SwinIT: Hierarchical Image-to-Image Translation Framework without Cycle Consistency
IEEE Transactions on Circuits and Systems for Video Technology(2024)
摘要
Image-to-image (I2I) translation often requires establishing cycle consistency between the source and the translated images across different domains. However, cycle consistency requires redundant reconstruction, and is too restrictive to satisfy the bijection assumption between the two domains. In this paper, we propose SwinIT, a hierarchical Swin-transformer I2I Translation framework without using cycle consistency. Specifically, we carefully design symmetrical encoders for content and style flows, then explore newly proposed adaptive denormalization and normalization strategies. This framework can effectively capture and fuse content and style representations in a coarse-to-fine manner, ensuring our method achieves high performance without cycle consistency. Guided by element-wise feature adaptive denormalization, our model focuses on preserving semantic structure information. Due to the semantic mismatch between unpaired source and exemplar images, we introduce cross-attention adaptive instance normalization to help achieve better alignment. However, because the original optimization objective lacks direct supervision to preserve high-frequency information, rich edge details are lost during the translation. We propose a wavelet transformation matching loss to recover the details by converting the image into multi-frequency parts. We validate our proposed method in various I2I translation tasks, including arbitrary style transfer, multi-modal image synthesis, and semantic image synthesis, demonstrating its effectiveness in both qualitative and quantitative evaluations.
更多查看译文
关键词
Transformer,Wavelet transformation,Image-to-image translation
AI 理解论文
溯源树
样例
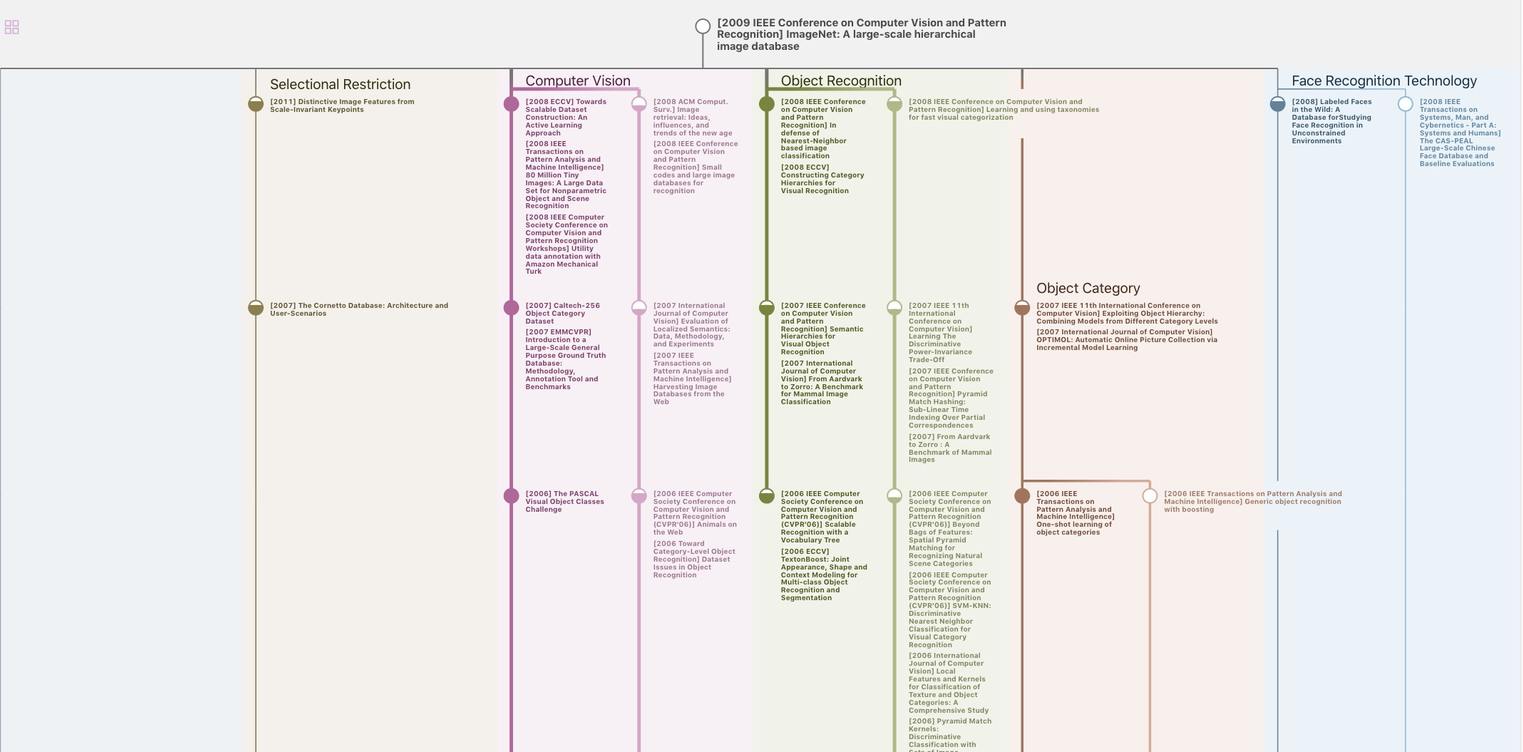
生成溯源树,研究论文发展脉络
Chat Paper
正在生成论文摘要