Dynamic Nonlinear Indoor Environment Thermal State Estimation With Unknown Inputs Using PSO Guided Regularizer Based Adaptive EKF
IEEE TRANSACTIONS ON AUTOMATION SCIENCE AND ENGINEERING(2024)
Abstract
The present paper shows how the dynamic indoor temperature profile of an HVAC (Heating, Ventilation, and Air Conditioning) system in a building can be developed using Kalman filters, in presence of unknown inputs. An RC network based dynamic, nonlinear thermal model is first developed for the indoor environment with a novel consideration of relative humidity factor. Then an extended Kalman Filter based algorithm in presence of unknown inputs (called EKF-UI) and an adaptive variation of this EKF-UI algorithm (called AdEKF-UI) are developed for the real indoor environment under consideration. Next, a particle swarm optimization (PSO) guided adaptive extended Kalman filter with unknown inputs (PSOgAdEKF-UI) algorithm is proposed to overcome limitations of the EKF-UI and AdEKF-UI algorithms, especially under bad initialization situations. This PSOgAdEKF-UI algorithm proposes an effective utilization of regularizer based initializations for the initial state estimation error covariance matrix and the measurement noise covariance matrix. Extensive experiments showed that, overall, PSOgAdEKF-UI algorithm could outperform EKF-UI and AdEKF-UI algorithms by 46.59% and 20.66%, respectively, in terms of mean square error, while estimating an unknown state.
MoreTranslated text
Key words
HVAC,Heuristic algorithms,Nonlinear dynamical systems,Kalman filters,State estimation,Buildings,Indoor environment,Nonlinear thermal model,RC network,extended Kalman filter,PSO,adaptive EKF,state estimation
AI Read Science
Must-Reading Tree
Example
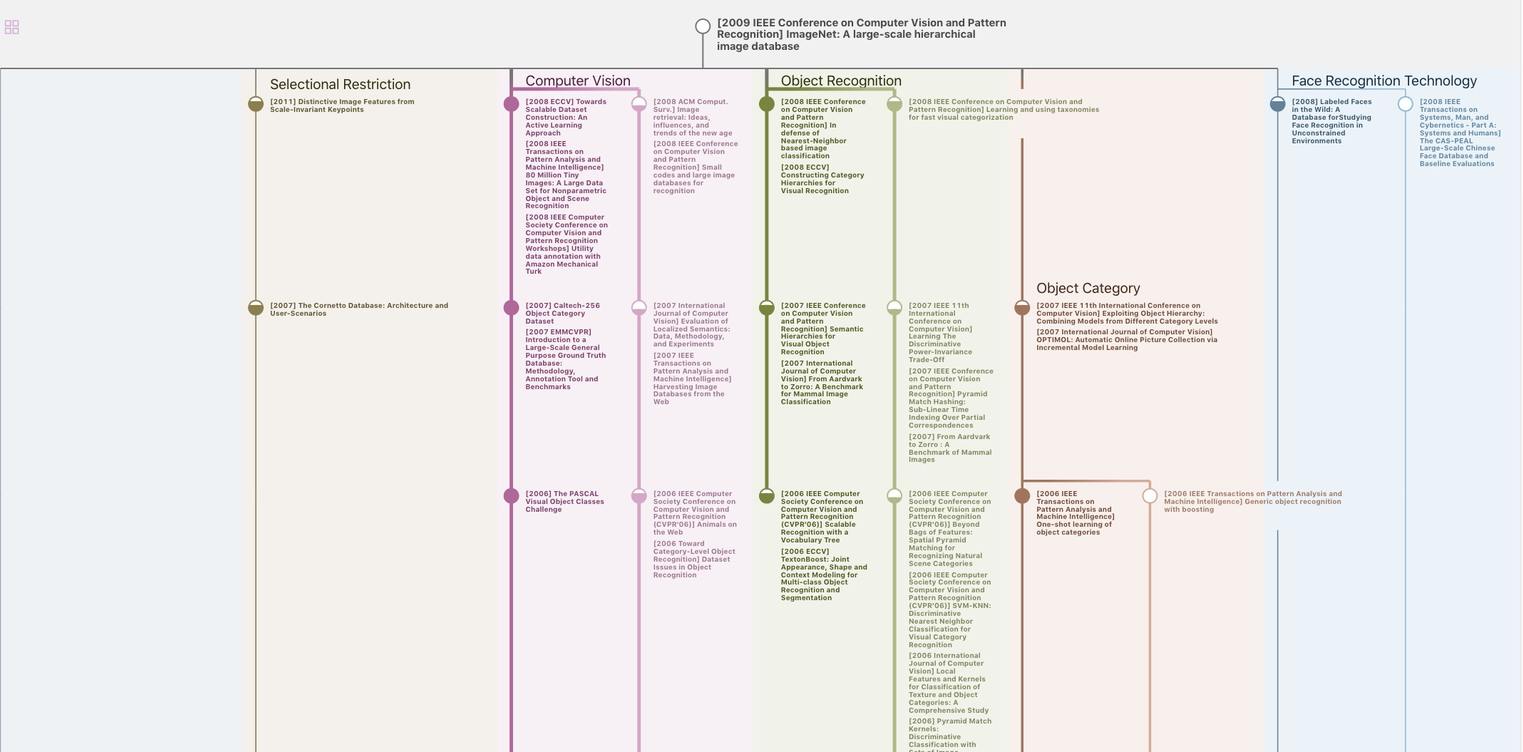
Generate MRT to find the research sequence of this paper
Chat Paper
Summary is being generated by the instructions you defined