Statistics Enhancement Generative Adversarial Networks for Diverse Conditional Image Synthesis
IEEE Transactions on Circuits and Systems for Video Technology(2023)
Abstract
Conditional generative adversarial networks (cGANs) aim to synthesize diverse images given the input conditions and the latent codes, but they are prone to map an input to a single output regardless of the variations in latent code, which is also well known as the mode collapse problem of cGANs. To alleviate the problem, in this paper, we investigate explicitly enhancing the statistical dependency between the latent code and the synthesized image in cGANs by utilizing mutual information neural estimators to estimate and maximize the conditional mutual information (CMI) between them given the input condition. The method provides a new perspective from information theory to improve diversity for cGANs and can facilitate many existing conditional image synthesis frameworks with a simple neural estimator extension. Moreover, our studies show that several key designs, including the neural estimator choice, the neural estimator’s network design, and the sampling strategy, are crucial to the success of the method. Extensive experiments on four popular conditional image synthesis tasks, including class-conditioned image generation, paired and unpaired image-to-image translation, and text-to-image generation, demonstrate the effectiveness and superiority of the proposed method.
MoreTranslated text
Key words
Diverse conditional image synthesis,generative adversarial network,mode collapse,mutual information
AI Read Science
Must-Reading Tree
Example
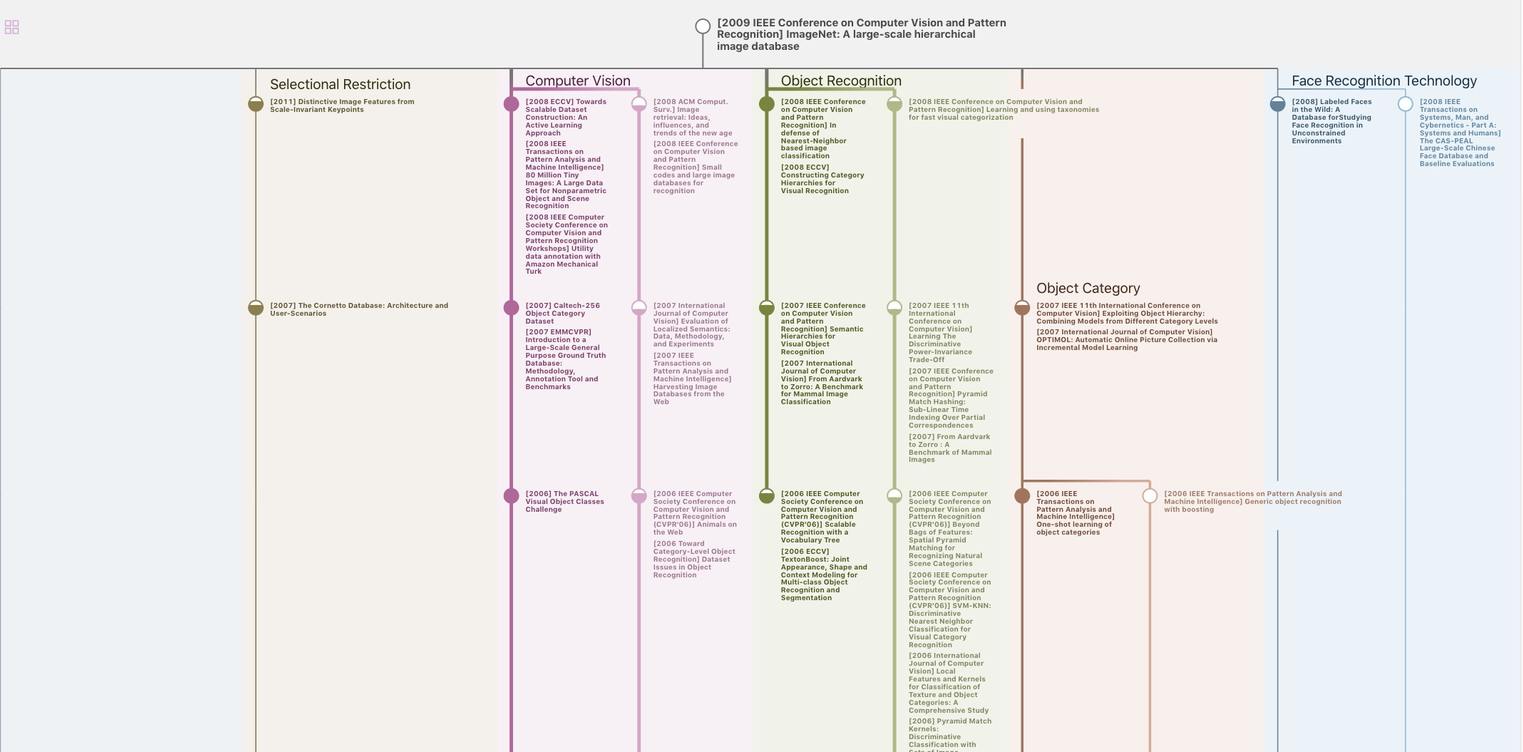
Generate MRT to find the research sequence of this paper
Chat Paper
Summary is being generated by the instructions you defined