Resource Knowledge-Driven Heterogeneous Graph Learning for Website Fingerprinting.
IEEE Trans. Cogn. Commun. Netw.(2024)
Abstract
Website fingerprinting (WF) attacks play a crucial role in network traffic analysis for ensuring network security and management. Despite increasing TLS encryption for user privacy, HTTP traffic dominates phishing and pirate website. Fast flux service networks, round robin domain name system, and content delivery networks have rendered IP address or domain name-based WF attacks less effective. Manual feature-based machine learning and recent end-to-end deep learning methods have showed promise. Nevertheless, website content updates induce concept-drift, limiting their accuracy. This study exploits the fact that resource types and website layouts are usually consistent, whereas specific resources are dynamically changing. The resource knowledge extracted from HTTP request packets is utilized to construct a graph representation of website browsing traffic. Then, a heterogeneous graph neural network specifically designed for website fingerprinting using this representation is proposed. This resource knowledge-driven graph learning framework can retain valuable pattern information while mitigating the impact of the concept-drift. The proposed WF attack is evaluated using a real-world dataset comprising over 120,000 malicious and more than 940,000 benign website flows. It can achieve over 98% accuracy when determining benign-malicious websites and 97.6% in identifying website types. These results demonstrate a notable improvement over state-of-the-art WF attacks.
MoreTranslated text
Key words
Network traffic analysis,website fingerprinting,HTTP,resource knowledge,heterogeneous graph neural networks
AI Read Science
Must-Reading Tree
Example
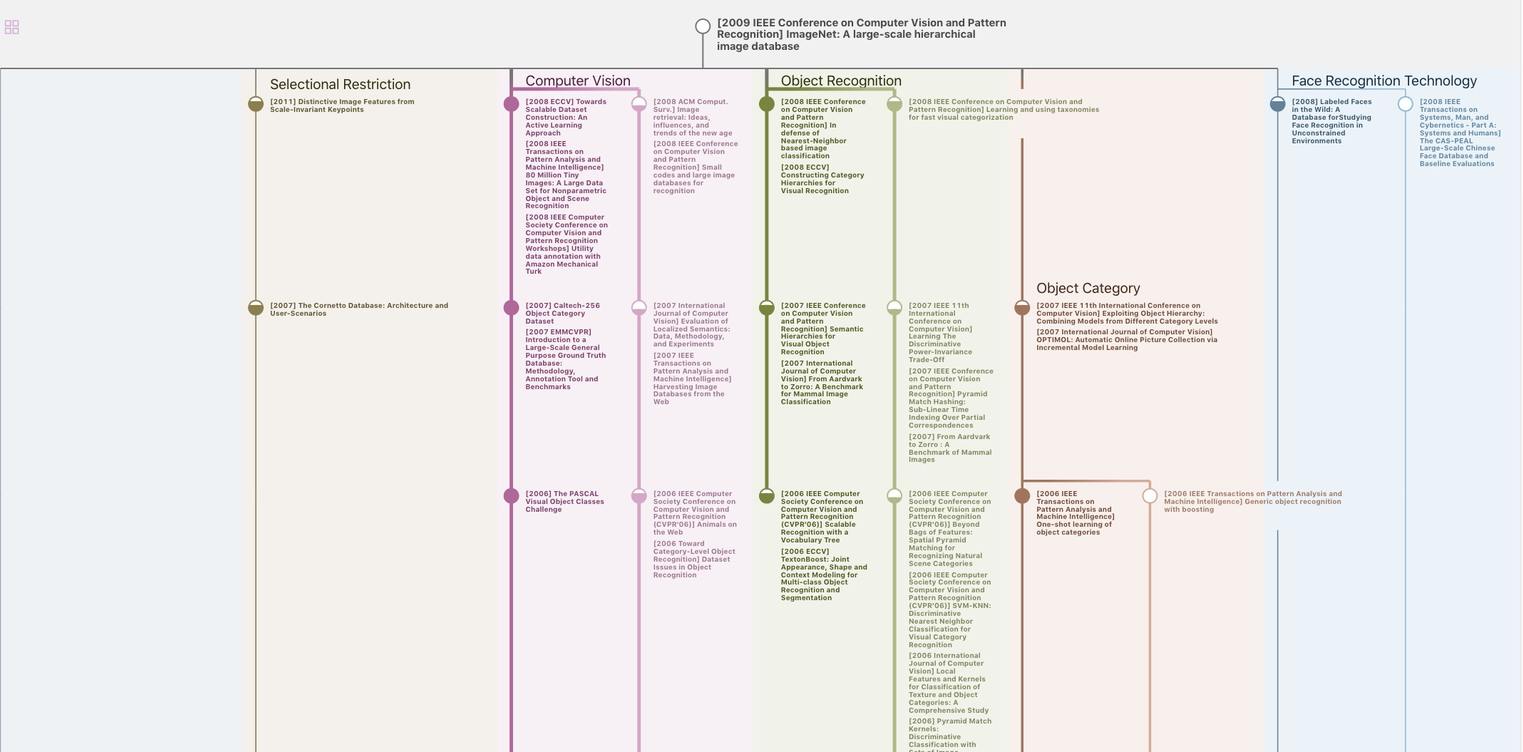
Generate MRT to find the research sequence of this paper
Chat Paper
Summary is being generated by the instructions you defined