An Efficient Multi-Edge Server Coalition Computation Offloading Scheme of Sensor-Edge-Cloud
IEEE ACCESS(2024)
摘要
The high latency and high energy consumption of wireless body areas networks (WBANs) for computing-intensive tasks is intolerable, especially for remote interventional surgery. In this paper, a multi-mobile edge server collaborative computation offloading scheme is proposed, which enables tasks to choose a server and offload a certain proportion of computation to efficiently handle computing-intensive services for massive users. More specifically, we formulate the problem of minimizing system latency and energy consumption, and then model the task offloading and resource allocation process as a Markov decision process (MDP). We have developed a scheme called m4m-PDQN to optimize offloading decisions, aiming to minimize the weighted sum of latency and energy consumption. Compared to existing single-server offloading schemes, it is more effective in utilizing computing resources and reducing waiting time and energy consumption for computing tasks in the multiple-server collaborative computing scenarios. The experimental results show that it outperforms other algorithms in terms of performance and efficiency, significantly improving the quality of service (QoS) for wearable wireless body area networks for medical applications.
更多查看译文
关键词
Multi-access edge computing (MEC),reinforcement learning,telemedicine,wireless body area networks
AI 理解论文
溯源树
样例
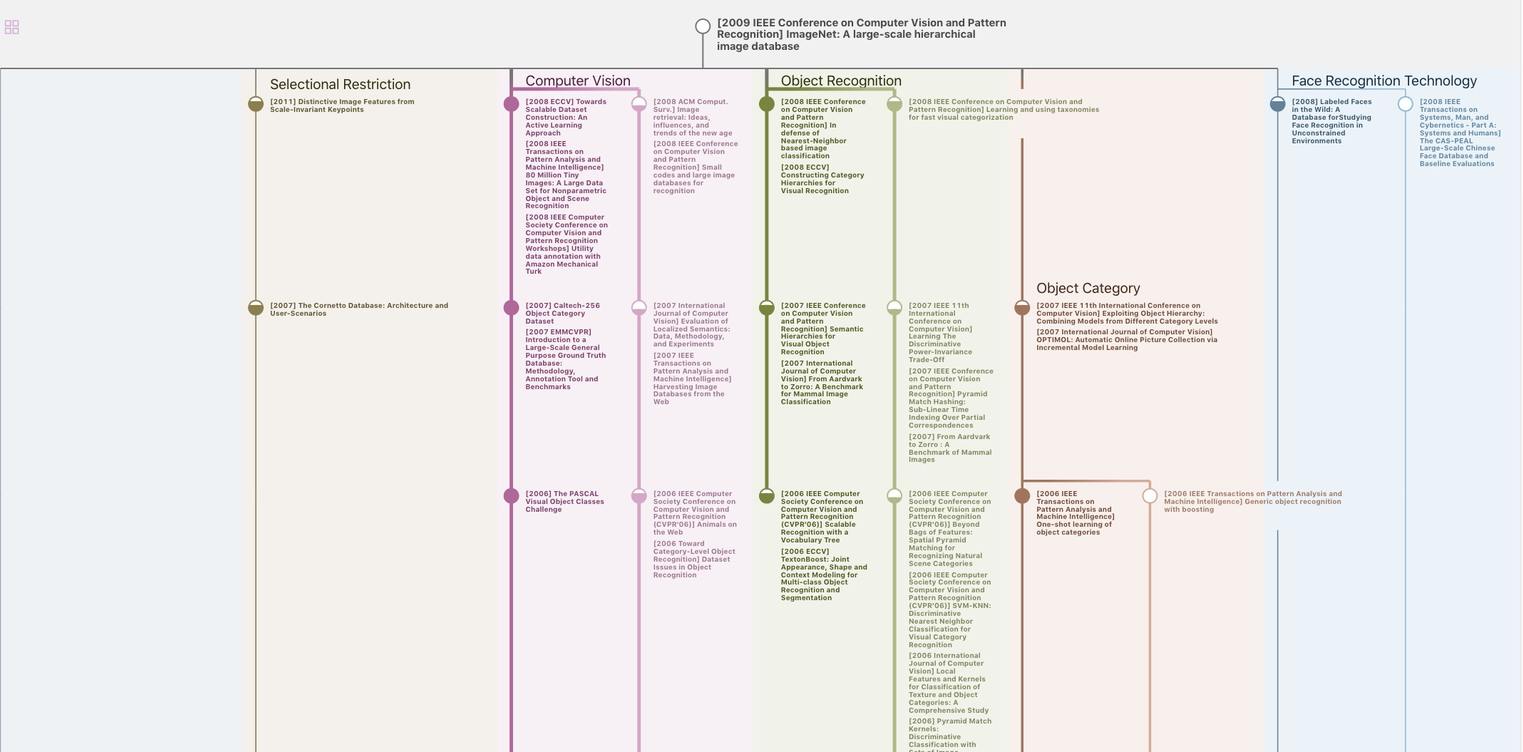
生成溯源树,研究论文发展脉络
Chat Paper
正在生成论文摘要