Multi-Task Learning of the PatchTCN-TST Model for Short-Term Multi-Load Energy Forecasting Considering Indoor Environments in a Smart Building
IEEE ACCESS(2024)
摘要
Energy consumption in buildings contributes to over a third of global energy consumption and 28% of greenhouse gas emissions. With urbanization and population growth, rising building energy demand can lead to environmental degradation. While significant renewable resources are used to generate electricity to mitigate environmental problems, demand-side management remains crucial for achieving net-zero emissions and enhancing energy efficiency. Accurate building load forecasting is pivotal in devising optimal demand response schemes to shift or reduce the demand on power grids. Recent studies have achieved progressive breakthroughs in building energy forecasting through machine learning algorithms. However, most studies focused on building-level energy forecasting rather than individual load forecasting, which cannot support controlled demand response programs. In this study, we propose a multi-task learning model incorporating Patch, Temporal Convolutional Network and Time-Series Transformer (PatchTCN-TST) based on the channel-independent strategy for floor-level multiple electricity loads and indoor environmental forecasting. The PatchTCN-TST model is implemented to predict future data ranging from one-step ahead to three-step ahead on a real-world office building in Bangkok, Thailand. The experiment results indicate that the prediction performance of our model outperforms the prevalent methods, including LSTM, GRU, TCN, Transformer, Informer and Autoformer. The PatchTCN-TST model demonstrates superior accuracy in three forecasting scenarios, significantly reducing MAE, MSE, RMSE, and aSMAPE by 34%, 23%, 12%, and 36.4%, respectively, compared to the best baseline model.
更多查看译文
关键词
Deep learning,Smart buildings,demand response,time-series forecasting,smart buildings
AI 理解论文
溯源树
样例
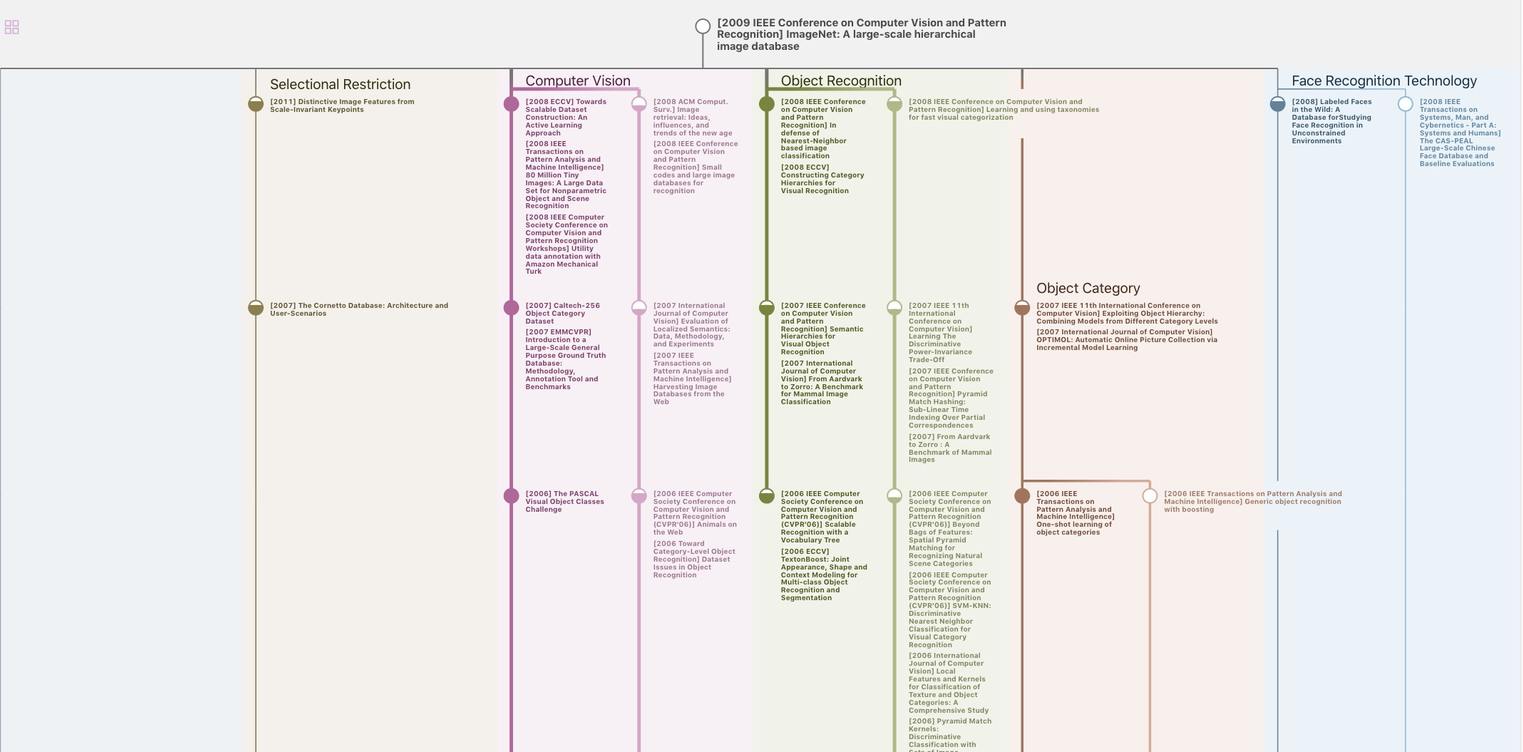
生成溯源树,研究论文发展脉络
Chat Paper
正在生成论文摘要