that is helpful in knowing their future application trend. Finally, the challenges associated with the underwater target detection are highlighted and the future research paradigms are identified. The conducted study is helpful in providing a thorough analysis of the underwater target detection algorithms, their feasibility in various applications with future challenges and defined strategies for further investigation.
更多查看译文
关键词
Classification algorithms,Feature extraction,YOLO,Radar tracking,Deep learning,Computer architecture,Target tracking,Underwater target detection,deep learning,underwater object detection,convolutional neural networks,ConVNNs
AI 理解论文
溯源树
样例
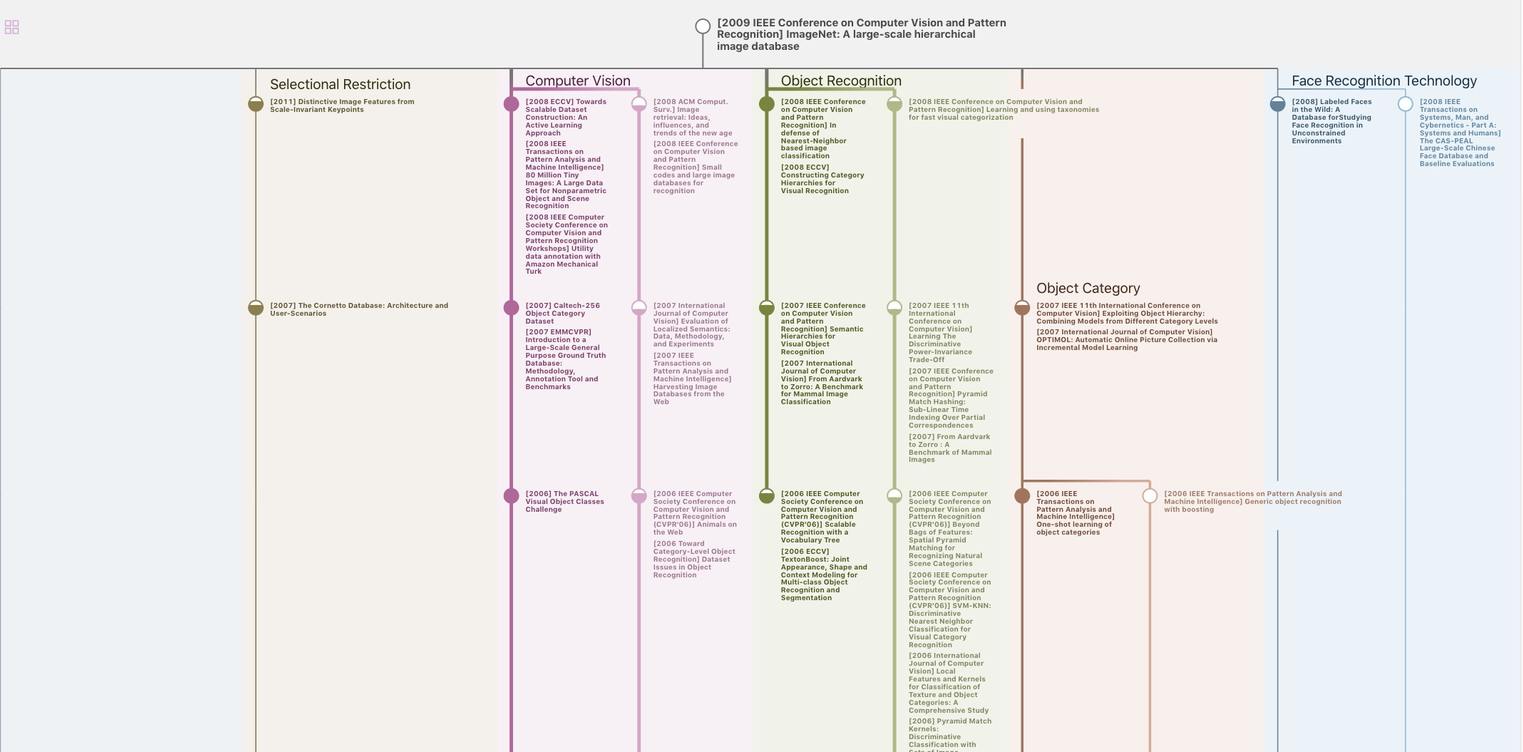
生成溯源树,研究论文发展脉络
Chat Paper
正在生成论文摘要